Fast adaptive neighbors clustering via embedded clustering
Neurocomputing(2020)
摘要
Recently, spectral clustering (SC) has been gaining more and more attention due to its excellent performance in unsupervised learning. However, the computational complexity of the SC is high. Also, the adjacency graph matrix of the SC is ofen constructed by the Gaussian kernel, so the clustering result is sensitive to the kernel parameter σ. Since most large-scale datasets are high-dimensional and sparse, it is a great challenge to apply the SC to these data. Therefore, a fast adaptive neighbor clustering method based on the embedded clustering (FANCEC) is proposed. First, m anchors are selected from raw data. Next, a bipartite graph matrix Z connecting the raw data and anchors is constructed in a parameter-free manner. Then, the graph embedded data are obtained from raw data by the singular value decomposition (SVD) method. The graph embedded data extracts and combines valid information from raw data while discarding the redundant information. After that, m anchors are selected from graph embedded data, and the adjacency matrix S is initialized. Finally, the adaptive neighbor strategy is used to update matrix S until optimal function convergences. The clustering result of the FANCEC can be obtained directly without the post-processing that is required in the k-means method. The experimental results show that the proposed FANCEC can reduce time-consumption for large-scale data and obtain a good comprehensive clustering effect compared with the traditional SC methods.
更多查看译文
关键词
Fast clustering,Large-scale data,Anchor-based graph embedded,Adaptive neighbor method
AI 理解论文
溯源树
样例
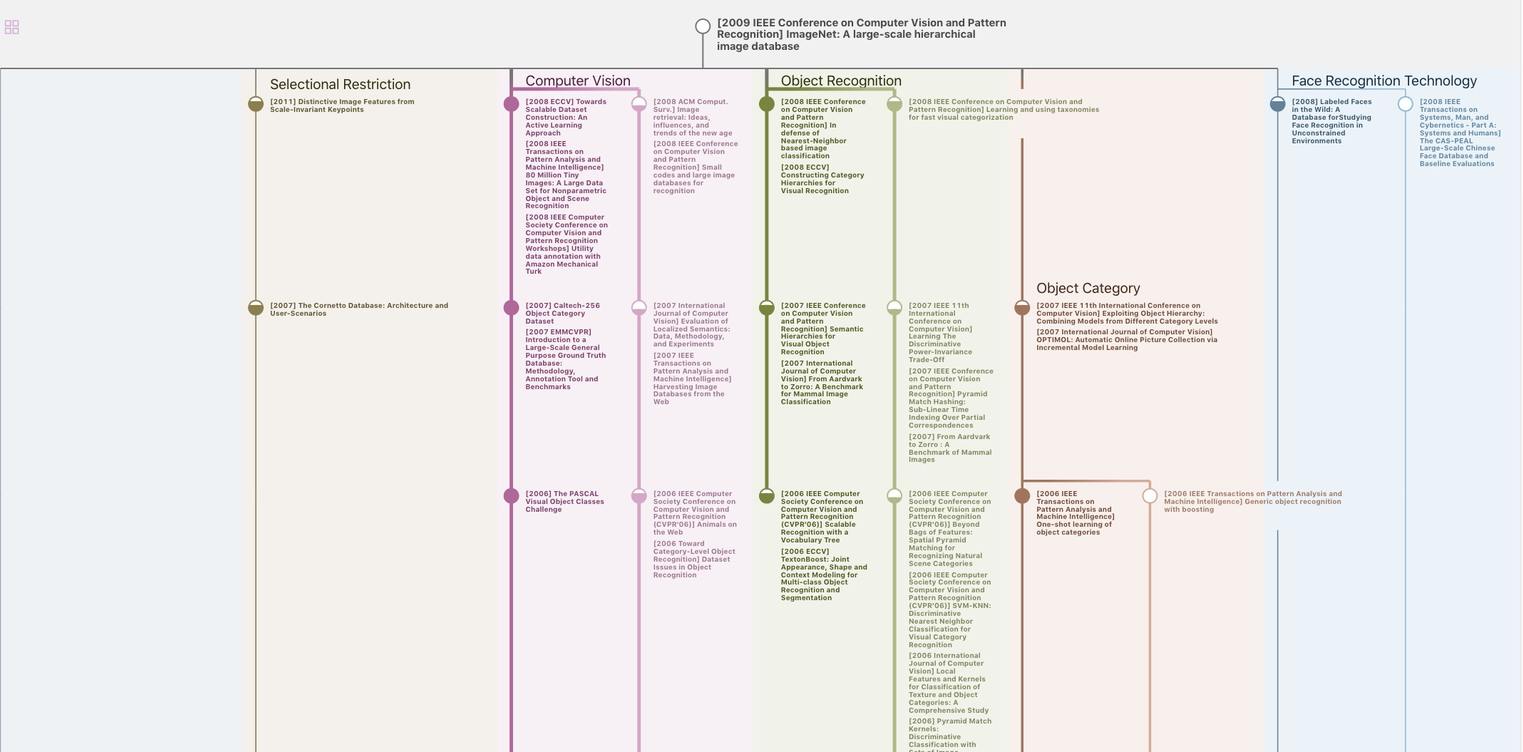
生成溯源树,研究论文发展脉络
Chat Paper
正在生成论文摘要