Machine-learning prediction for quasiparton distribution function matrix elements
PHYSICAL REVIEW D(2020)
摘要
There have been rapid developments in the direct calculation in lattice QCD (LQCD) of the Bjorken-x dependence of hadron structure through large-momentum effective theory (LaMET). LaMET overcomes the previous limitation of LQCD to moments (that is, integrals over Bjorken x) of hadron structure, allowing LQCD to directly provide the kinematic regions where the experimental values are least known. LaMET requires large-momentum hadron states to minimize its systematics and allow us to reach small-x reliably. This means that very fine lattice spacing to minimize lattice artifacts at order (P(z)a)(n) will become crucial for next-generation LaMET-like structure calculations. Furthermore, such calculations require operators with long Wilson-link displacements, especially in finer lattice units, increasing the communication costs relative to that of the propagator inversion. In this work, we explore whether machine-learning algorithms can make predictions of correlators to reduce the computational cost of these LQCD calculations. We consider two algorithms, gradient-boosting decision tree and linear models, applied to LaMET data, the matrix elements needed to determine the kaon and eta(s) unpolarized parton distribution functions (PDFs), meson distribution amplitude (DA), and the nucleon gluon PDF. We find that both algorithms can reliably predict the target observables with different prediction accuracy and systematic errors. The predictions from smaller displacement z to larger ones work better than those for momentum p due to the higher correlation among the data.
更多查看译文
AI 理解论文
溯源树
样例
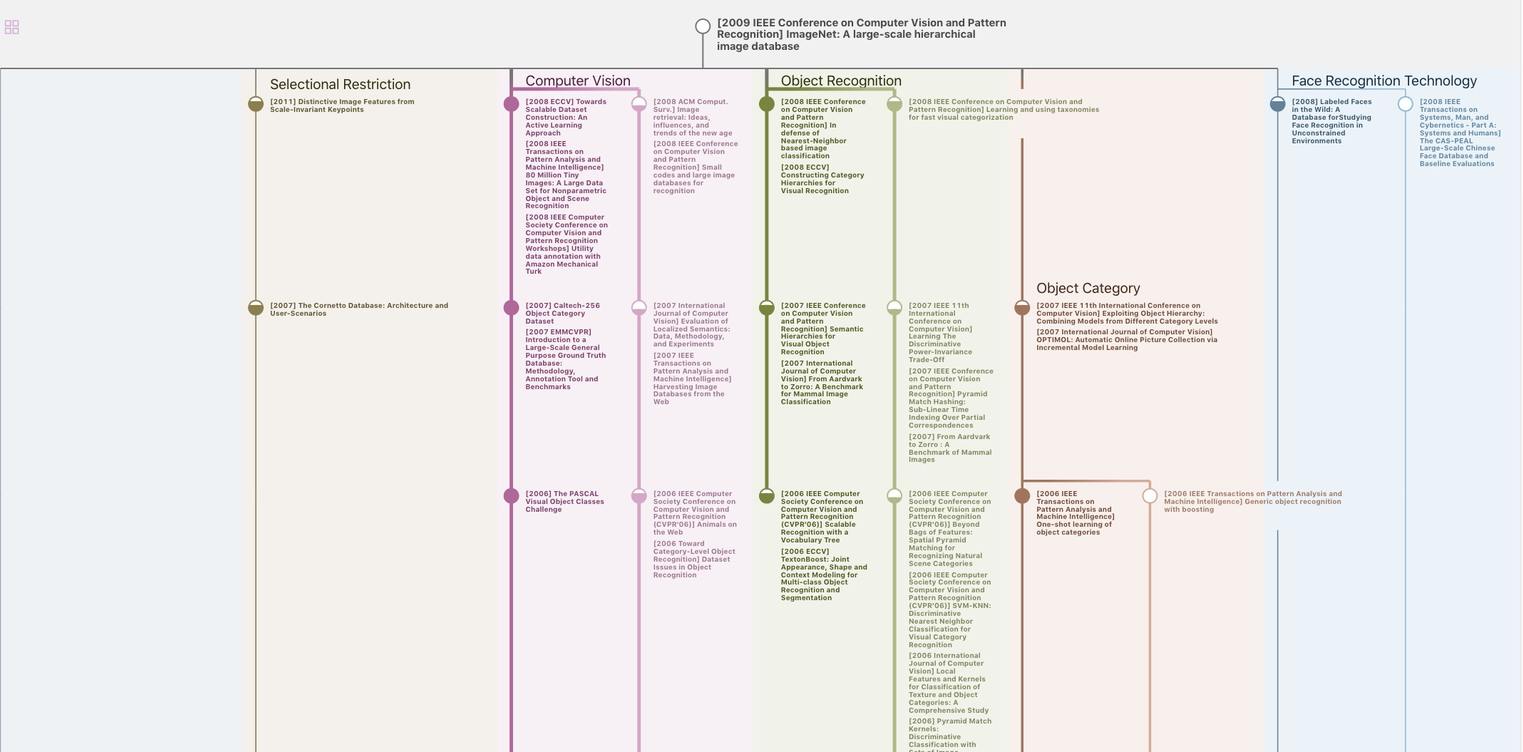
生成溯源树,研究论文发展脉络
Chat Paper
正在生成论文摘要