Deflectometric Data Segmentation For Surface Inspection: A Fully Convolutional Neural Network Approach
JOURNAL OF ELECTRONIC IMAGING(2020)
摘要
The purpose of this paper is to explore the use of fully convolutional neural networks (FCN) to perform a semantic segmentation of deflectometric recordings for quality control of reflective surfaces. The proposed method relies on a U-net network to identify the location and boundaries of the object and the possible defective areas present on it by performing a pixel-wise classification based on local curvatures and data modulation. Experiments were performed on a real industrial problem using four variations of the architecture. The results demonstrate that the method combining geometric and photometric information enables the identification of a wider variety of shape and texture imperfections, with the resulting segmentations closely correlated with the visual impact of the defects. In addition, several suggestions are presented for near-term industrial utilization. (C) 2020 SPIE and IS&T
更多查看译文
关键词
surface inspection, specular surfaces, defect detection, deflectometry, fully convolutional neural networks, segmentation
AI 理解论文
溯源树
样例
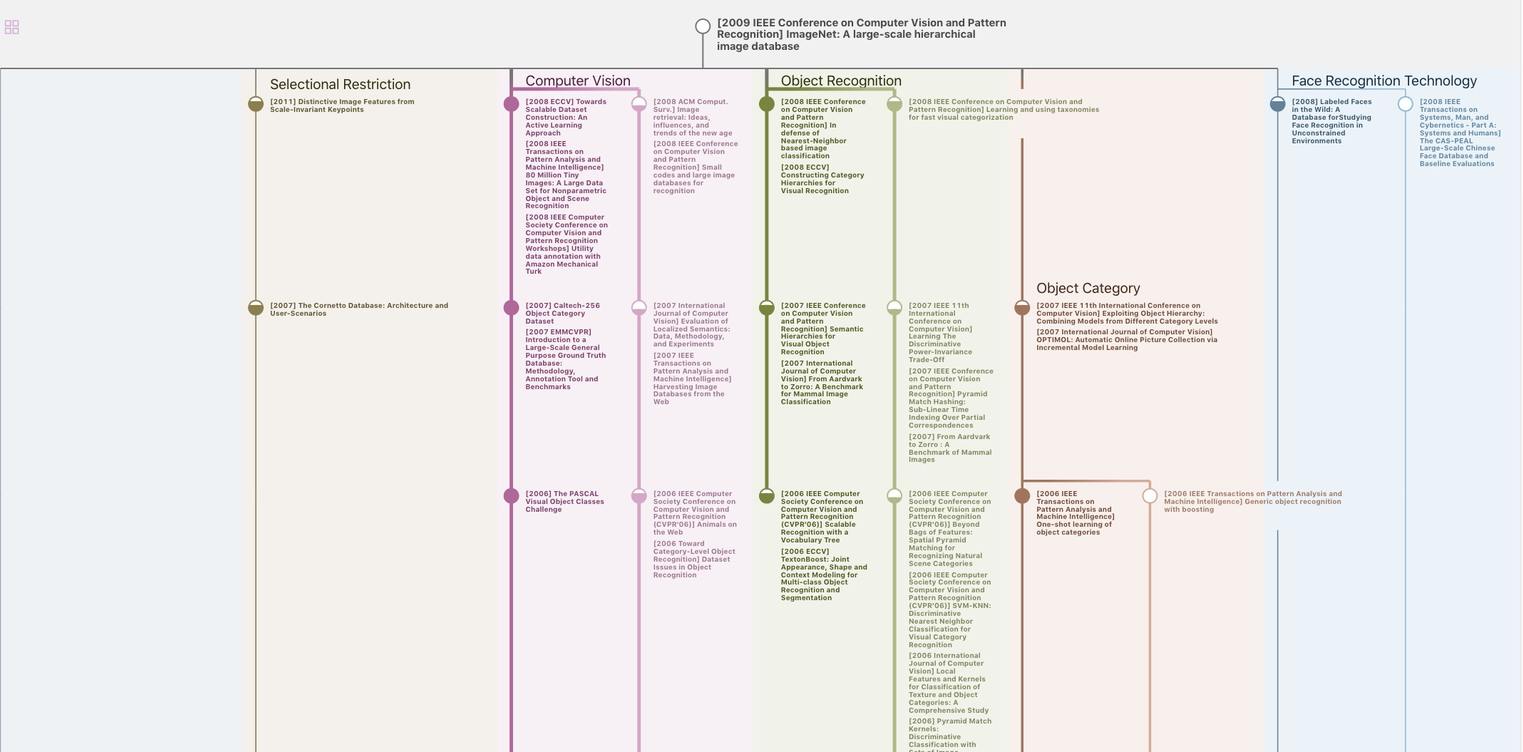
生成溯源树,研究论文发展脉络
Chat Paper
正在生成论文摘要