Evaluation of Feature and Signature based Training Approaches for Malware Classification using Autoencoders
2020 INTERNATIONAL CONFERENCE ON COMMUNICATION SYSTEMS & NETWORKS (COMSNETS)(2020)
摘要
Malware analysis has become a critical and notable area of research importance due to rapid growth in the development and application of internet based systems. Recent advances in artificial intelligence (AI) particularly with data mining enabled the implementation of AI based malware classification and detection systems. AI based malware analysis systems are predominantly signature based and are built on available malware datasets. This paper tries to evaluate the capability of a feature based malware classification using autoencoders. In so doing, this paper presents a new approach for creating a synthetic malware dataset based on signature and features which could be used to train and test both traditional and artificial intelligence based malware detection systems. Various experiments are carried out using autoencoders training on feature based and signature based datasets and tested on a synthetic dataset. The experiments also carried out with multiple datasets and topologies. The experiment results show that the feature based training is proved to be efficient for synthetic, signature and feature based datasets compared to signature based approach. Feature based stacked autoencoders (5-layered) is able to achieve a classification accuracy of 95.6% more than 11.6% when compared with the signature based system which could achieve only 84.6%.
更多查看译文
关键词
malware classification,signature,training approaches,feature
AI 理解论文
溯源树
样例
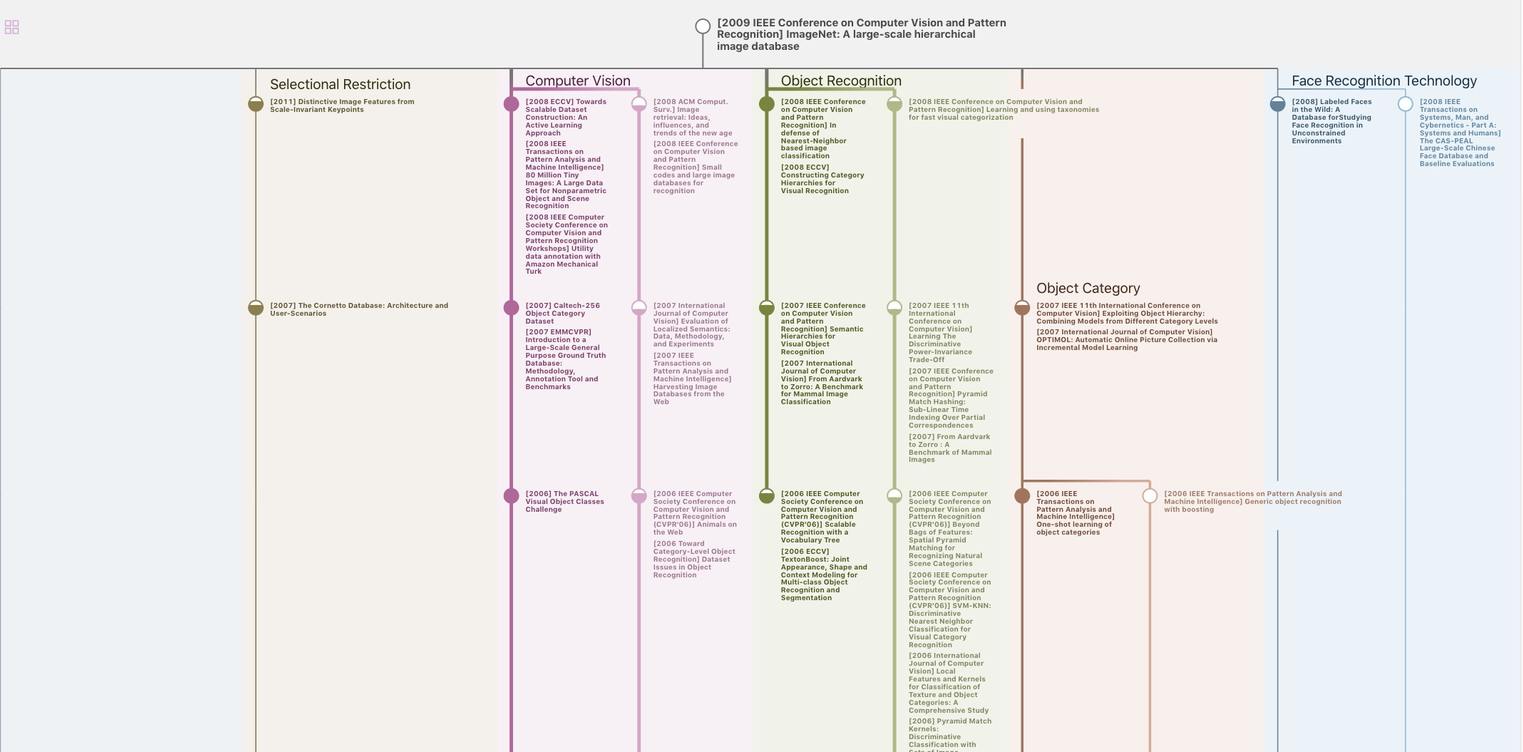
生成溯源树,研究论文发展脉络
Chat Paper
正在生成论文摘要