Generation of Consistent Sets of Multi-Label Classification Rules with a Multi-Objective Evolutionary Algorithm
GECCO '20: Genetic and Evolutionary Computation Conference Cancún Mexico July, 2020(2020)
摘要
Multi-label classification consists in classifying an instance into two or more classes simultaneously. Recently, the interest in interpretable classification models have grown, partially as a consequence of regulations such as the General Data Protection Regulation. In this context, we propose a multi-objective evolutionary algorithm that generates multiple rule-based multi-label classification models, allowing users to choose among models that offer different compromises between predictive power and interpretability. The most important contributions of this work are: the generated models are based on sets (unordered collection) of rules and the rule creation mechanism employs a conflict avoidance algorithm which guarantees that all rules within a model are consistent with each other. We conducted experiments on synthetic and real-world datasets and compared our results with state-of-the-art algorithms in terms of predictive performance (F-Score) and interpretability (model size), and demonstrate that our best models had comparable F-Score and smaller model sizes.
更多查看译文
关键词
classification,algorithm,multi-label,multi-objective
AI 理解论文
溯源树
样例
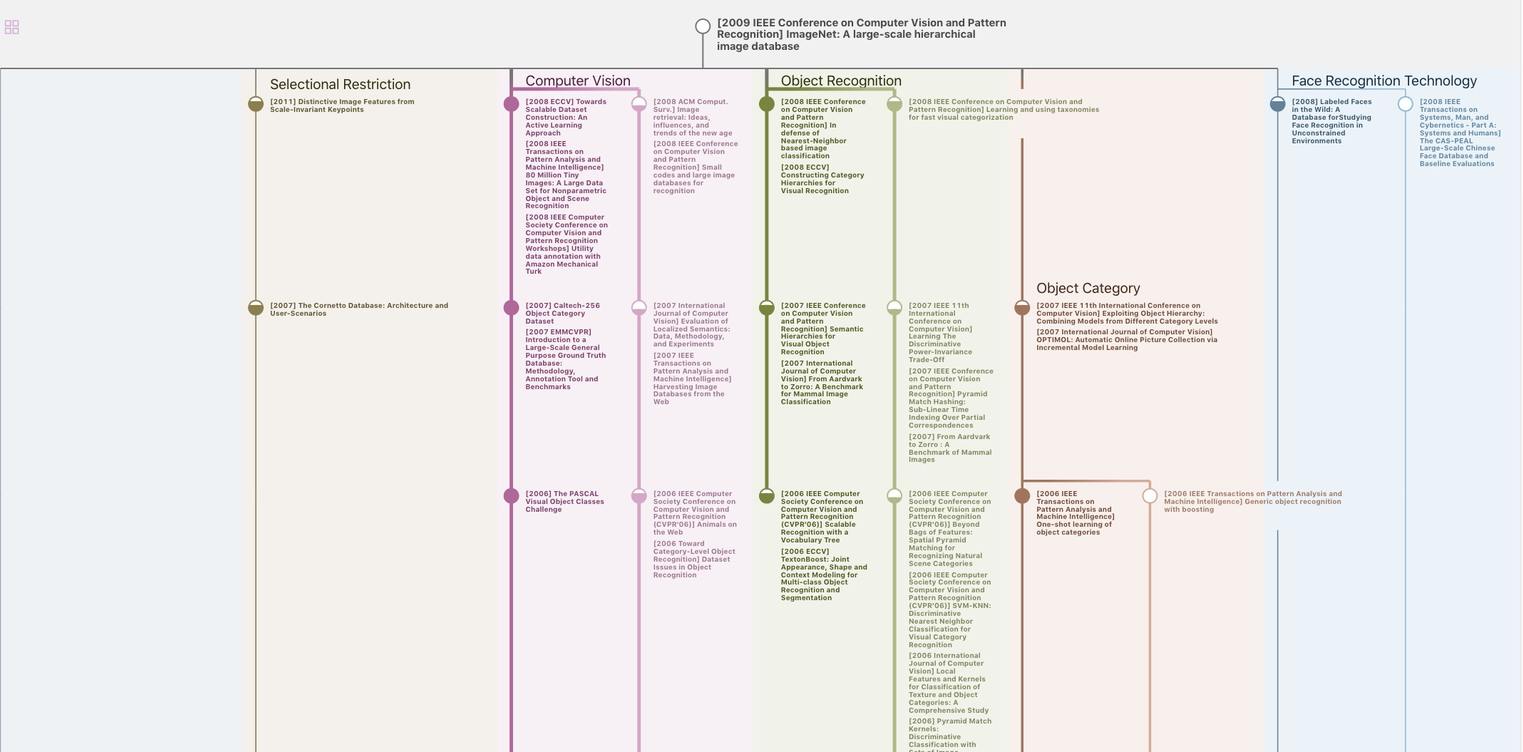
生成溯源树,研究论文发展脉络
Chat Paper
正在生成论文摘要