Brain Mri-Based 3d Convolutional Neural Networks For Classification Of Schizophrenia And Controls
42ND ANNUAL INTERNATIONAL CONFERENCES OF THE IEEE ENGINEERING IN MEDICINE AND BIOLOGY SOCIETY: ENABLING INNOVATIVE TECHNOLOGIES FOR GLOBAL HEALTHCARE EMBC'20(2020)
摘要
Convolutional Neural Network (CNN) has been successfully applied on classification of both natural images and medical images but limited studies applied it to differentiate patients with schizophrenia from healthy controls. Given the subtle, mixed, and sparsely distributed brain atrophy patterns of schizophrenia, the capability of automatic feature learning makes CNN a powerful tool for classifying schizophrenia from controls as it removes the subjectivity in selecting relevant spatial features. To examine the feasibility of applying CNN to classification of schizophrenia and controls based on structural Magnetic Resonance Imaging (MRI), we built 3D CNN models with different architectures and compared their performance with a handcrafted feature-based machine learning approach. Support vector machine (SVM) was used as classifier and Voxel-based Morphometry (VBM) was used as feature for handcrafted feature-based machine learning. 3D CNN models with sequential architecture, inception module and residual module were trained from scratch. CNN models achieved higher cross-validation accuracy than handcrafted feature-based machine learning. Moreover, testing on an independent dataset, 3D CNN models greatly outperformed handcrafted feature-based machine learning. This study underscored the potential of CNN for identifying patients with schizophrenia using 3D brain MR images and paved the way for imaging-based individual-level diagnosis and prognosis in psychiatric disorders.
更多查看译文
关键词
Brain,Humans,Magnetic Resonance Imaging,Neural Networks, Computer,Schizophrenia,Support Vector Machine
AI 理解论文
溯源树
样例
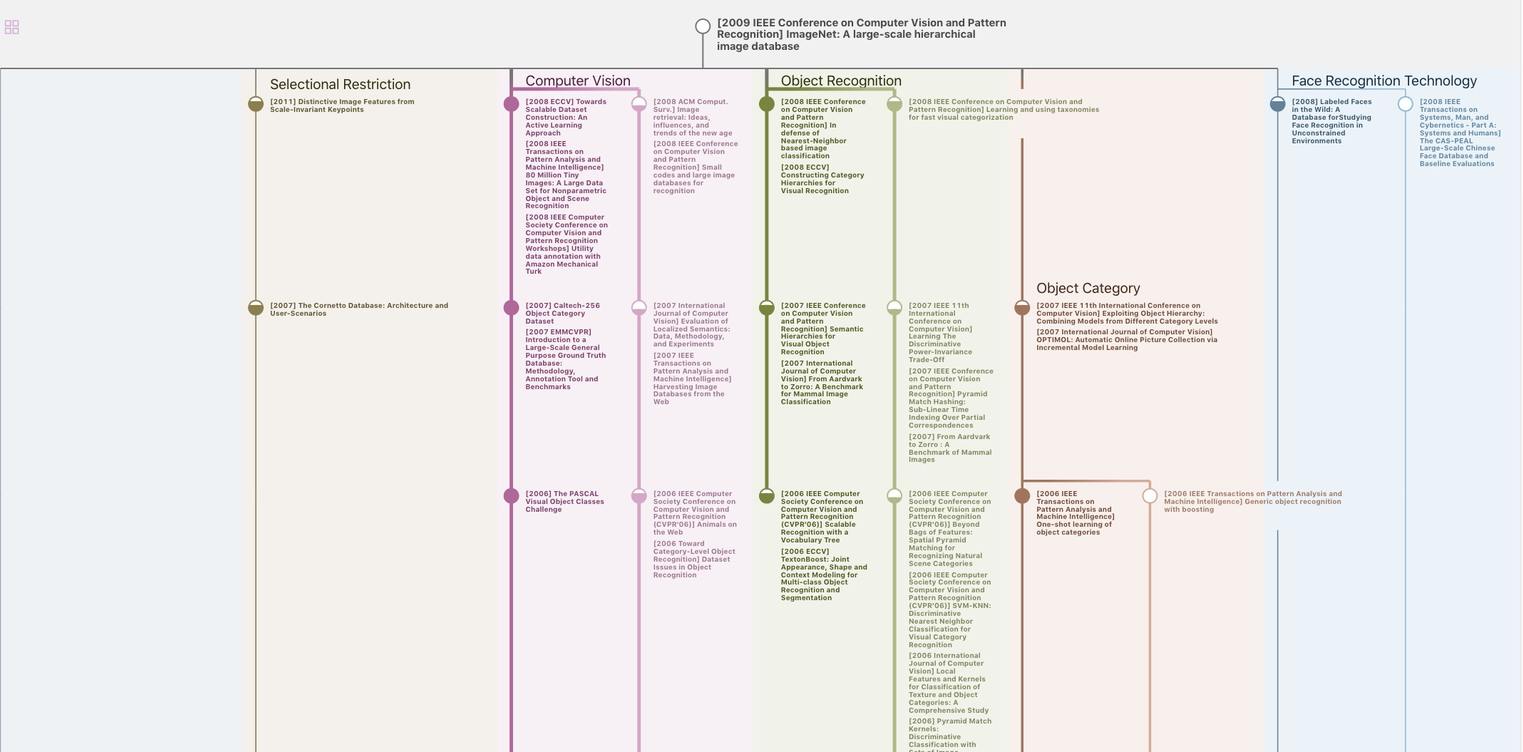
生成溯源树,研究论文发展脉络
Chat Paper
正在生成论文摘要