Knowledge granularity based incremental attribute reduction for incomplete decision systems
International Journal of Machine Learning and Cybernetics(2020)
摘要
Attribute reduction is an important application of rough set theory. With the dynamic changes of data becoming more and more common, traditional attribute reduction, also called static attribute reduction, is no longer efficient. How to update attribute reducts efficiently gets more and more attention. In the light of the variation about the number of objects, we focus on incremental attribute reduction approaches based on knowledge granularity which can be used to measure the uncertainty in incomplete decision systems. We first introduce incremental mechanisms to calculate knowledge granularity for incomplete decision systems when multiple objects vary dynamically. Then, incremental attribute reduction algorithms for incomplete decision systems when adding multiple objects and when deleting multiple objects are proposed respectively. Finally, comparative experiments on different real-life data sets are conducted to demonstrate the effectiveness and efficiency of the proposed incremental algorithms for updating attribute reducts with the variation of multiple objects in incomplete decision systems.
更多查看译文
关键词
Incremental attribute reduction,Knowledge granularity,Incomplete decision system,Rough sets
AI 理解论文
溯源树
样例
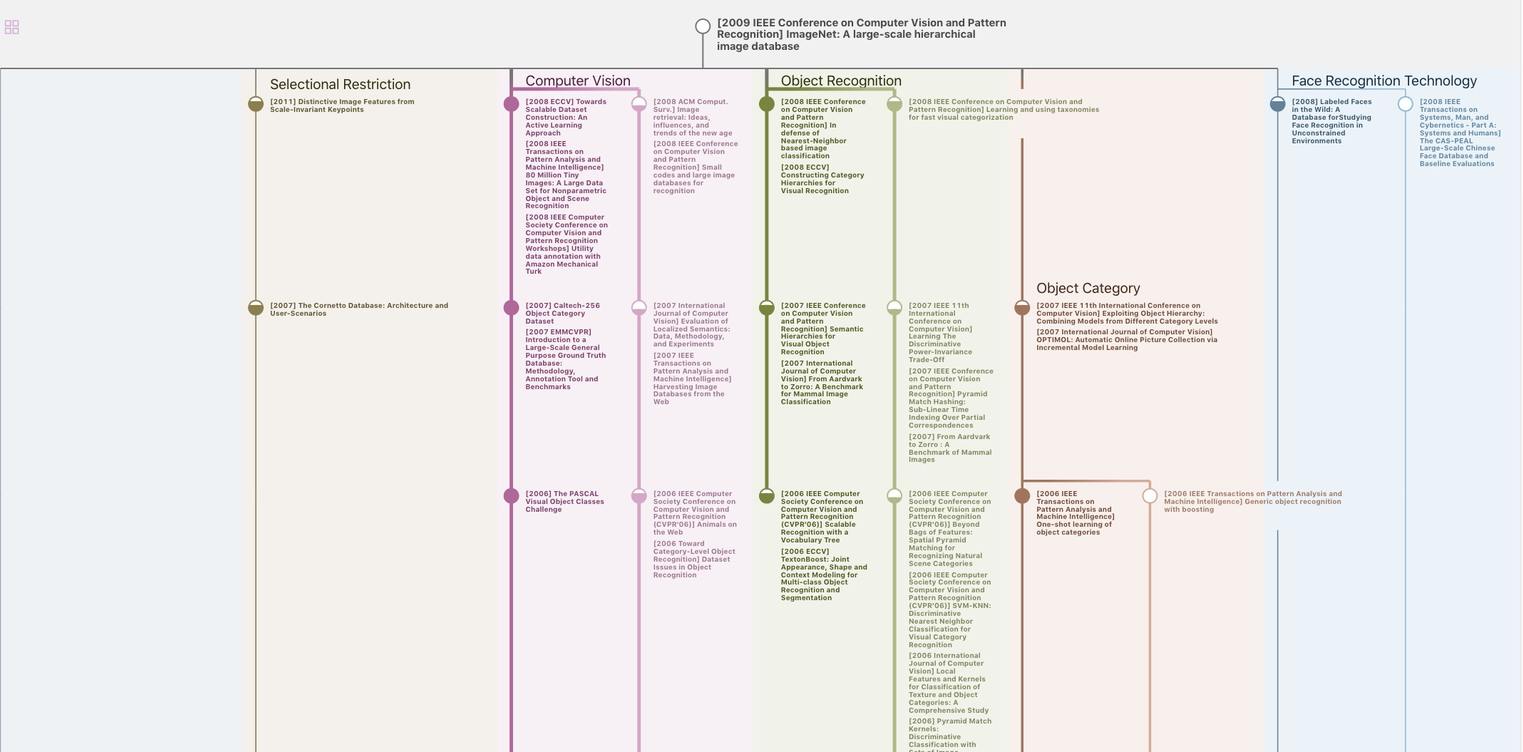
生成溯源树,研究论文发展脉络
Chat Paper
正在生成论文摘要