Particle Swarm Optimization based Optimization for Batch Processes
chinese automation congress(2019)
Abstract
Considering trajectory optimization and local extremum problems, a 2D batch process optimization method with initial state optimization is proposed in this paper. It integrates the information of time axis and batch axis unique to batch process. Particle swarm optimization (PSO), which is used to optimize the initial value so that it has good tracking performance and the ability to avoid falling into local optimum. At the same time, model identification and dynamic R parameter compensation are used to compensate the mismatch between the model and the controlled object, so that it has zero error tracking ability. By solving MPC optimization problem with time-varying predictive horizon, the control law is obtained, which has good tracking performance and robustness against disturbance and uncertainty. In addition, model identification and dynamic R parameters are used to compensate model mismatch, which makes zero error tracking possible. Secondly, the convergence analysis and tracking performance of the proposed integrated model predictive learning control system are strictly proved. Finally, the effectiveness of this method is verified by simulation.
MoreTranslated text
Key words
particle swarm optimization, model predictive control, batch process, dynamic R-parameter, model identification
AI Read Science
Must-Reading Tree
Example
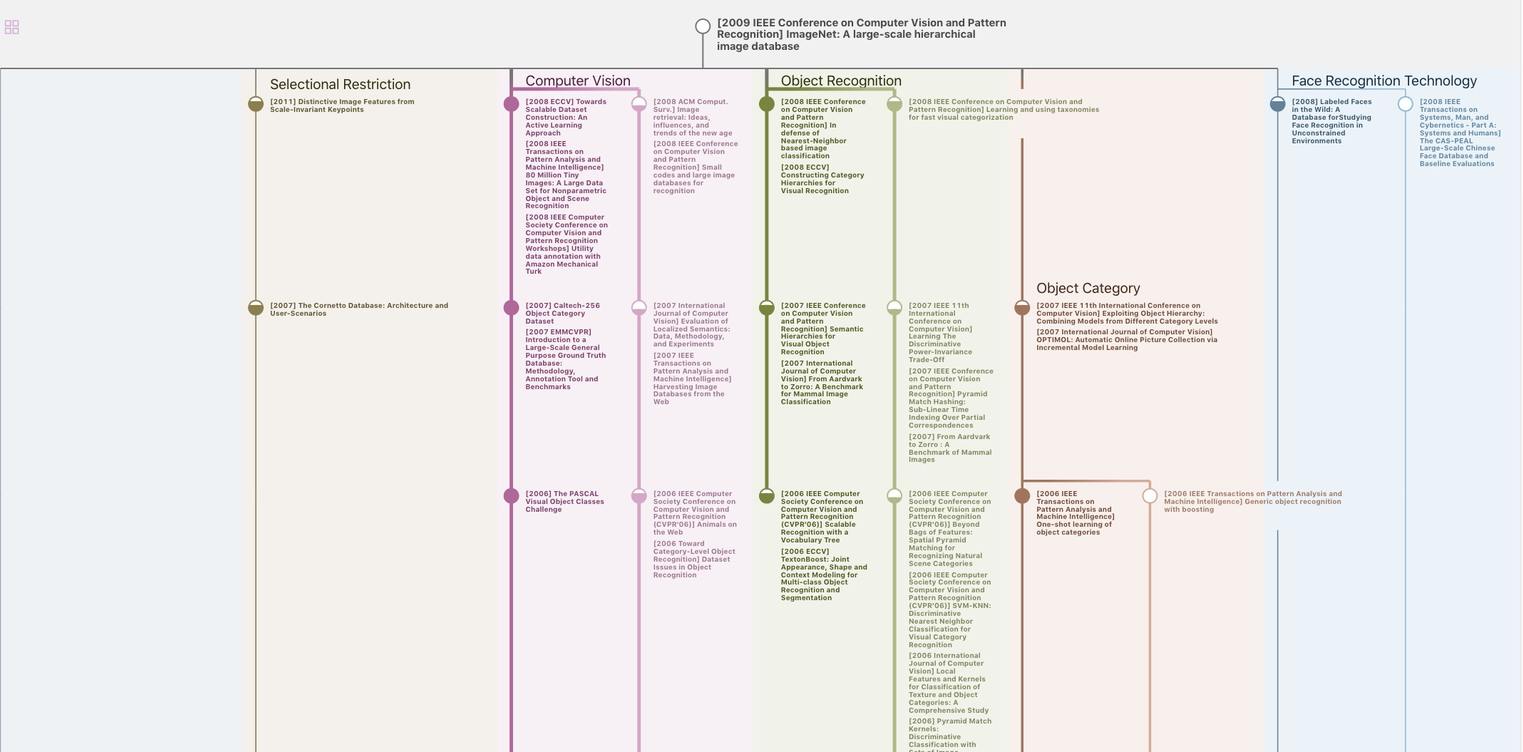
Generate MRT to find the research sequence of this paper
Chat Paper
Summary is being generated by the instructions you defined