Prediction of admission in pediatric emergency department with deep neural networks and triage textual data.
Neural Networks(2020)
摘要
Emergency department (ED) overcrowding is a global condition that severely worsens attention to patients, increases clinical risks and affects hospital cost management. A correct and early prediction of ED’s admission is of high value and a motivation to adopt machine learning models. However, several of these studies do not consider data collected in textual form, which is a feature set that contains detailed information about patients and presents great potential for medical health care improvement. To this end, we propose and compare predictive models for admission that use both structured and unstructured data available at triage time. In total, our dataset comprised 499,853 pediatric ED’s presentations (with an admission rate of 5.76%) of patients with age up to 18 years old observed over 3.5 years. Our best model consists of a 2-stage architecture with a deep neural network (DNN) to extract information from textual data followed by a gradient boosting classifier. This combined model achieved a value of 0.892 for the Area Under the Curve (AUC) in the test data. We highlight the importance of DNN-based text processing for better prediction, since the absence of text features resulted in AUC reduction of approximately two percentage points. Also, the feature importance of text was higher than that of the Manchester Triage System (MTS), which is a widely used risk classification protocol. These results suggest that activations from a trained DNN should be used in transfer learning setups in future studies.
更多查看译文
关键词
Deep neural networks,Emergency department admission,Gradient boosting,Prediction model,Triage,Unstructured data
AI 理解论文
溯源树
样例
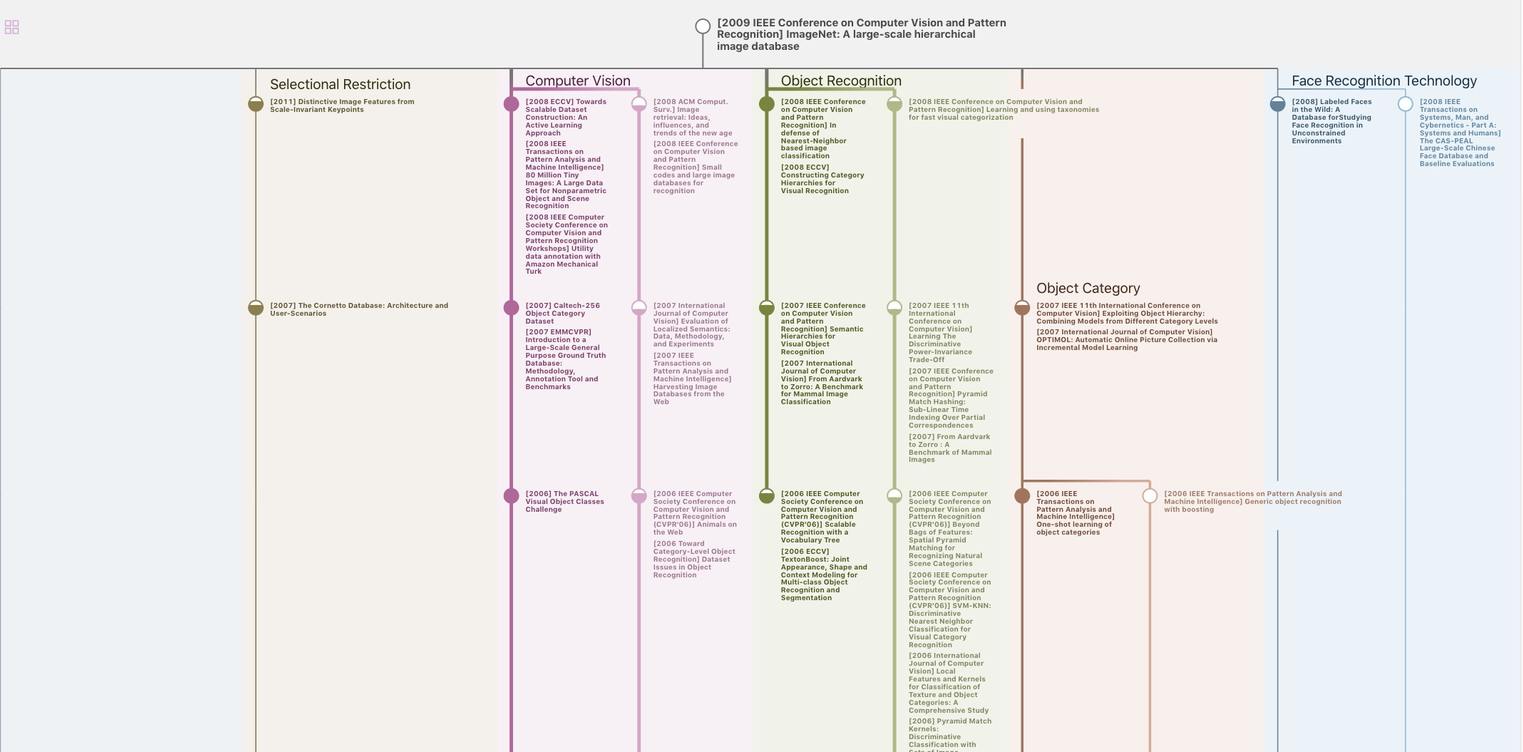
生成溯源树,研究论文发展脉络
Chat Paper
正在生成论文摘要