Unsupervised Weighting of Transfer Rules in Rule-Based Machine Translation using Maximum-Entropy Approach
JOURNAL OF INFORMATION SCIENCE AND ENGINEERING(2020)
摘要
In this paper we present an unsupervised method for learning a model to distinguish between ambiguous selection of structural transfer rules in a rule-based machine translation (MT) system. In rule-based MT systems, transfer rules are the component responsible for converting source language morphological and syntactic structures to target language structures. These transfer rules function by matching a source language pattern of lexical items and applying a sequence of actions. There can, however, be more than one potential sequence of actions for each source language pattern. Our model consists of a set of maximum entropy (or logistic regression) classifiers, one trained for each source language pattern, which select the highest probability sequence of rules for a given sequence of patterns. We perform experiments on the Kazakh - Turkish language pair - a low-resource pair of morphologically-rich languages - and compare our model to two reference MT systems, a rule-based system where transfer rules are applied in a left-to-right longest match manner and to a state-of-the-art system based on the neural encoder-decoder architecture. Our system outforms both of these reference systems in three widely used metrics for machine translation evaluation.
更多查看译文
关键词
machine translation,weighting,structural transfer rules,ambiguous rules,disambiguation
AI 理解论文
溯源树
样例
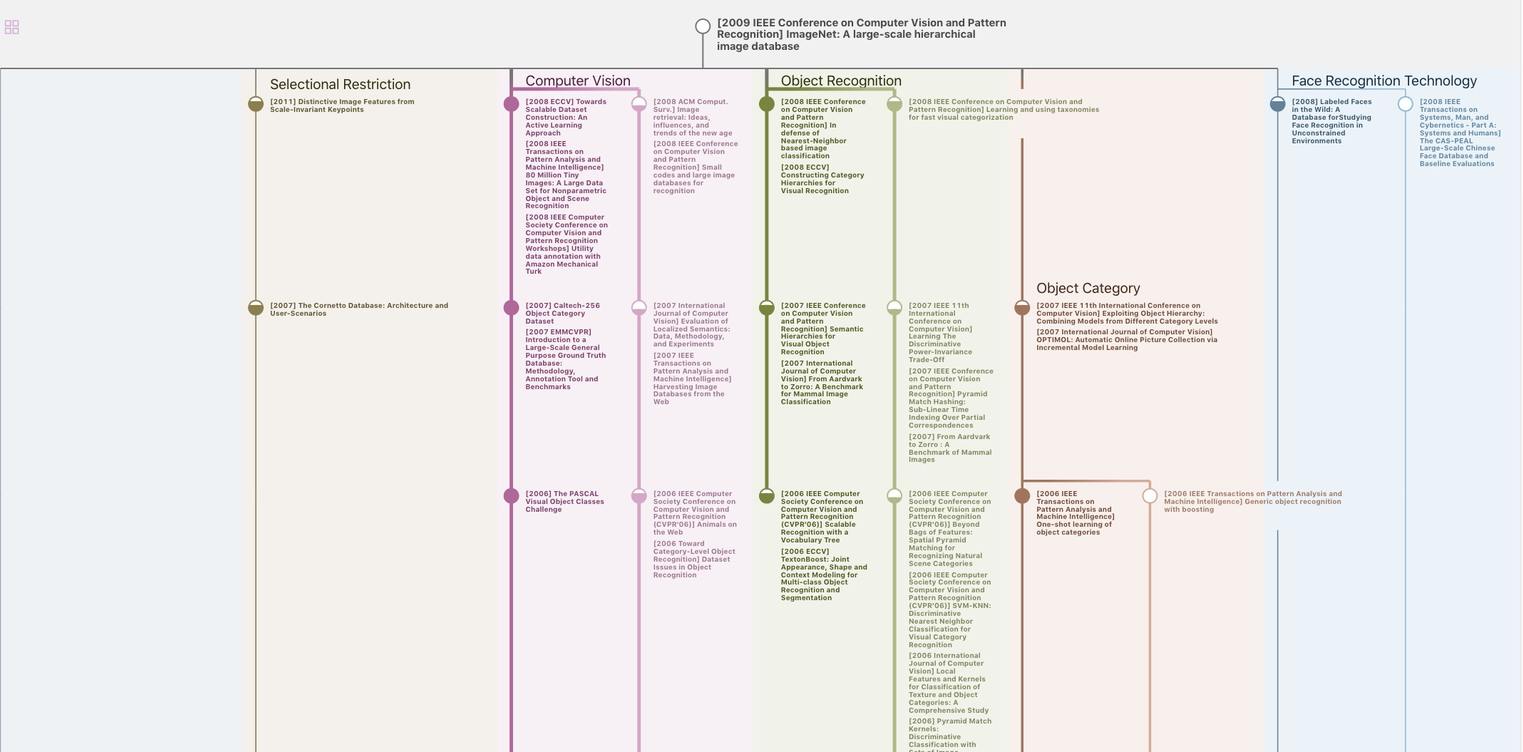
生成溯源树,研究论文发展脉络
Chat Paper
正在生成论文摘要