A Bypass-Based U-Net For Medical Image Segmentation
INTELLIGENCE SCIENCE AND BIG DATA ENGINEERING: VISUAL DATA ENGINEERING, PT I(2019)
摘要
U-Net has been one of the important deep learning models applied for biomedical image segmentation for a few years. In this paper, inspired by the way how fully convolutional network (FCN) makes dense predictions, we modify U-Net by adding a new bypass for the expansive path. Before combining the contracting path with the upsampled output, we connect with the feature maps from a deeper encoding convolutional layer for the decoding up-convolutional units, and sum up the information learned from both sides. Also, we have implemented this modification to recurrent residual convolutional neural network based on U-Net as well. The experimental results show that the proposed bypass-based U-Net can gain further context information, especially the details from the previous convolutional layer, and outperforms the original U-Net on the DRIVE dataset for retinal vessel segmentation and the ISBI 2018 challenge for skin lesion segmentation.
更多查看译文
关键词
U-Net, Medical image segmentation, Retinal vessel segmentation, Skin lesion segmentation
AI 理解论文
溯源树
样例
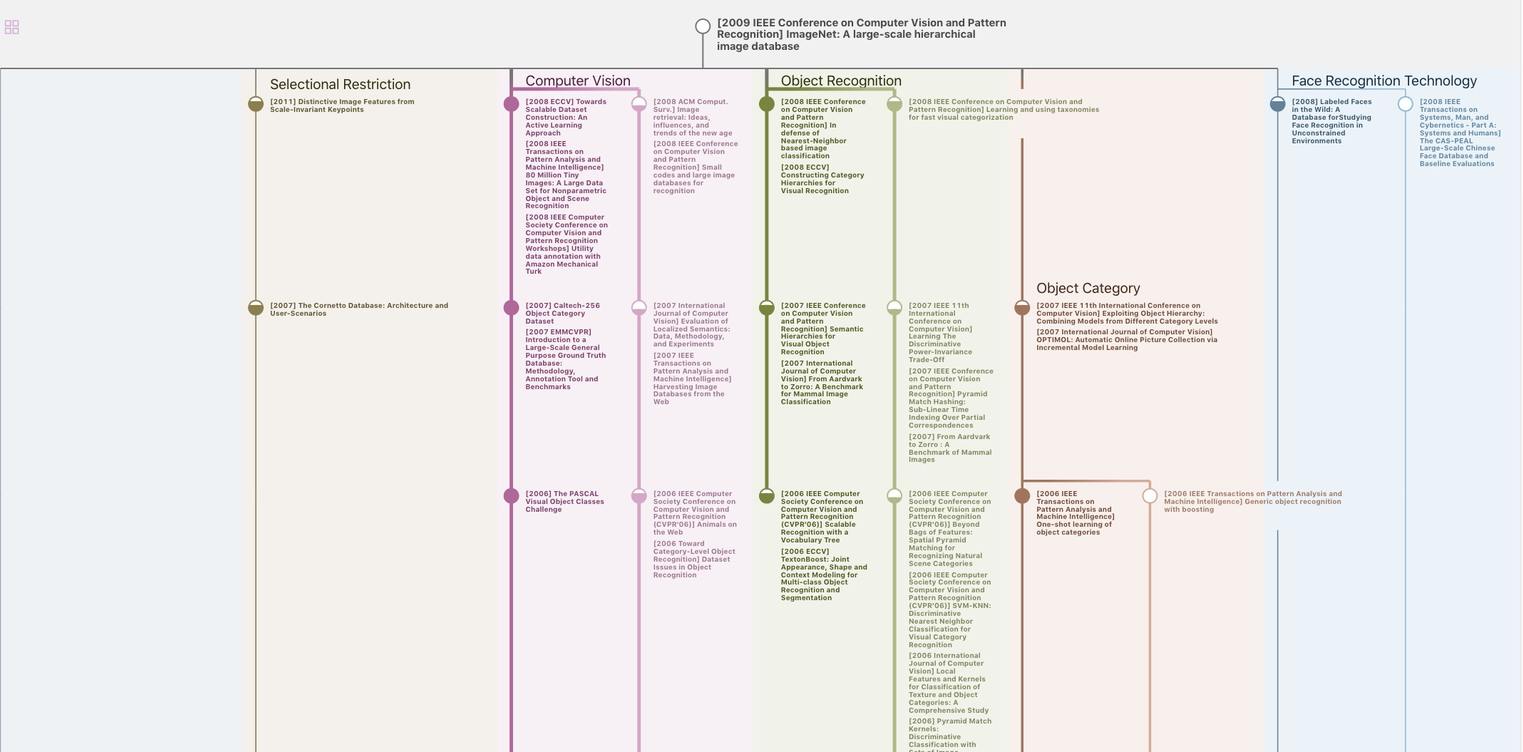
生成溯源树,研究论文发展脉络
Chat Paper
正在生成论文摘要