Digging into the multi-scale structure for a more refined depth map and 3D reconstruction
NEURAL COMPUTING & APPLICATIONS(2020)
摘要
Extracting dense depth from a single image is an important yet challenging computer vision task. Compared with stereo depth estimation, sensing the depth of a scene from monocular images is much more difficult and ambiguous because the epipolar geometry constraints cannot be exploited. The recent development of deep learning technologies has introduced significant progress in monocular depth estimation. This paper aims to explore the effects of multi-scale structures on the performance of monocular depth estimation and further obtain a more refined 3D reconstruction by using our predicted depth and corresponding uncertainty. First, we explore three multi-scale architectures and compare the qualitative and quantitative results of some state-of-the-art approaches. Second, in order to improve the robustness of the system and provide the reliability of the predicted depth for subsequent 3D reconstruction, we estimate the uncertainty of noisy data by modeling such uncertainty in a new loss function. Last, the predicted depth map and corresponding depth uncertainty are incorporated into a monocular reconstruction system. The experiments of monocular depth estimation are mainly performed on the widely used NYU V2 depth dataset, on which the proposed method achieves a state-of-the-art performance. For the 3D reconstruction, the implementation of our proposed framework can reconstruct more smooth and dense models on various scenes.
更多查看译文
关键词
Depth estimation,Multi-scale,3D reconstruction,Uncertainty estimation
AI 理解论文
溯源树
样例
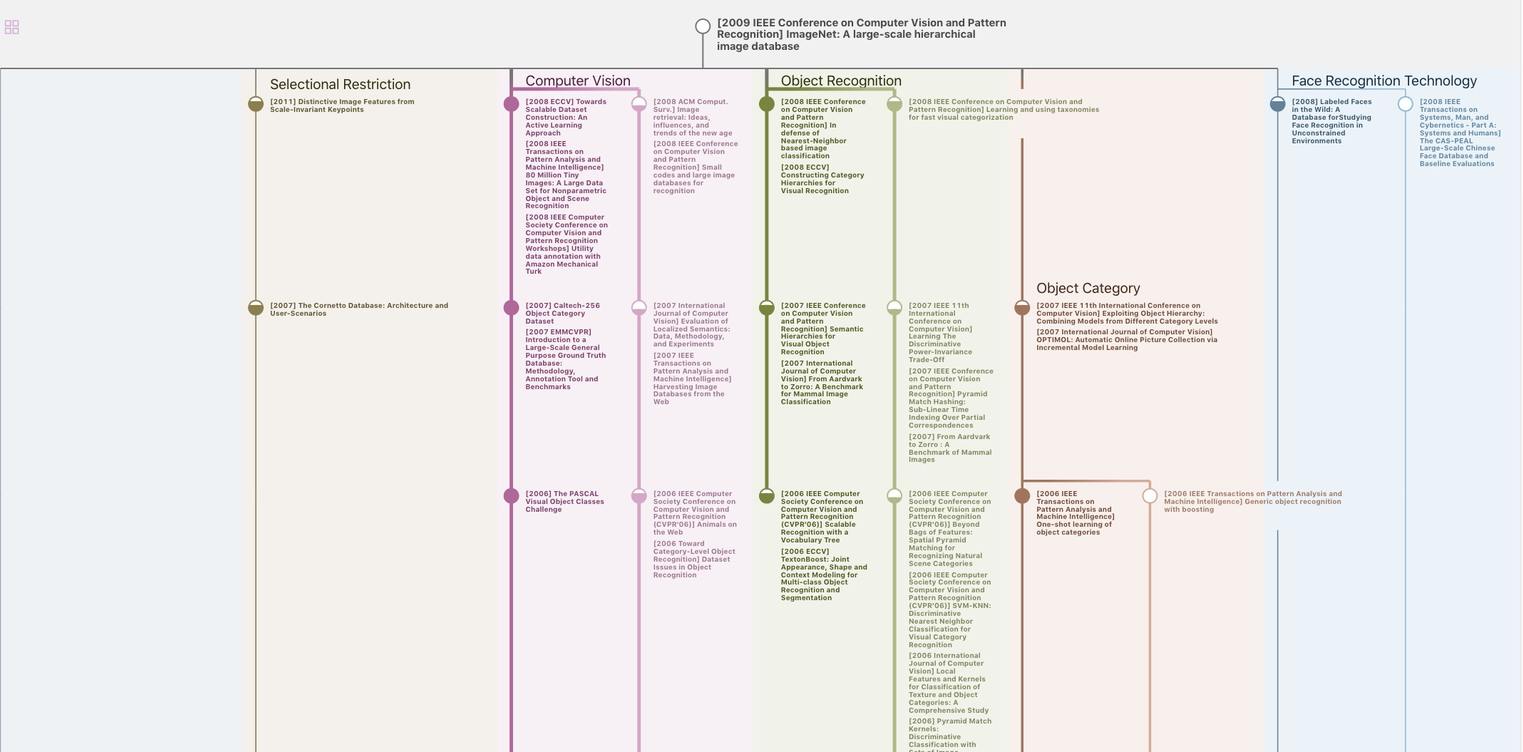
生成溯源树,研究论文发展脉络
Chat Paper
正在生成论文摘要