Towards End-to-End Multilingual Question Answering
INFORMATION SYSTEMS FRONTIERS(2020)
Abstract
Multilingual question answering (MLQA) is a critical part of an accessible natural language interface. However, current solutions demonstrate performance far below that of monolingual systems. We believe that deep learning approaches are likely to improve performance in MLQA drastically. This work aims to discuss the current state-of-the-art and remaining challenges. We outline requirements and suggestions for practical parallel data collection and describe existing methods, benchmarks and datasets. We also demonstrate that a simple translation of texts can be inadequate in case of Arabic, English and German languages (on InsuranceQA and SemEval datasets), and thus more sophisticated models are required. We hope that our overview will re-ignite interest in multilingual question answering, especially with regard to neural approaches.
MoreTranslated text
Key words
Question answering,Multilingual natural language processing,Neural natural language processing,Deep learning,Multilingual question answering,Cross-lingual question answering
AI Read Science
Must-Reading Tree
Example
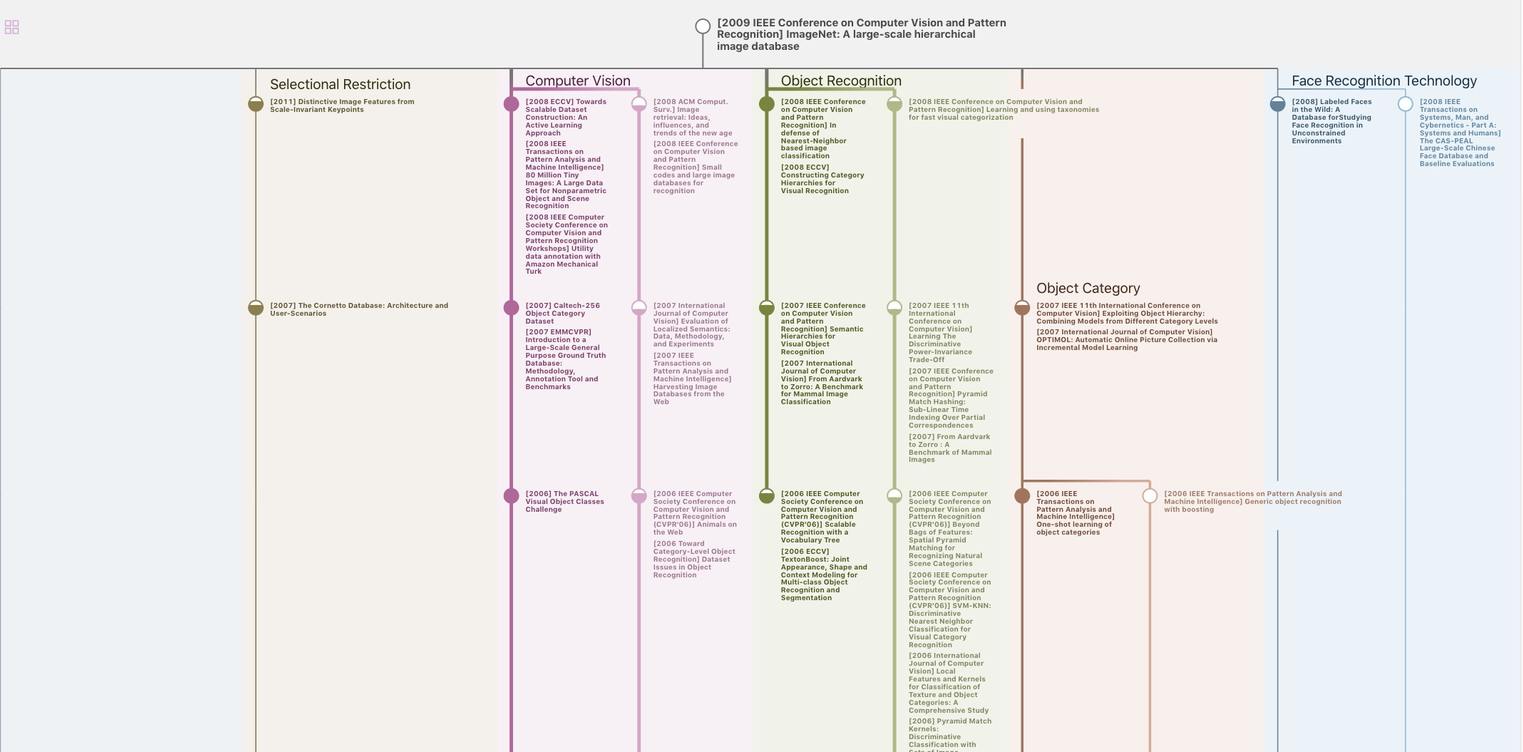
Generate MRT to find the research sequence of this paper
Chat Paper
Summary is being generated by the instructions you defined