Pseudo Estimation and Variable Selection in Regression
Journal of statistical planning and inference(2020)
摘要
Often a problem in linear regression either for correlated data or high-dimensional data is the singularity of the sample covariance matrix. While dealing with an ill-conditioned covariance matrix has been a longstanding challenge in the statistical literature, recent proposals relying on adding noises to the original data have found their successes in obtaining reliable estimates and making predictions. It has been shown that perturbing data with noises has a close relationship to the well-known ridge estimator. In this paper, we propose to add noises to the predictors with a known covariance structure, and call the estimator obtained in such a way a “pseudo estimator”. A new variable selection procedure based on the concept of pseudo confidence interval which works for both correlated predictors and “large p small n” problem is proposed. We study the theoretical properties of the proposed pseudo estimator and variable selection procedure. Furthermore, we use an ensemble step to stabilize the pseudo estimation (variable selection) results. The advantages of the proposed method are demonstrated by both simulation studies and real data analyses.
更多查看译文
关键词
Correlated predictors,Dimension reduction,Ensemble,Large p small n,Measurement error regression,Ridge regression
AI 理解论文
溯源树
样例
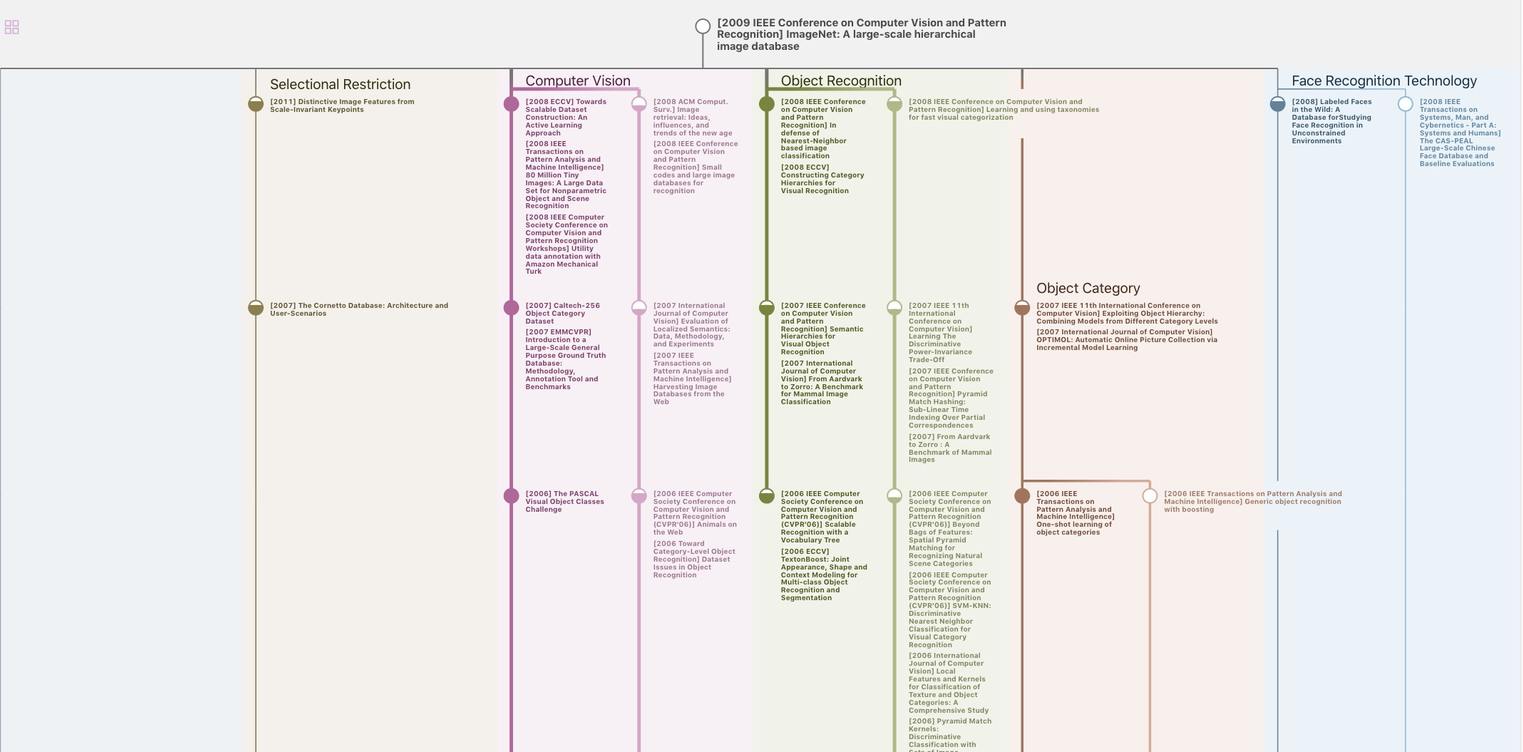
生成溯源树,研究论文发展脉络
Chat Paper
正在生成论文摘要