A Subsolid Nodules Imaging Reporting System (SSN-IRS) for Classifying 3 Subtypes of Pulmonary Adenocarcinoma
CLINICAL LUNG CANCER(2020)
摘要
It is essential to identify the subsolid nodules subtype preoperatively to select the optimal treatment algorithm. We developed and validated an imaging reporting system using a classification and regression tree model that based on computed tomography imaging characteristics (291 cases in training group, 146 cases in testing group). The model showed high sensitivity and accuracy of classification. Our model can help clinicians to make follow-up recommendations or decisions for surgery for clinical patients with a subsolid nodule. Objectives: To develop an imaging reporting system for the classification of 3 adenocarcinoma subtypes of computed tomography (CT)-detected subsolid pulmonary nodules (SSNs) in clinical patients. Methods: Between November 2011 and October 2017, 437 pathologically confirmed SSNs were retrospectively identified. SSNs were randomly divided 2:1 into a training group (291 cases) and a testing group (146 cases). CT-imaging characteristics were analyzed using multinomial univariable and multivariable logistic regression analysis to identify discriminating factors for the 3 adenocarcinoma subtypes (pre-invasive lesions, minimally invasive adenocarcinoma, and invasive adenocarcinoma). These factors were used to develop a classification and regression tree model. Finally, an SSN Imaging Reporting System (SSN-IRS) was constructed based on the optimized classification model. For validation, the classification performance was evaluated in the testing group. Results: Of the CT-derived characteristics of SSNs, qualitative density (nonsolid or part-solid), core (non-core or core), semantic features (pleural indentation, vacuole sign, vascular invasion), and diameter of solid component (>= 6 mm or >6 mm), were the most important factors for the SSN-IRS. The total sensitivity, specificity, and diagnostic accuracy of the SSN-IRS was 89.0% (95% confidence interval [CI], 84.8%-92.4%), 74.6% (95% CI, 70.8%-78.1%), and 79.4% (95% CI, 76.5%-82.0%) in the training group and 84.9% (95% CI, 78.1%-90.3%), 68.5% (95% CI, 62.8%-73.8%), and 74.0% (95% CI, 69.6%-78.0%) in the testing group, respectively. Conclusions: The SSN-IRS can classify 3 adenocarcinoma subtypes using CT-based characteristics of subsolid pulmonary nodules. This classification tool can help clinicians to make follow-up recommendations or decisions for surgery in clinical patients with SSNs. (C) 2020 The Author(s). Published by Elsevier Inc.
更多查看译文
关键词
Decision trees,Diagnosis,Lung,Solitary pulmonary nodule,X-ray computed tomography
AI 理解论文
溯源树
样例
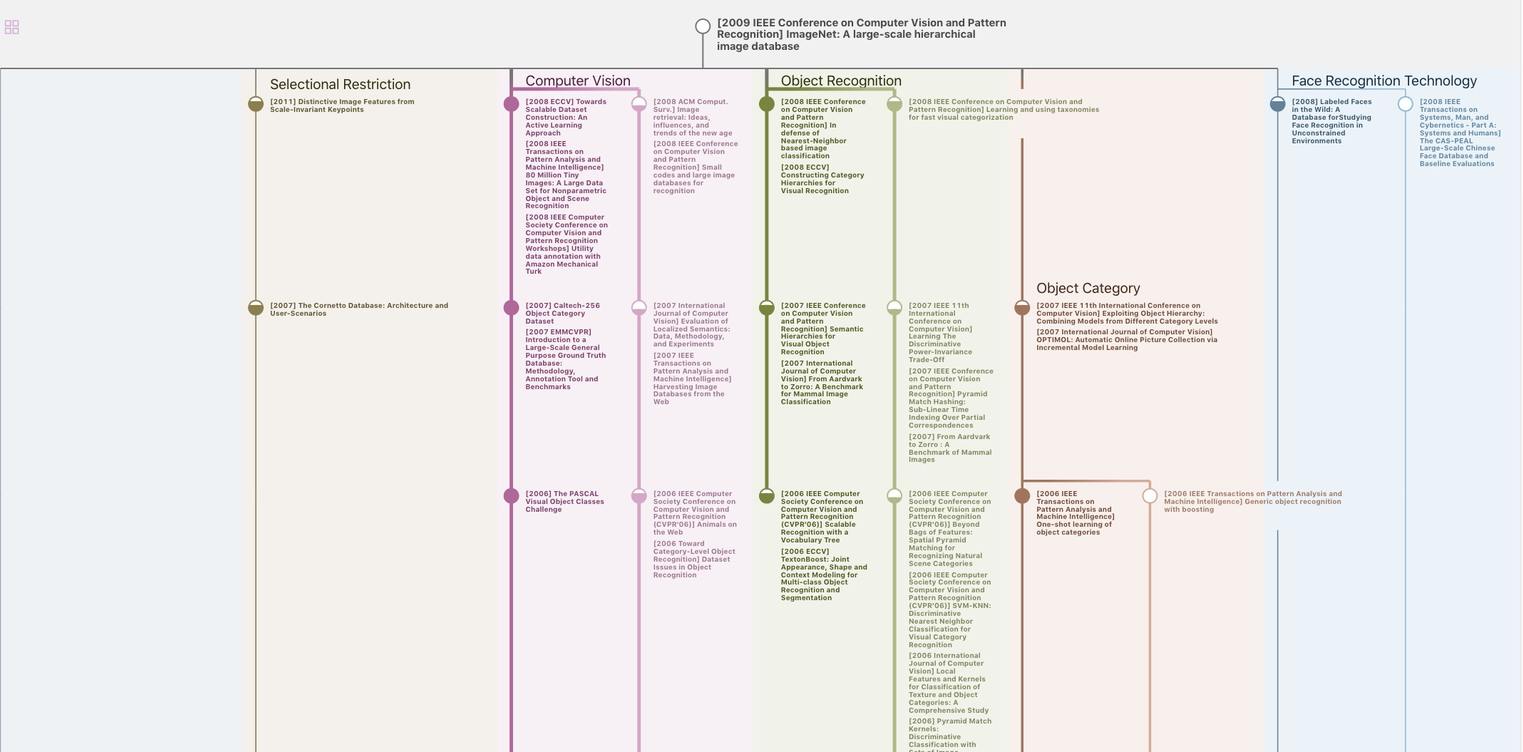
生成溯源树,研究论文发展脉络
Chat Paper
正在生成论文摘要