Can We Understand Image Semantics from Conventional Neural Networks?
RTIP2R (1)(2018)
Abstract
The Convolutional Neural Networks (CNNs) have been employed successfully for object identification, behavior analysis, letters and digits recognition, etc. The researchers in computer vision committee have studied the capacity of this model in two directions. The first one is improving its performance by increasing layers of the network, using learned features, more data, or more computing (GPUs). The second one is theoretical understanding in architecture design, in optimization and in a generalization of deep networks. One of the first researches in limitation of CNNs in understudying the semantics of images has done by Hosseini et al. in 2017. This result puts the researchers in CNNs community to do more researches to keep up with proper understanding and continued advances in the fields. This paper also forces on analysis the CNNs capability in understanding semantics information in images by recognizing images of the same shape and semantics but opposite the intensity. Experimental results were done on MNIST and GTSRB dataset indicates the limitation of CNNs in understanding the image semantic.
MoreTranslated text
Key words
Drawback of CNN,Semantic image,Convolution
AI Read Science
Must-Reading Tree
Example
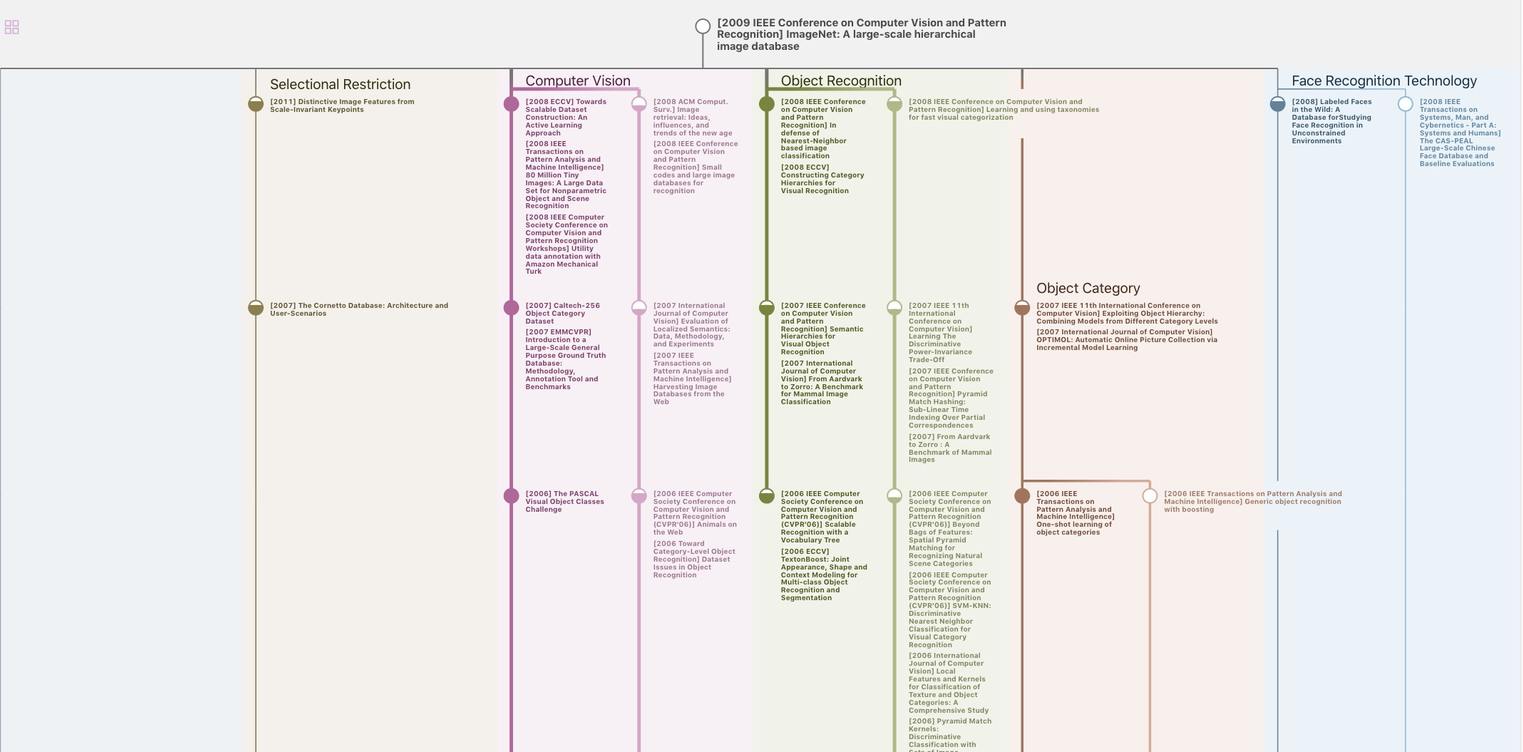
Generate MRT to find the research sequence of this paper
Chat Paper
Summary is being generated by the instructions you defined