An Efficient Machine Learning Approach for Accurate Short Term Solar Power Prediction
computational intelligence(2020)
摘要
Solar based electricity generations have experienced a strong and impactful growth in the recent years. The regulation, scheduling, dispatching and unit commitment of intermittent solar power is dependent on the accuracy of the forecasting methods. In this paper, a robust Expanded Extreme Learning Machine (EELM) is proposed to accurately predict the solar power for different time horizon and weather condition. The proposed EELM technique has no randomness due to the absence of random input layer weights and takes very less time to predict the solar power efficiently. The performance of the proposed EELM is validated through historical data collected from National Renewable Energy Laboratory (NREL) through various performance metrics. The efficacy of the proposed EELM method is evaluated against basic ELM and Functional Link Neural Network (FLNN) for 5 minute and 1 hour ahead time horizon.
更多查看译文
关键词
Extreme Learning Machine (ELM),Solar Forecasting,Functional Link Neural Network (FLNN)
AI 理解论文
溯源树
样例
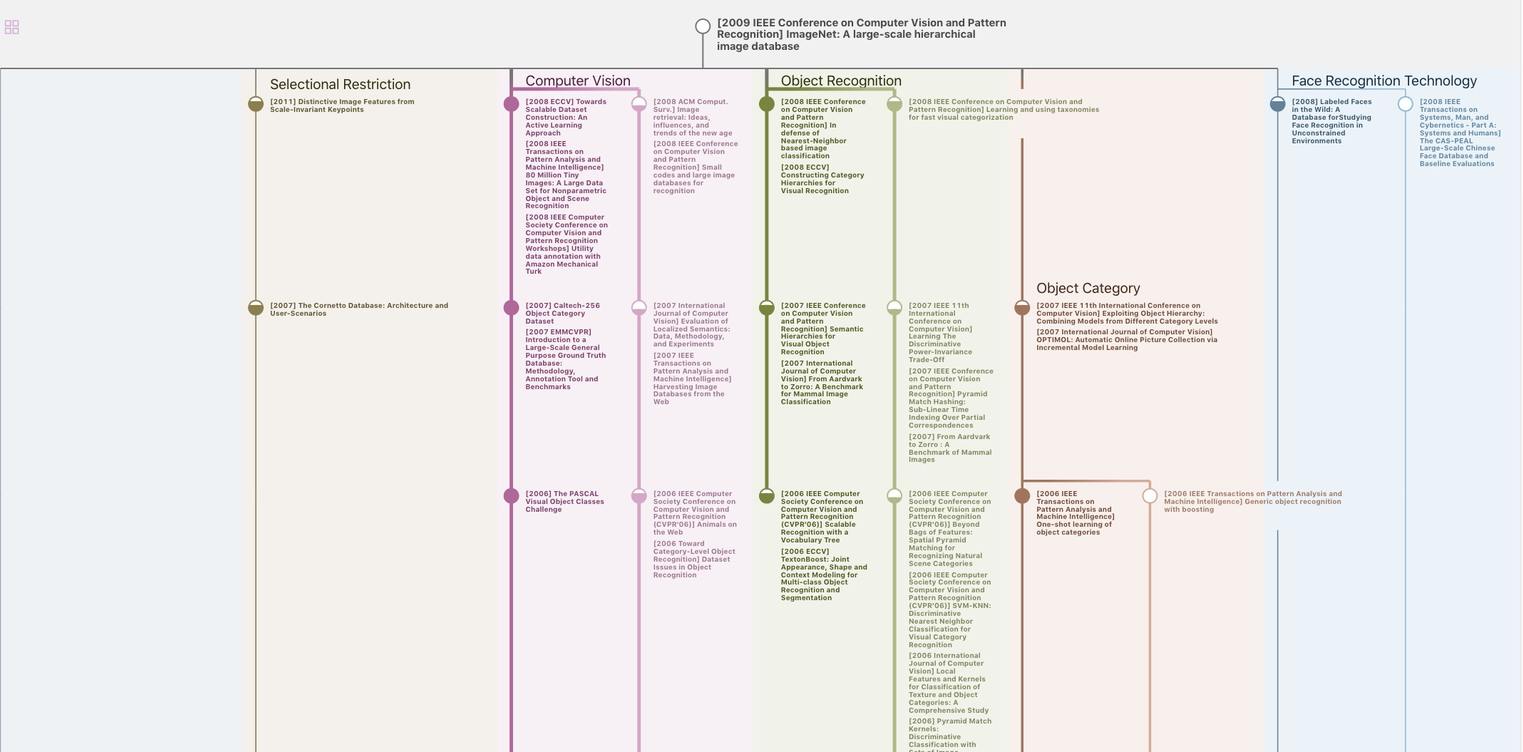
生成溯源树,研究论文发展脉络
Chat Paper
正在生成论文摘要