Improving auditory attention decoding performance of linear and non-linear methods using state-space model
2020 IEEE INTERNATIONAL CONFERENCE ON ACOUSTICS, SPEECH, AND SIGNAL PROCESSING(2020)
Abstract
Identifying the target speaker in hearing aid applications is crucial to improve speech understanding. Recent advances in electroencephalography (EEG) have shown that it is possible to identify the target speaker from single-trial EEG recordings using auditory attention decoding (AAD) methods. AAD methods reconstruct the attended speech envelope from EEG recordings, based on a linear least-squares cost function or non-linear neural networks, and then directly compare the reconstructed envelope with the speech envelopes of speakers to identify the attended speaker using Pearson correlation coefficients. Since these correlation coefficients are highly fluctuating, for a reliable decoding a large correlation window is used, which causes a large processing delay. In this paper, we investigate a state-space model using correlation coefficients obtained with a small correlation window to improve the decoding performance of the linear and the non-linear AAD methods. The experimental results show that the state-space model significantly improves the decoding performance.
MoreTranslated text
Key words
auditory attention decoding, state-space model, neural network, EEG signal, brain computer interface
AI Read Science
Must-Reading Tree
Example
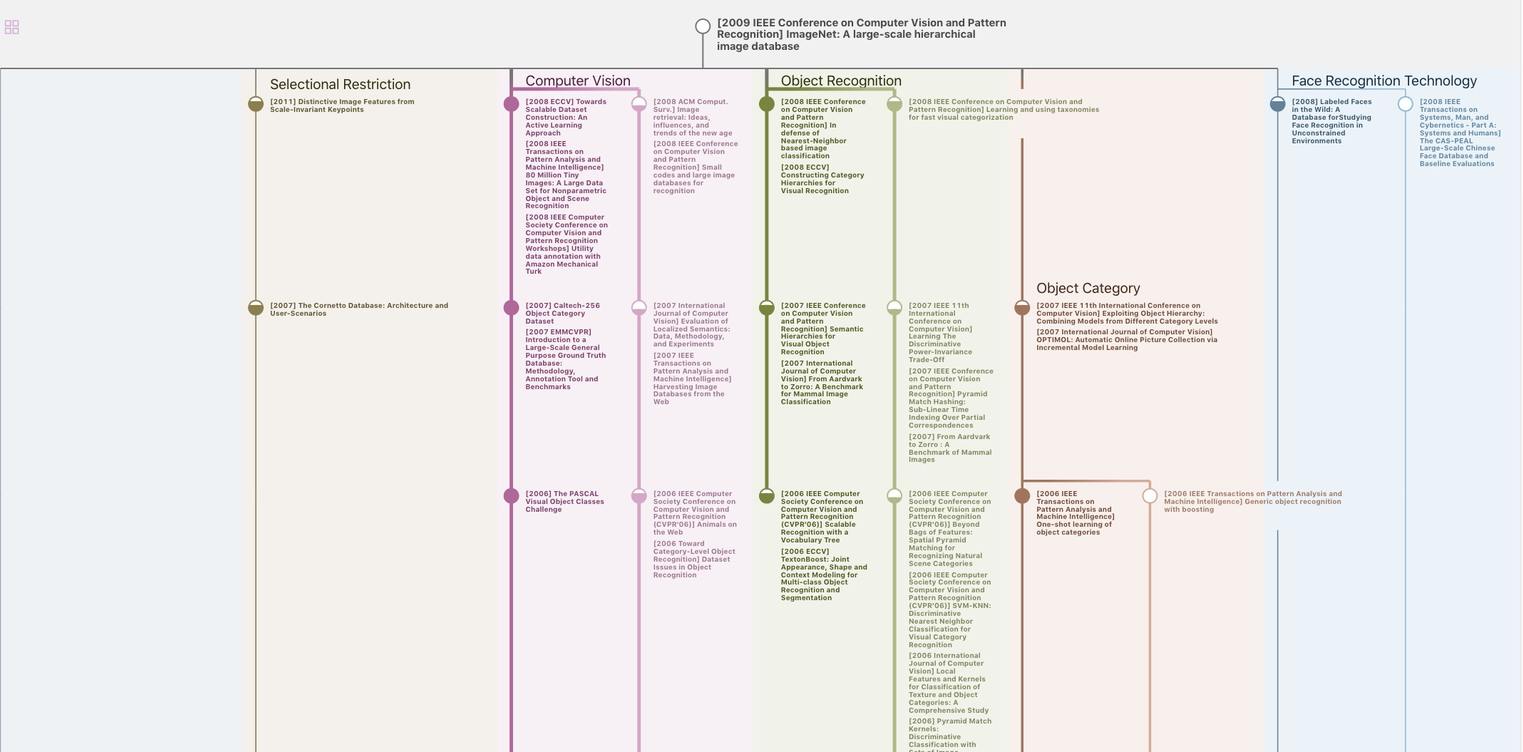
Generate MRT to find the research sequence of this paper
Chat Paper
Summary is being generated by the instructions you defined