Learning with Resistive Switching Neural Networks
international electron devices meeting(2019)
摘要
With the slowdown of Moore’s law and the intensification of memory wall as well as von-Neumann bottleneck, processing-in-memory with emerging non-volatile analog devices, such as RRAMs or memristors, is a potential solution to accelerate machine learning in hardware neural networks, which may drastically improve the energy-area efficiency. In this paper, we discuss three major types of learning, namely the supervised, reinforcement, and unsupervised learning that are implemented with various 1-transistor-1-memristor (1T1R) based neural networks.
更多查看译文
关键词
unsupervised learning,resistive switching neural networks,Moore's law,memory wall,von-Neumann bottleneck,emerging nonvolatile analog devices,memristors,hardware neural networks,energy-area efficiency,1-transistor-1-memristor based neural networks,reinforcement
AI 理解论文
溯源树
样例
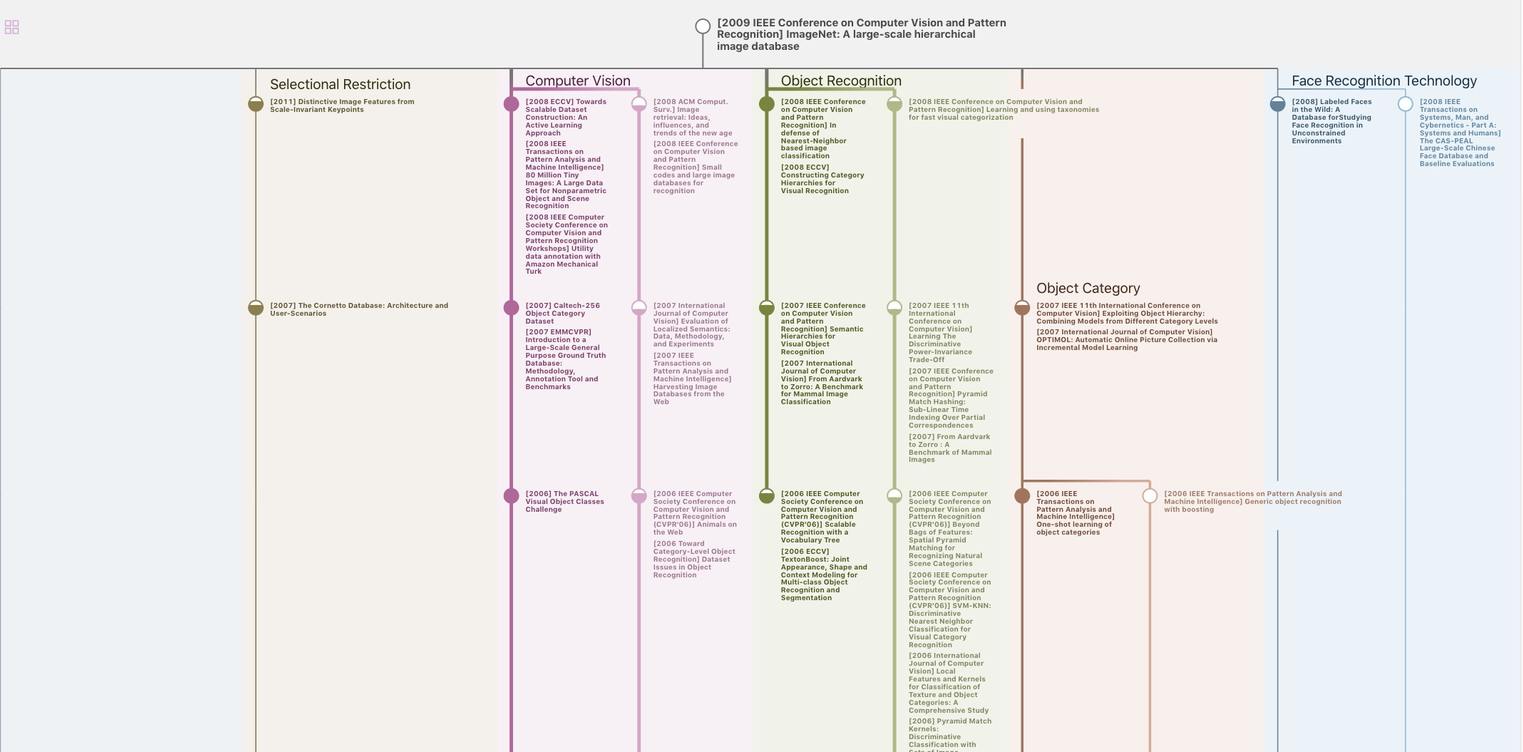
生成溯源树,研究论文发展脉络
Chat Paper
正在生成论文摘要