Real-time 3D object proposal generation and classification under limited processing resources
Robotics and Autonomous Systems(2020)
摘要
The task of detecting 3D objects is important in various robotic applications. The existing deep learning-based detection techniques have achieved impressive performances. However, these techniques are limited to being run on a graphics processing unit (GPU) in a real-time environment. To achieve real-time 3D object detection with limited computational resources, we propose an efficient detection method based on 3D proposal generation and classification. The proposal generation is based mainly on point segmentation, while proposal classification is performed by a lightweight convolution neural network (CNN). KITTI datasets are then used to validate our method. It takes only 0.082 s for our method to process one point block with one core of the central processing unit (CPU). In addition to efficiency, the experimental results also demonstrate the capability of the proposed method of producing a competitive performance in object recall and classification.
更多查看译文
关键词
Point cloud segmentation,3D object detection,Optimization,CNN
AI 理解论文
溯源树
样例
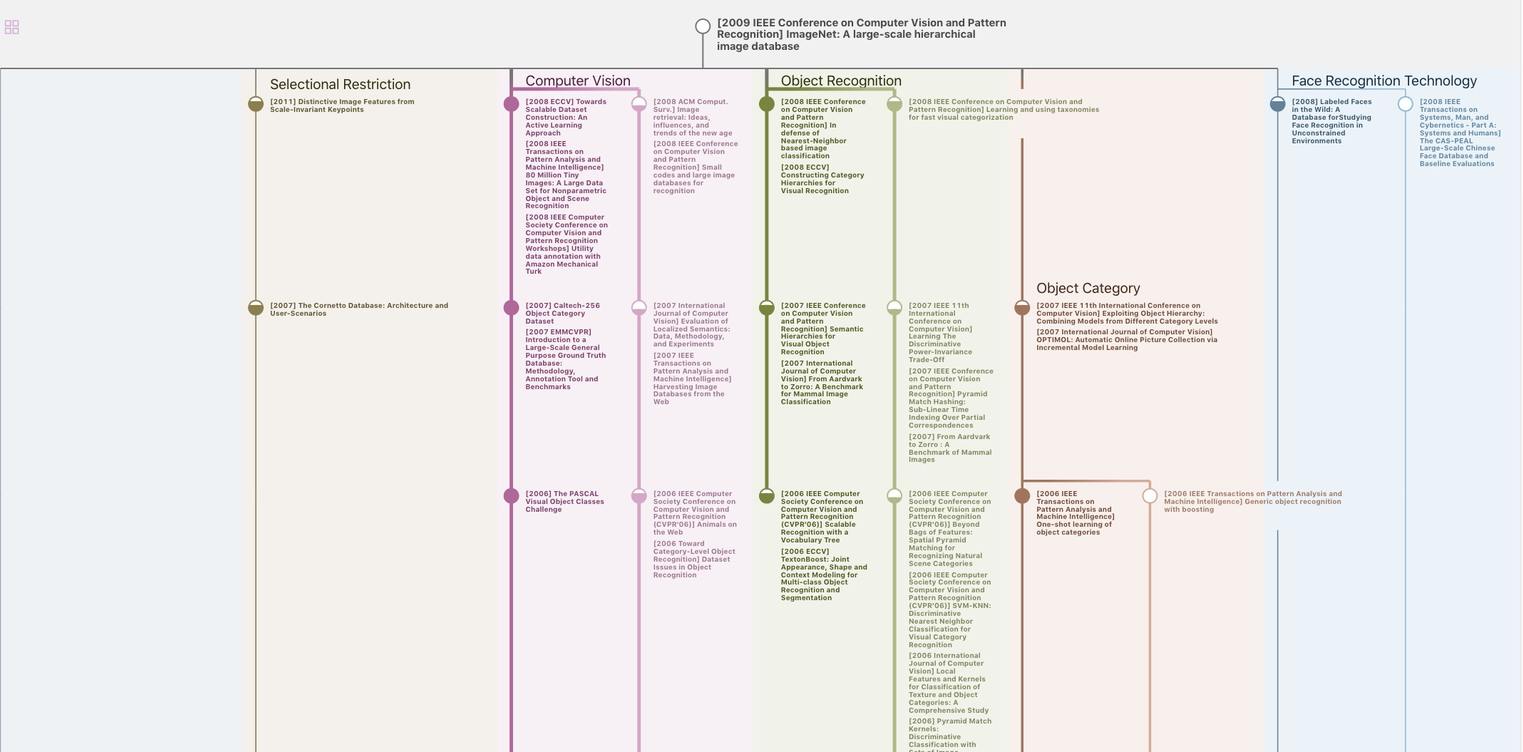
生成溯源树,研究论文发展脉络
Chat Paper
正在生成论文摘要