Last Round Convergence and No-Instant Regret in Repeated Games with Asymmetric Information
arxiv(2023)
摘要
This paper considers repeated games in which one player has more information about the game than the other players. In particular, we investigate repeated two-player zero-sum games where only the column player knows the payoff matrix A of the game. Suppose that while repeatedly playing this game, the row player chooses her strategy at each round by using a no-regret algorithm to minimize her (pseudo) regret. We develop a no-instant-regret algorithm for the column player to exhibit last round convergence to a minimax equilibrium. We show that our algorithm is efficient against a large set of popular no-regret algorithms of the row player, including the multiplicative weight update algorithm, the online mirror descent method/follow-the-regularized-leader, the linear multiplicative weight update algorithm, and the optimistic multiplicative weight update.
更多查看译文
关键词
repeated games,last round convergence,asymmetric information,no-instant
AI 理解论文
溯源树
样例
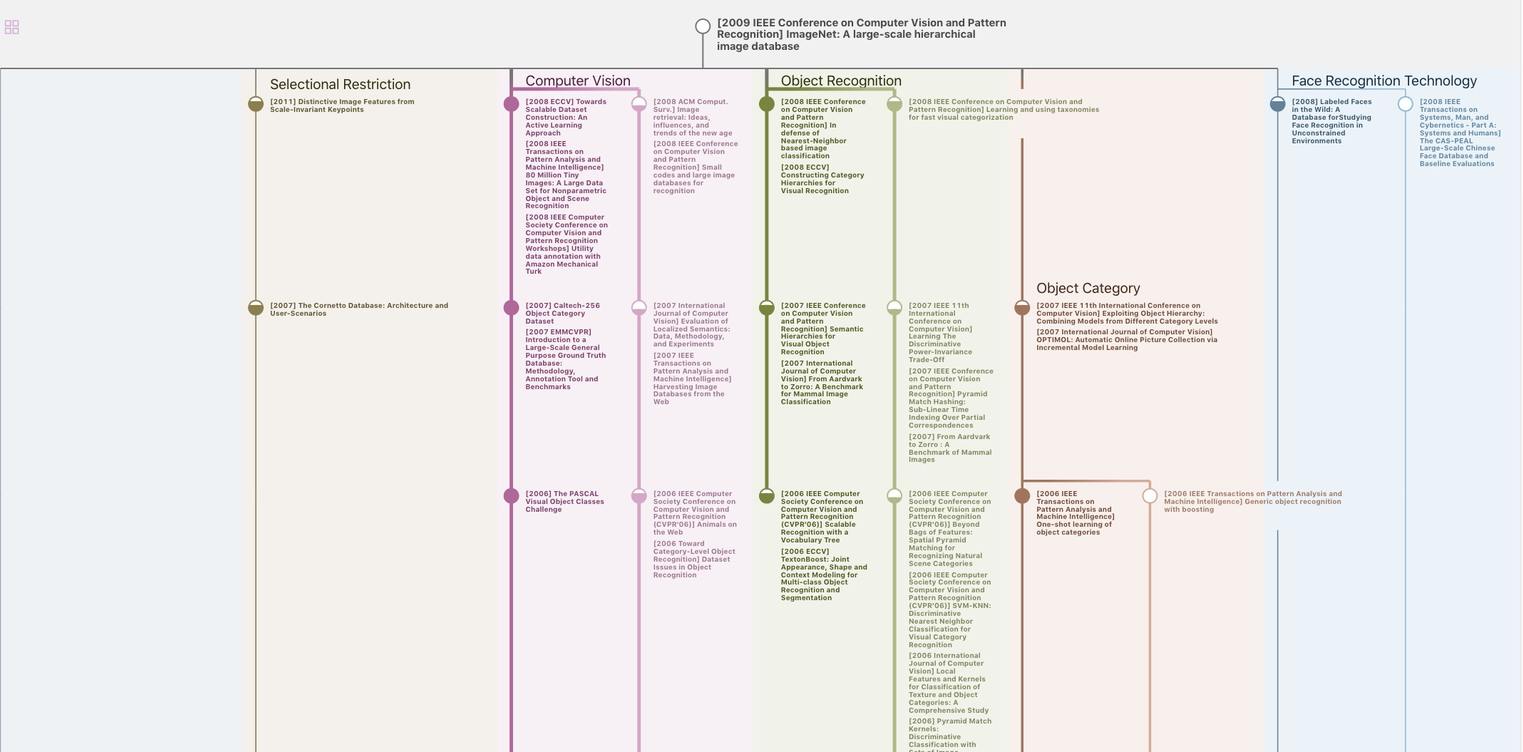
生成溯源树,研究论文发展脉络
Chat Paper
正在生成论文摘要