A Computer Vision Approach for Classifying Isometric Grip Force Exertion Levels.
ERGONOMICS(2020)
摘要
Exposure to high and/or repetitive force exertions can lead to musculoskeletal injuries. However, measuring worker force exertion levels is challenging, and existing techniques can be intrusive, interfere with human-machine interface, and/or limited by subjectivity. In this work, computer vision techniques are developed to detect isometric grip exertions using facial videos and wearable photoplethysmogram. Eighteen participants (19-24 years) performed isometric grip exertions at varying levels of maximum voluntary contraction. Novel features that predict forces were identified and extracted from video and photoplethysmogram data. Two experiments with two (High/Low) and three (0%MVC/50%MVC/100%MVC) labels were performed to classify exertions. The Deep Neural Network classifier performed the best with 96% and 87% accuracy for two- and three-level classifications, respectively. This approach was robust to leave subjects out during cross-validation (86% accuracy when 3-subjects were left out) and robust to noise (i.e. 89% accuracy for correctly classifying talking activities as low force exertions). Practitioner summary: Forceful exertions are contributing factors to musculoskeletal injuries, yet it remains difficult to measure in work environments. This paper presents an approach to estimate force exertion levels, which is less distracting to workers, easier to implement by practitioners, and could potentially be used in a wide variety of workplaces.
更多查看译文
关键词
Computer vision,high force exertions,facial expressions,machine learning
AI 理解论文
溯源树
样例
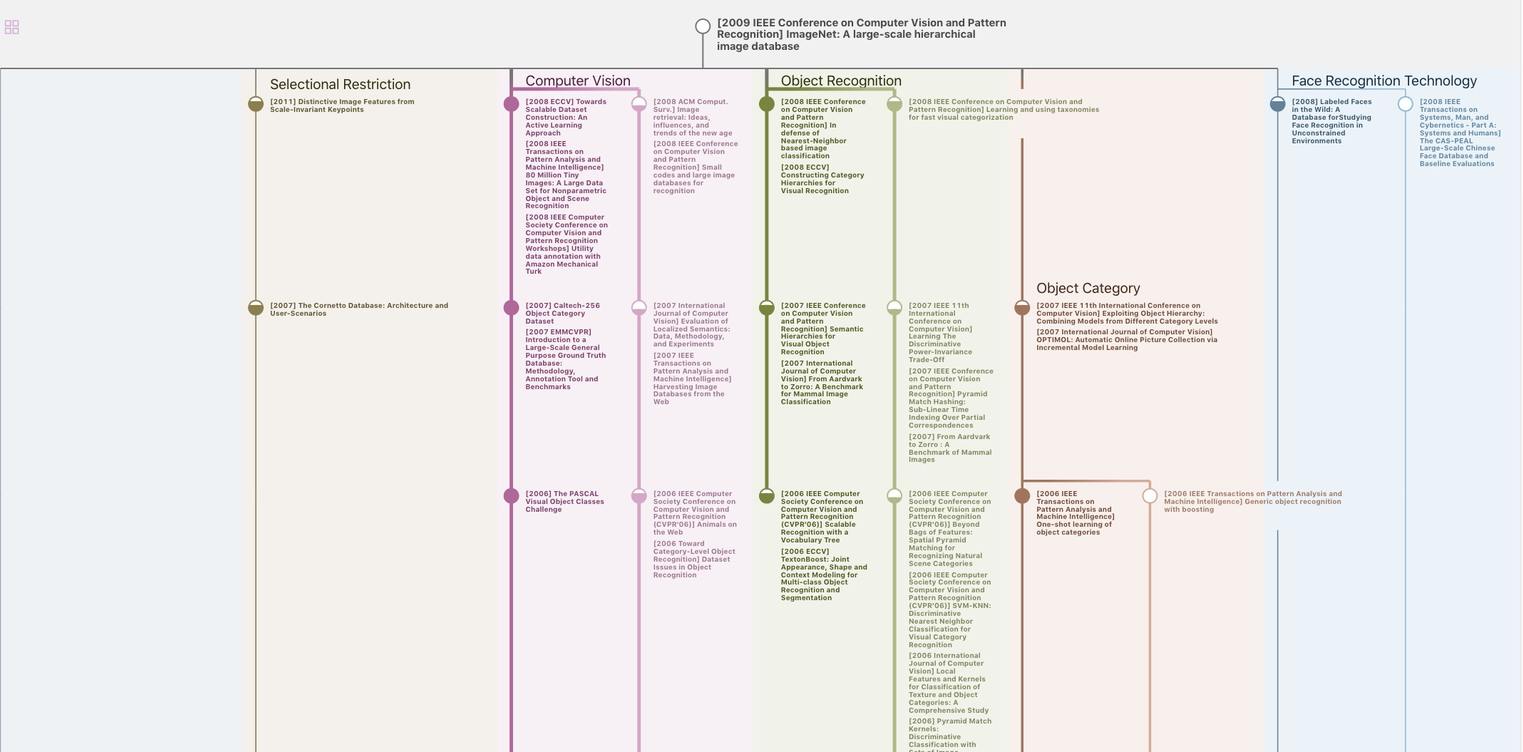
生成溯源树,研究论文发展脉络
Chat Paper
正在生成论文摘要