FeederGAN: Synthetic Feeder Generation via Deep Graph Adversarial Nets
IEEE Transactions on Smart Grid(2021)
摘要
This article presents a novel, automated, generative adversarial networks (GAN) based synthetic feeder generation mechanism, abbreviated as FeederGAN. FeederGAN digests real feeder models represented by directed graphs via a deep learning framework powered by GAN and graph convolutional networks (GCN). Information of a distribution feeder circuit is extracted from its model input files so that the device connectivity is mapped onto the adjacency matrix and the device characteristics, such as circuit types (i.e., 3-phase, 2-phase, and 1-phase) and component attributes (e.g., length and current ratings), are mapped onto the attribute matrix. Then, Wasserstein distance is used to optimize the GAN and GCN is used to discriminate the generated graphs from the actual ones. A greedy method based on graph theory is developed to reconstruct the feeder using the generated adjacency and attribute matrices. Our results show that the GAN generated feeders resemble the actual feeder in both topology and attributes verified by visual inspection and by empirical statistics obtained from actual distribution feeders.
更多查看译文
关键词
Deep learning,distribution system,generative adversarial networks (GAN),graph convolutional networks (GCN),graph theory,synthetic feeder
AI 理解论文
溯源树
样例
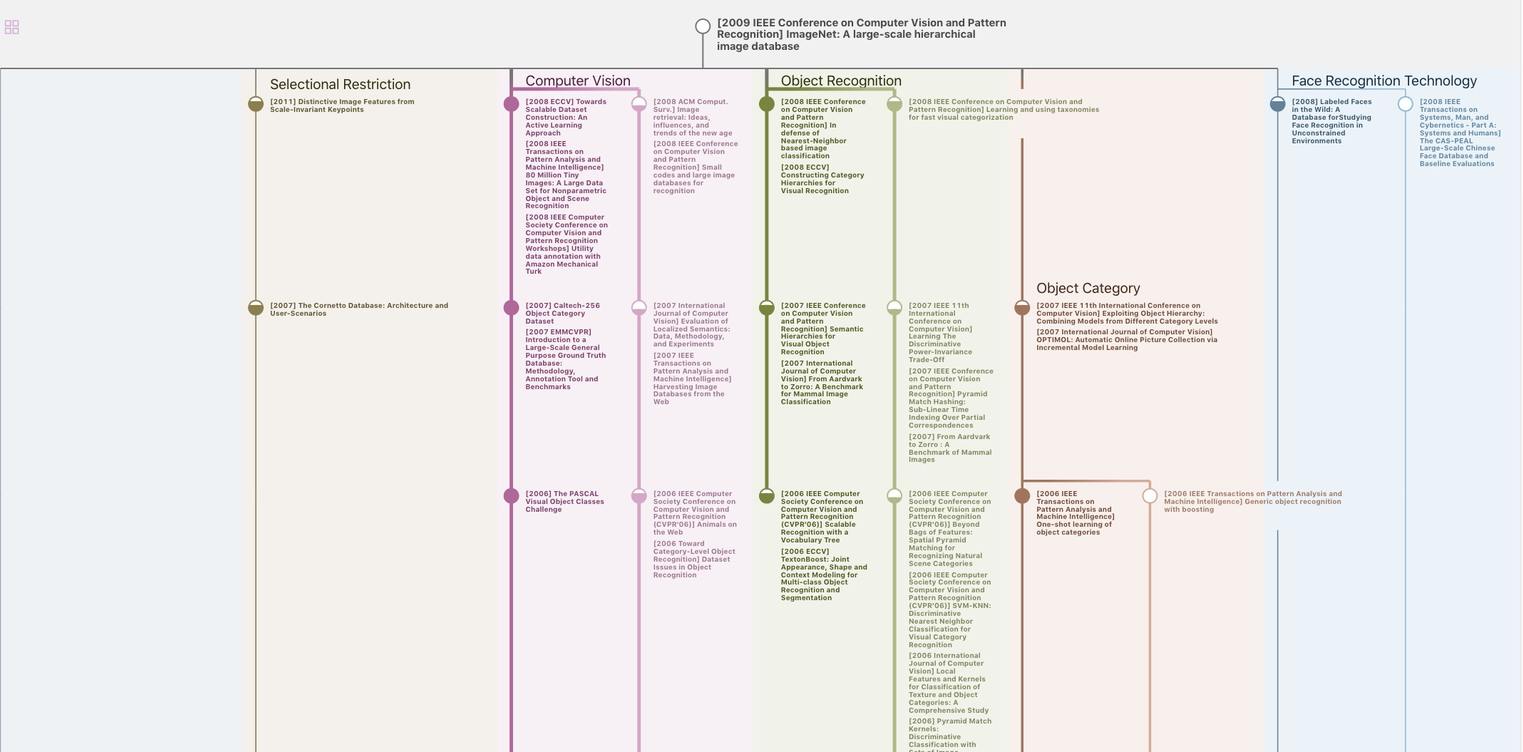
生成溯源树,研究论文发展脉络
Chat Paper
正在生成论文摘要