Active Community Detection With Maximal Expected Model Change
INTERNATIONAL CONFERENCE ON ARTIFICIAL INTELLIGENCE AND STATISTICS, VOL 108(2020)
摘要
We present a novel active learning algorithm for community detection on networks. Our proposed algorithm uses a Maximal Expected Model Change (MEMC) criterion for querying network nodes label assignments. MEMC detects nodes that maximally change the community assignment likelihood model following a query. Our method is inspired by detection in the benchmark Stochastic Block Model (SBM), where we provide sample complexity analysis and empirical study with SBM and real network data for binary as well as for the multi-class settings. The analysis also covers the most challenging case of sparse degree and below-detection-threshold SBMs, where we observe a super-linear error reduction. MEMC is shown to be superior to the random selection baseline and other state-of-the-art active learners.
更多查看译文
关键词
Change Detection,Ensemble Learning,Active Learning,Semi-Supervised Learning,Adaptive Algorithms
AI 理解论文
溯源树
样例
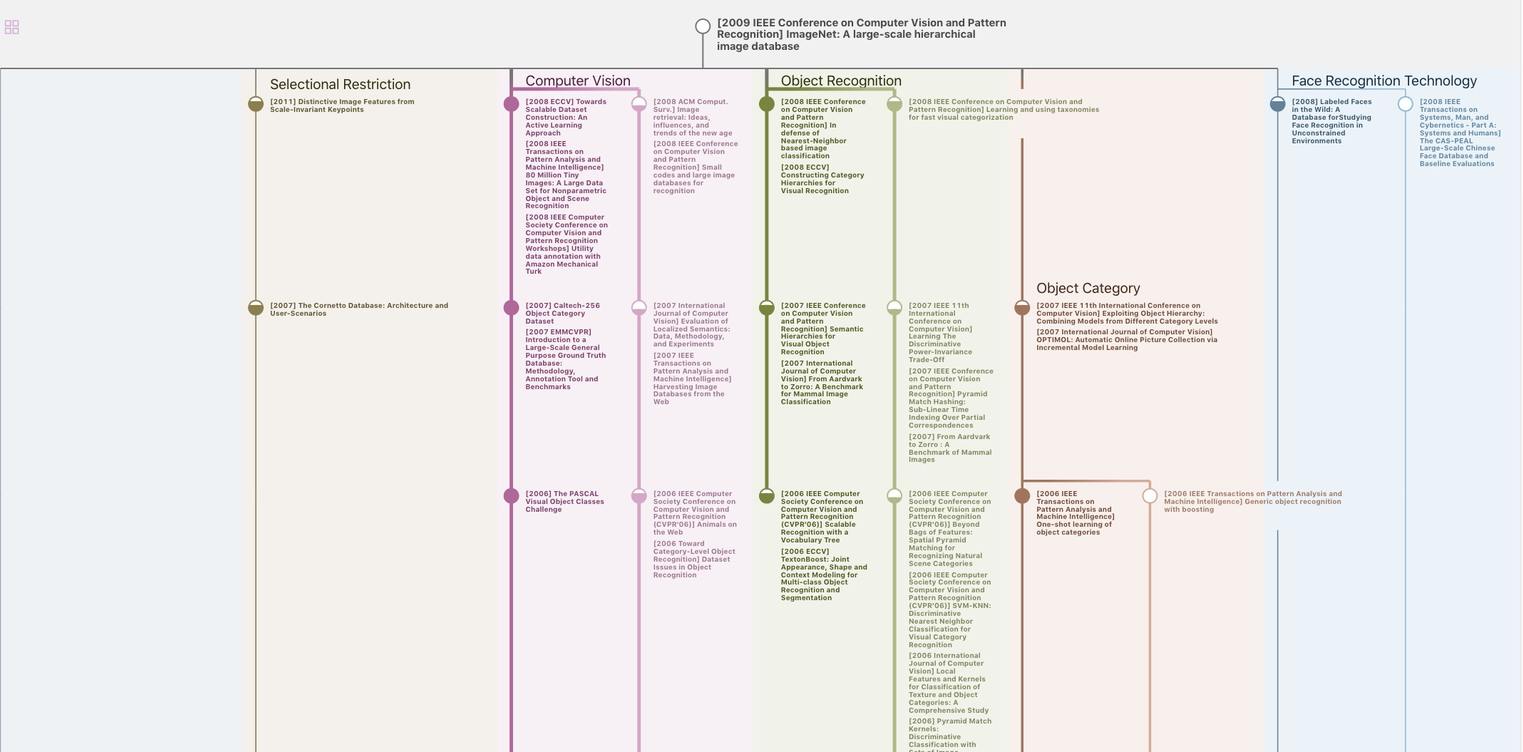
生成溯源树,研究论文发展脉络
Chat Paper
正在生成论文摘要