Nonlinear state-space modeling approaches to real-time autonomous geosteering
Journal of Petroleum Science and Engineering(2020)
摘要
We develop a nonlinear state-space modeling (SSM) framework for autonomous geosteering in real time, which aims to estimate the relative position between the wellbore and a target formation marker. Within a Bayesian framework, our approach formulates a coherent way of integrating information from various sources: (i) our prior understanding about the horizontal drilling and the target formation; (ii) the geometric interactions between the wellbore trajectory and the target formation layer; (iii) the log from a pilot reference well as a stratigraphic signature of the geological structure for a given region; and (iv) the observed log sequence collected along the wellbore by downhole sensors. We provide an extensive overview of the state-of-the-art inference algorithms for nonlinear SSM and apply those methods to the geosteering application. Using synthetic and real geosteering datasets, our proposed framework shows notable improvements over existing alternatives with higher correlation scores between the interpreted and the reference logs. To test the reliability of our framework, we further apply the framework on a benchmark set of 400 horizontal wells randomly sampled from the Permian Basin. We demonstrate that our algorithms obtain consistent performance that matches or exceeds competing methods and the human interpretation. Our framework provides geosteering geologists with accurate interpretations and appropriate uncertainty quantification, and helps them navigate the drilling bit into the target formation with confidence.
更多查看译文
关键词
Bayesian methods,Nonlinear state-space model,Particle filter,Autonomous geosteering
AI 理解论文
溯源树
样例
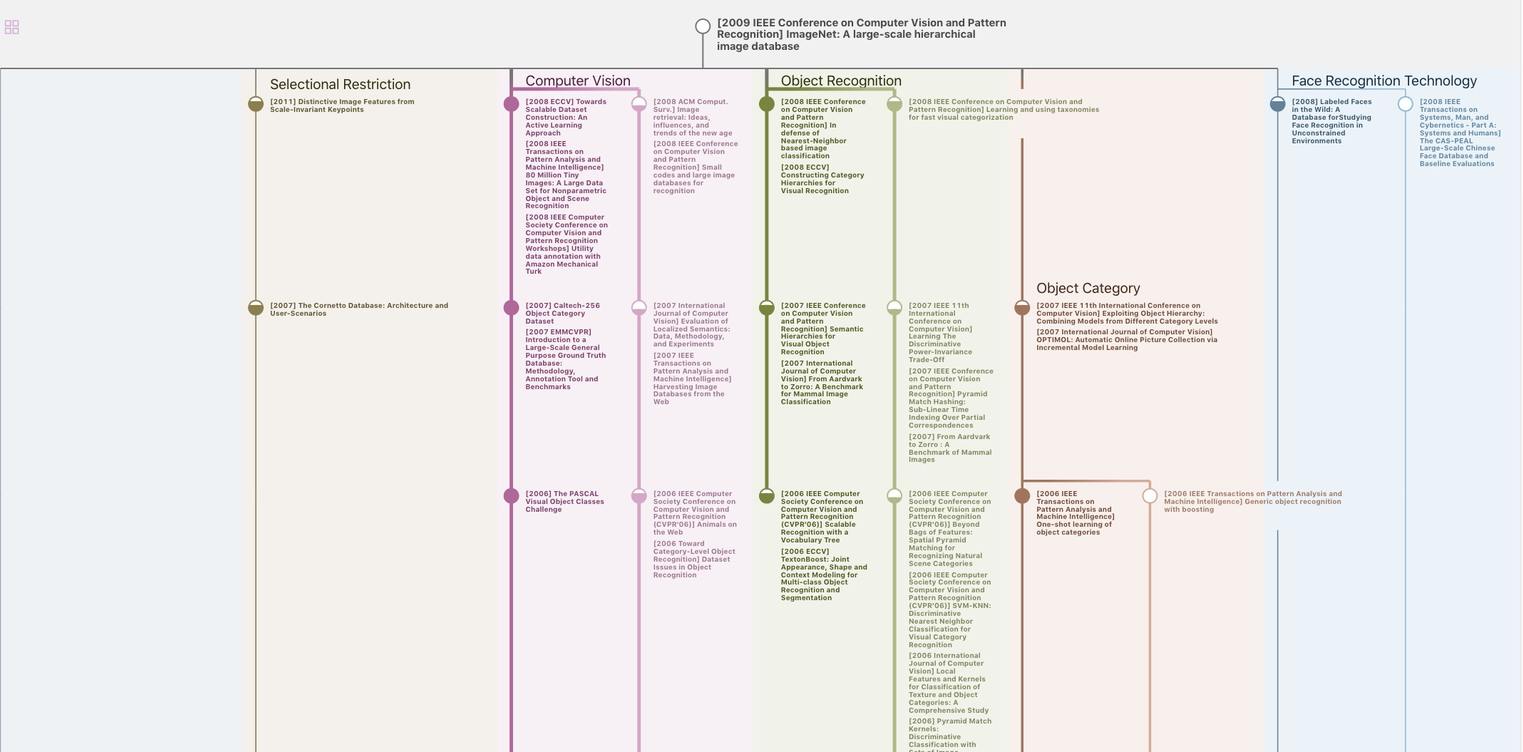
生成溯源树,研究论文发展脉络
Chat Paper
正在生成论文摘要