Joint Optimization Of Deep Neural Network-Based Dereverberation And Beamforming For Sound Event Detection In Multi-Channel Environments
SENSORS(2020)
摘要
In this paper, we propose joint optimization of deep neural network (DNN)-supported dereverberation and beamforming for the convolutional recurrent neural network (CRNN)-based sound event detection (SED) in multi-channel environments. First, the short-time Fourier transform (STFT) coefficients are calculated from multi-channel audio signals under the noisy and reverberant environments, which are then enhanced by the DNN-supported weighted prediction error (WPE) dereverberation with the estimated masks. Next, the STFT coefficients of the dereverberated multi-channel audio signals are conveyed to the DNN-supported minimum variance distortionless response (MVDR) beamformer in which DNN-supported MVDR beamforming is carried out with the source and noise masks estimated by the DNN. As a result, the single-channel enhanced STFT coefficients are shown at the output and tossed to the CRNN-based SED system, and then, the three modules are jointly trained by the single loss function designed for SED. Furthermore, to ease the difficulty of training a deep learning model for SED caused by the imbalance in the amount of data for each class, the focal loss is used as a loss function. Experimental results show that joint training of DNN-supported dereverberation and beamforming with the SED model under the supervision of focal loss significantly improves the performance under the noisy and reverberant environments.
更多查看译文
关键词
sound event detection,dereverberation,acoustic beamforming,convolutional recurrent neural network,joint optimization
AI 理解论文
溯源树
样例
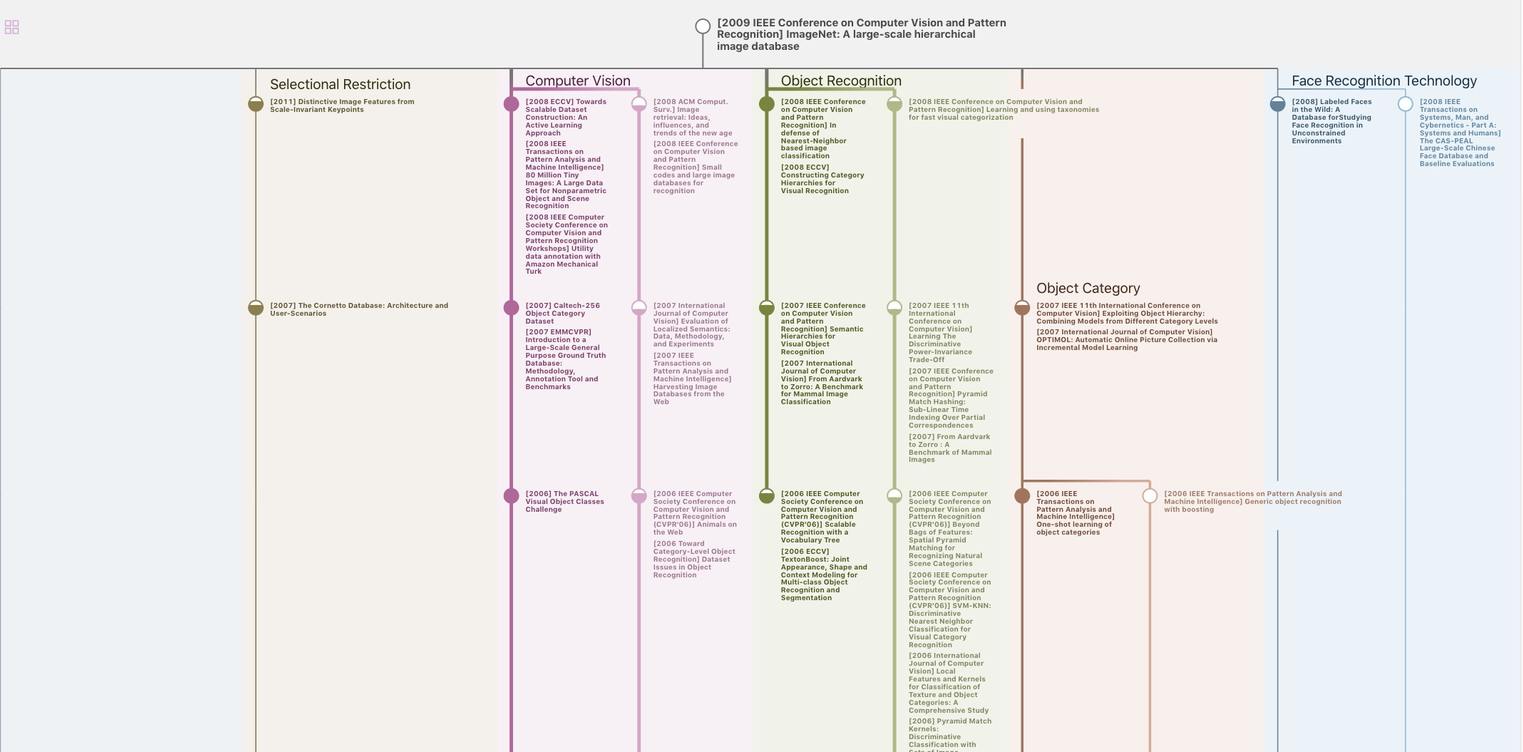
生成溯源树,研究论文发展脉络
Chat Paper
正在生成论文摘要