Learning Cross-domain Semantic-Visual Relationships for Transductive Zero-Shot Learning
PATTERN RECOGNITION(2023)
Abstract
Zero-Shot Learning (ZSL) learns models for recognizing new classes. One of the main challenges in ZSL is the domain discrepancy caused by the category inconsistency between training and testing data. Domain adaptation is the most intuitive way to address this challenge. However, existing domain adaptation techniques cannot be directly applied into ZSL due to the disjoint label space between source and target domains. This work proposes the Transferrable Semantic-Visual Relation (TSVR) approach towards transductive ZSL. TSVR redefines image recognition as predicting the similarity/dissimilarity labels for semantic-visual fusions consisting of class attributes and visual features. After the above transformation, the source and target domains can have the same label space, which hence enables to quantify domain discrepancy. For the redefined problem, the number of similar semantic-visual pairs is significantly smaller than that of dissimilar ones. To this end, we further propose to use Domain-Specific Batch Normalization to align the domain discrepancy.
MoreTranslated text
Key words
Zero -shot learning, Transfer learning, Domain adaptation
AI Read Science
Must-Reading Tree
Example
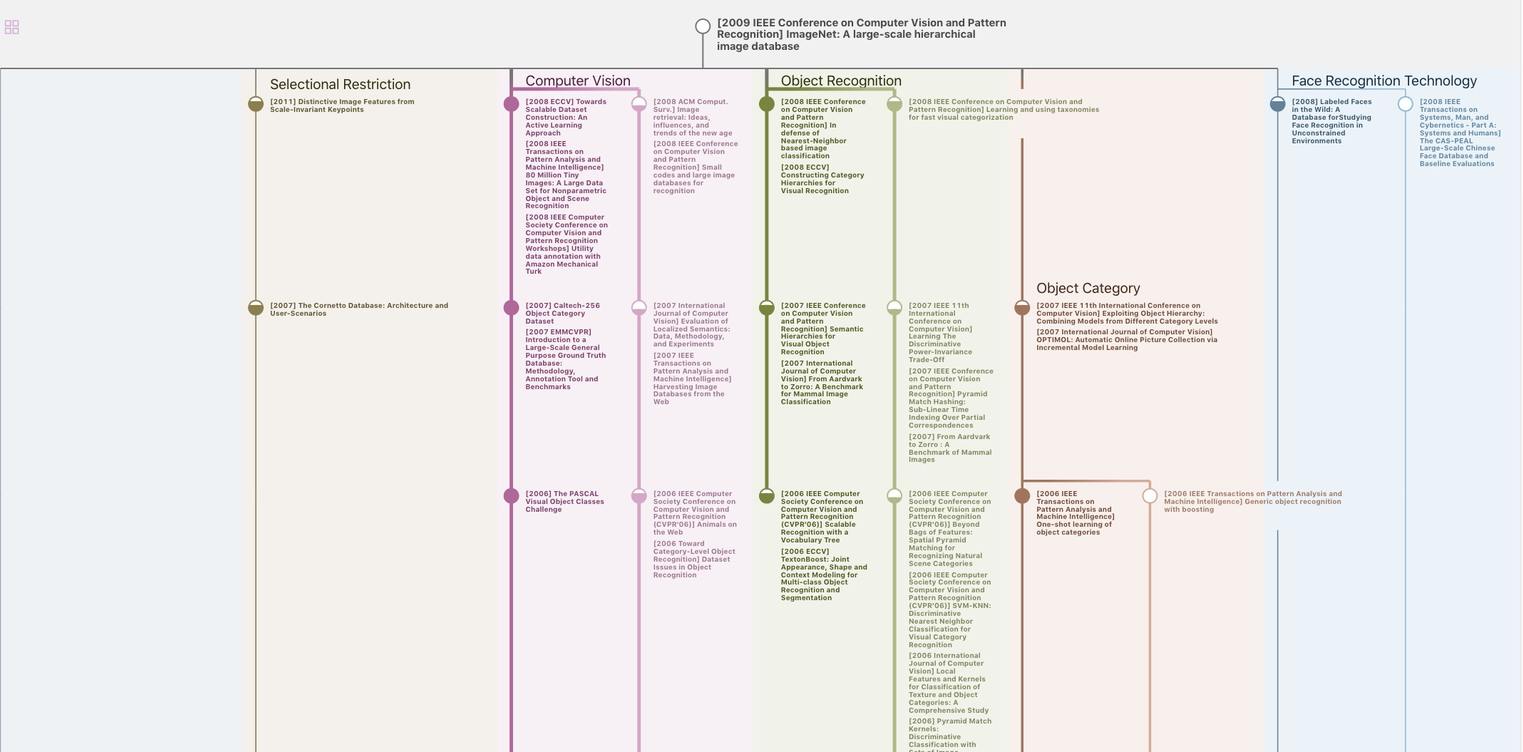
Generate MRT to find the research sequence of this paper
Chat Paper
Summary is being generated by the instructions you defined