Quality-Aware Human-Machine Text Extraction for Biocollections using Ensembles of OCRs
2019 15th International Conference on eScience (eScience)(2019)
摘要
Information Extraction (IE) from imaged text is affected by the output quality of the text-recognition process. Misspelled or missing text may propagate errors or even preclude IE. Low confidence in automated methods is the reason why some IE projects rely exclusively on human work (crowdsourcing). That is the case of biological collections (biocollections), where the metadata (Darwin-core Terms) found in digitized labels are transcribed by citizen scientists. In this paper, we present an approach to reduce the number of crowdsourcing tasks required to obtain the transcription of the text found in biocollections' images. By using an ensemble of Optical Character Recognition (OCR) engines - OCRopus, Tesseract, and the Google Cloud OCR - our approach identifies the lines and characters that have a high probability of being correct. This reduces the need for crowdsourced transcription to be done for only low confidence fragments of text. The number of lines to transcribe is also reduced through hybrid human-machine crowdsourcing where the output of the ensemble of OCRs is used as the first "human" transcription of the redundant crowdsourcing process. Our approach was tested in six biocollections (2,966 images), reducing the number of crowdsourcing tasks by 76% (58% due to lines accepted by the ensemble of OCRs and about 18% due to accelerated convergence when using hybrid crowdsourcing). The automatically extracted text presented a character error rate of 0.001 (0.1%).
更多查看译文
关键词
OCR,Optical Character Recognition,Crowdsourcing,Biocollections,Ensemble,Hybrid,Information Extraction,Text Extraction,Human-machine
AI 理解论文
溯源树
样例
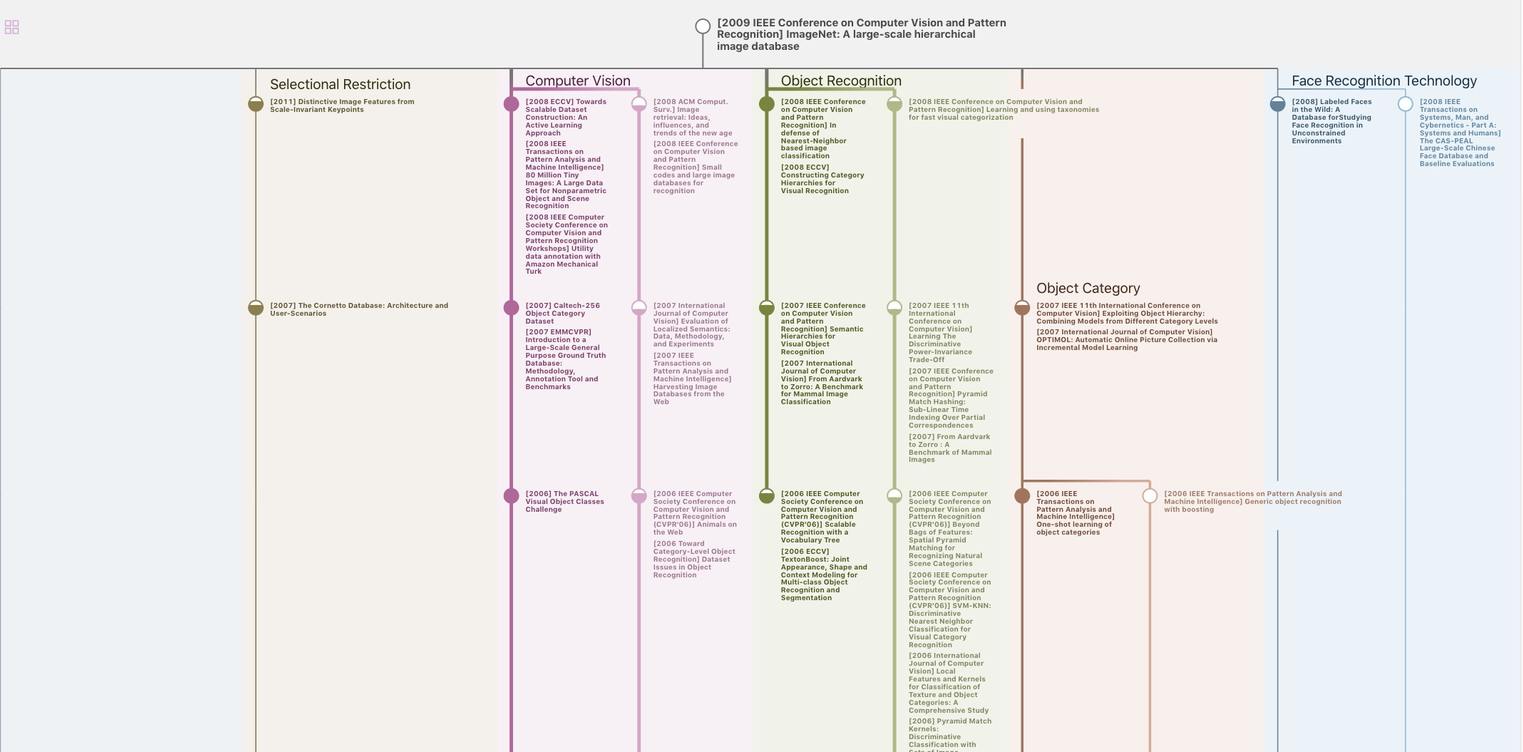
生成溯源树,研究论文发展脉络
Chat Paper
正在生成论文摘要