A Hybrid Learning Method for System Identification and Optimal Control
IEEE Transactions on Neural Networks and Learning Systems(2021)
摘要
We present a three-step method to perform system identification and optimal control of nonlinear systems. Our approach is mainly data-driven and does not require active excitation of the system to perform system identification. In particular, it is designed for systems for which only historical data under closed-loop control are available and where historical control commands exhibit low variability. In the first step, simple simulation models of the system are built and run under various conditions. In the second step, a neural network architecture is extensively trained on the simulation outputs to learn the system physics and retrained with historical data from the real system with stopping rules. These constraints avoid overfitting that arises by fitting closed-loop controlled systems. By doing so, we obtain one (or many) system model(s), represented by this architecture, whose behavior can be chosen to match more or less the real system. Finally, state-of-the-art reinforcement learning with a variant of domain randomization and distributed learning is used for optimal control of the system. We first illustrate the model identification strategy with a simple example, the pendulum with external torque. We then apply our method to model and optimize the control of a large building facility located in Switzerland. Simulation results demonstrate that this approach generates stable functional controllers that outperform on comfort and energy benchmark rule-based controllers.
更多查看译文
关键词
Building management systems,deep reinforcement learning (RL),optimal control,system identification
AI 理解论文
溯源树
样例
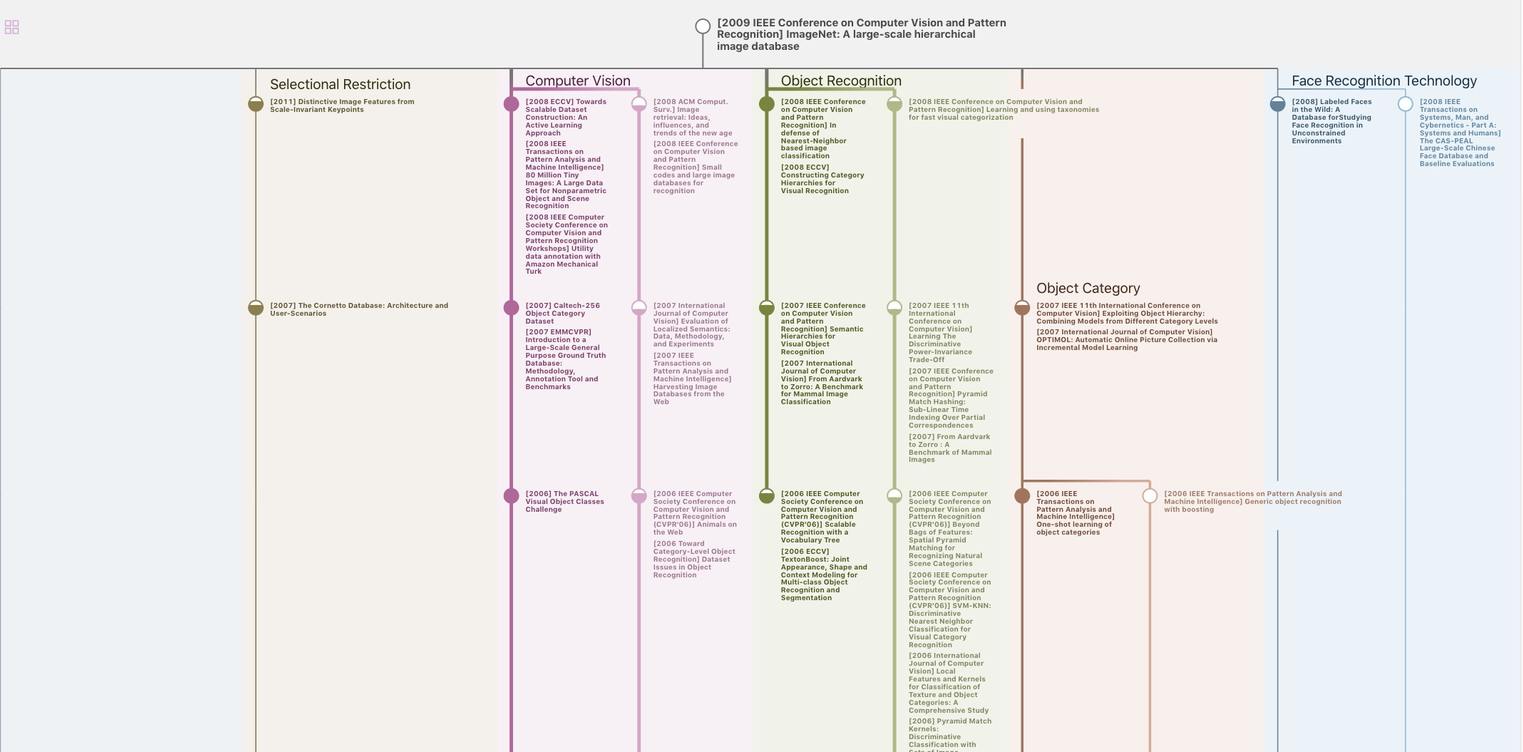
生成溯源树,研究论文发展脉络
Chat Paper
正在生成论文摘要