CPFNet: Context Pyramid Fusion Network for Medical Image Segmentation
IEEE Transactions on Medical Imaging(2020)
摘要
Accurate and automatic segmentation of medical images is a crucial step for clinical diagnosis and analysis. The convolutional neural network (CNN) approaches based on the U-shape structure have achieved remarkable performances in many different medical image segmentation tasks. However, the context information extraction capability of single stage is insufficient in this structure, due to the problems such as imbalanced class and blurred boundary. In this paper, we propose a novel Context Pyramid Fusion Network (named CPFNet) by combining two pyramidal modules to fuse global/multi-scale context information. Based on the U-shape structure, we first design multiple global pyramid guidance (GPG) modules between the encoder and the decoder, aiming at providing different levels of global context information for the decoder by reconstructing skip-connection. We further design a scale-aware pyramid fusion (SAPF) module to dynamically fuse multi-scale context information in high-level features. These two pyramidal modules can exploit and fuse rich context information progressively. Experimental results show that our proposed method is very competitive with other state-of-the-art methods on four different challenging tasks, including skin lesion segmentation, retinal linear lesion segmentation, multi-class segmentation of thoracic organs at risk and multi-class segmentation of retinal edema lesions.
更多查看译文
关键词
Image segmentation,Decoding,Task analysis,Lesions,Feature extraction,Medical diagnostic imaging
AI 理解论文
溯源树
样例
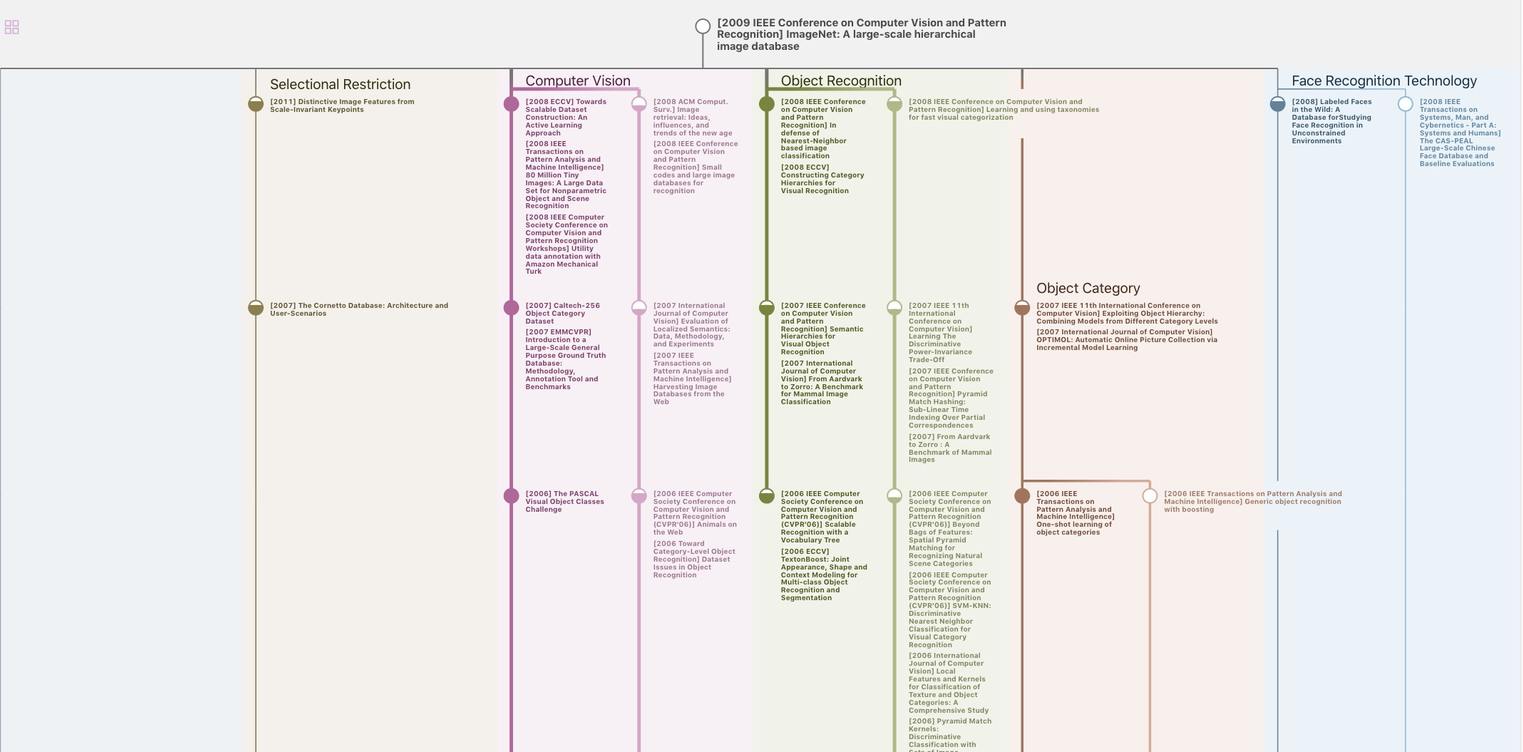
生成溯源树,研究论文发展脉络
Chat Paper
正在生成论文摘要