Forecasting Chinese carbon emissions based on a novel time series prediction method
ENERGY SCIENCE & ENGINEERING(2020)
Abstract
Many calculation methods have been developed to forecast carbon emissions (CE). These methods can be classified into two categories: time series prediction and variables regression. However, time series prediction cannot fully use all CE-related information and variables regression can only predict CE by knowing other variables in advance. To solve these problems, KLS is proposed in this paper which is a fusion of Kalman filter (KF), long short-term memory (LSTM), and support vector machine (SVM). LSTM is used to predict CE as a time series, and 10 of 14 variables are selected by ridge regression (RR) to regress CE by SVM. Finally, KF is used to integrate the results of LSTM and SVM based on their variances. Overall, statistical filtering and deep learning algorithms are used in this paper, which achieved the fusion of time series prediction and variables regression. To confirm the effectiveness of KLS, we trained KLS model by CE from 1965 to 2005 and tested the model by the data from 2006 to 2014. The results showed KLS was more accurate than other four existing methods. Finally, we predicted the CE of China in next decade.
MoreTranslated text
Key words
carbon emission,China,Kalman filtering,long short-term memory,support vector machine
AI Read Science
Must-Reading Tree
Example
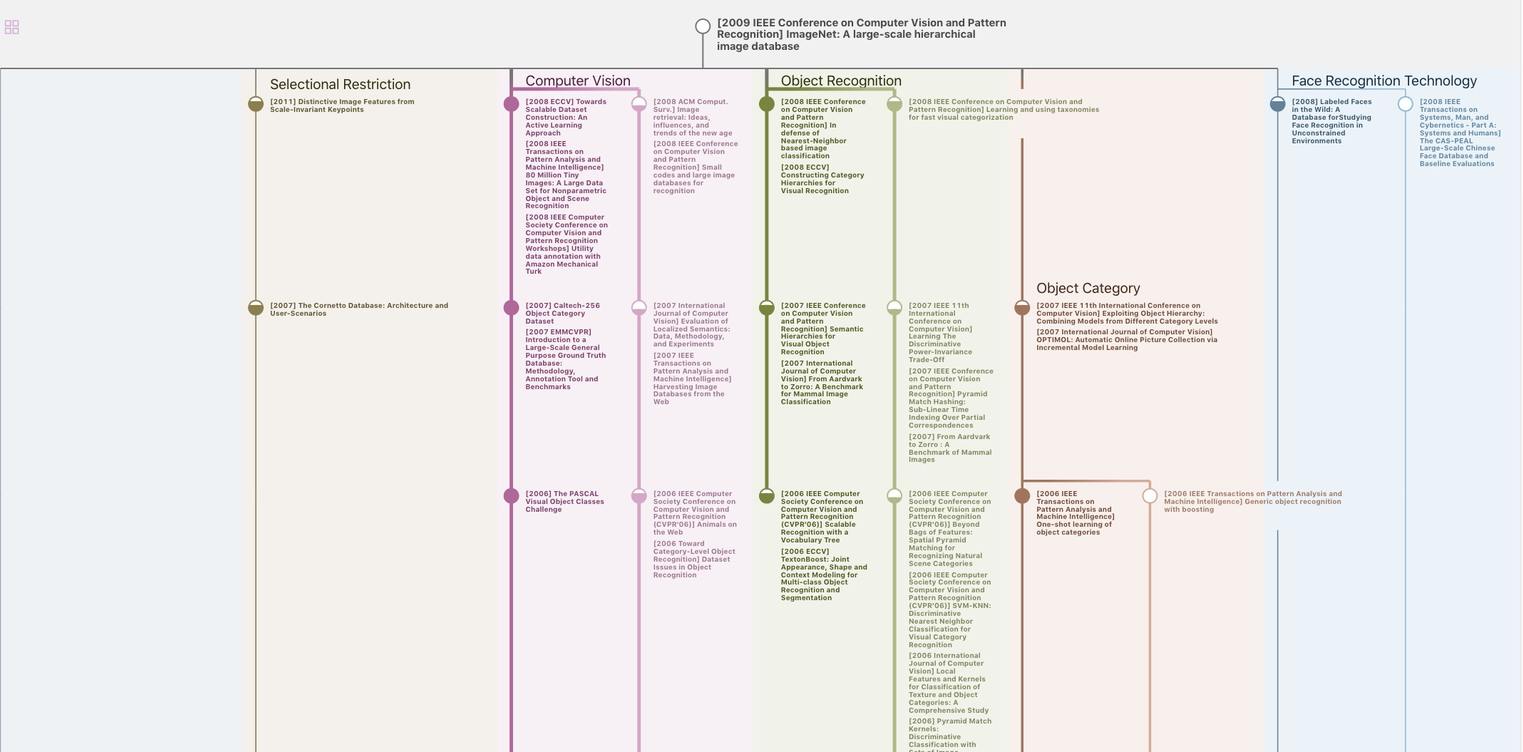
Generate MRT to find the research sequence of this paper
Chat Paper
Summary is being generated by the instructions you defined