Abstract P4-01-03: Computer vision and machine learning allows for the prediction of genomic instability using circulating tumor cell morphology in triple negative breast cancer patients
CANCER RESEARCH(2020)
摘要
Background: Tissue based tests of BRCA1/2 and other Homologous Recombination Gene (HRD) alterations associated with PARPi response are often limited by sample accessibility/availability, under-sampling due to tumor heterogeneity, and the lack of known clinical relevance for a significant fraction of DNA repair associated genomic alterations (e.g. VUS mutations). Assessment of HRD phenotypes at the DNA level in single Circulating Tumor Cells (CTCs) could in principle circumvent these issues. In past work, we developed a computer vision algorithm that accurately identifies CTCs with an HRD like phenotype, characterized by a high number of Large Scale Transitions (LSTs). This CTC image based biomarker (predicted LST or pLST) of genomic and/or chromosomal instability was found to associate strongly with androgen receptor targeted therapy resistance and also positively to PARPi response in metastatic prostate cancer. Here we sought to develop similar CTC based technology in Triple Negative Breast Cancer (TNBC). Methods: Training set: 521 CTCs from 26 metastatic prostate cancer patients. Test set: 179 CTCs from 24 TNBC patients. CTCs from both data sets were detected with the Epic Sciences platform and analyzed for 19 phenotypic digital pathology features, such as cytokeratin expression, nuclear texture, and cell size. The same CTCs were single-cell sequenced for the number of LSTs. LSTs are 10 Mb gains or losses in chromosomal structure and are a direct downstream measure of deficiencies in HR associated genes. A regression algorithm was generated and cross validated from the training set to predict the number of LSTs from CTC phenotypic features. The trained algorithm was then applied to the test set and the predicted number of LSTs and the actual number of LSTs determined by sequencing were compared to assess model or classifier performance. Results: The pLST algorithm is able to identify HRD like phenotypes defined as a single cell having a high number of LSTs at a sensitivity of 78%, specificity of 96% and accuracy of 87%. Conclusions: Despite being trained utilizing CTCs from prostate cancer, the pLST algorithm is able to accurately identify TNBC patients with a high number of LSTs in single CTCs consistent with an HRD like phenotype, suggesting possible sensitivity to PARPi or other therapies targeting genomic instability. Assessment of clinical associations in a TNBC cohort is ongoing. The ability to identify potential sensitivity to drugs targeting DNA repair using an immunfluoresence CTC staining method for CTC phenotype could help to stratify additional TNBC patients for both prognosis and for prediction of drug response. Citation Format: Adam Jendrisak, Jerry Lee, Angel Rodriguez, Ramsay Sutton, Joseph Schonhoft, Tiziano Pramparo, Rick Wenstrup, Yipeng Wang. Computer vision and machine learning allows for the prediction of genomic instability using circulating tumor cell morphology in triple negative breast cancer patients [abstract]. In: Proceedings of the 2019 San Antonio Breast Cancer Symposium; 2019 Dec 10-14; San Antonio, TX. Philadelphia (PA): AACR; Cancer Res 2020;80(4 Suppl):Abstract nr P4-01-03.
更多查看译文
AI 理解论文
溯源树
样例
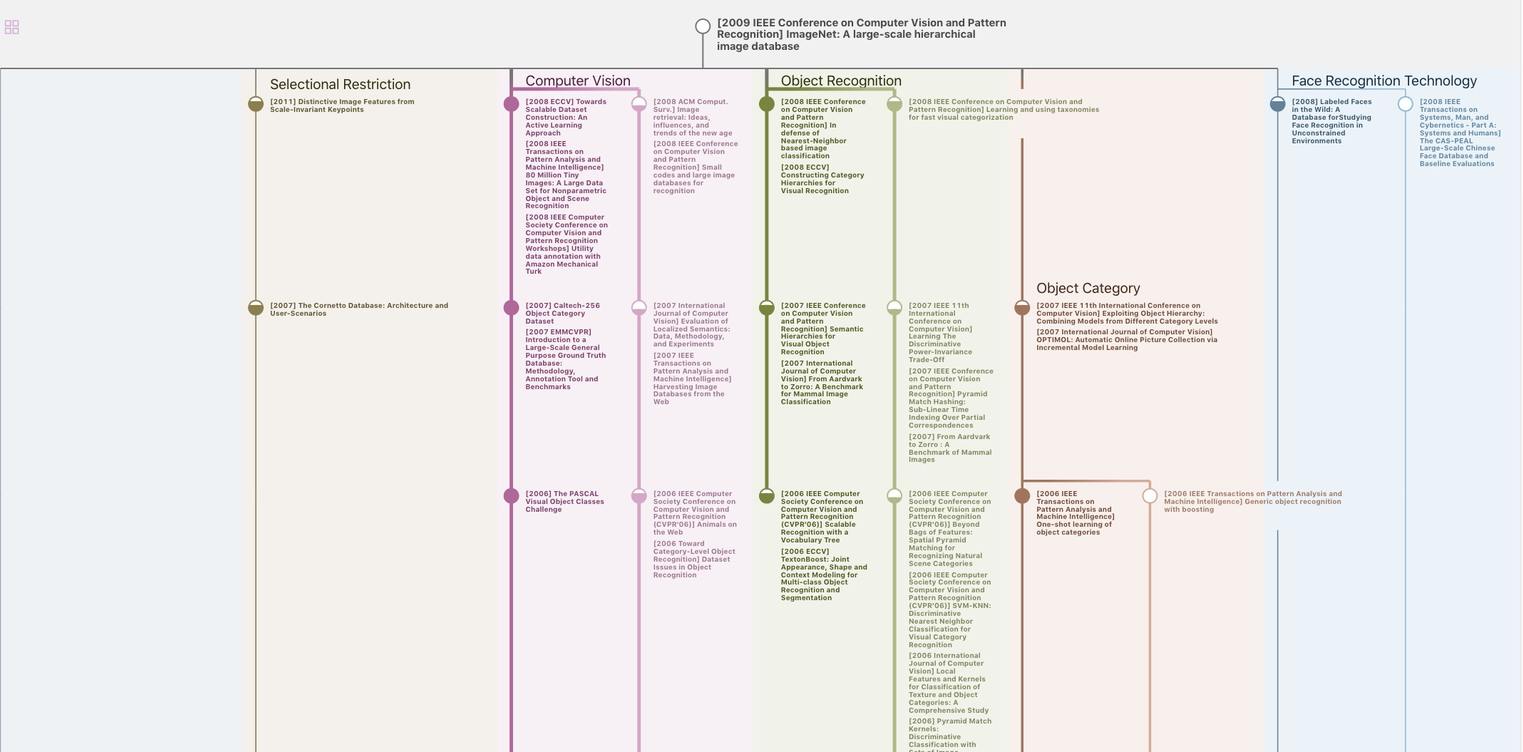
生成溯源树,研究论文发展脉络
Chat Paper
正在生成论文摘要