Hierarchical Models: Intrinsic Separability in High Dimensions
arxiv(2020)
摘要
It has long been noticed that high dimension data exhibits strange patterns. This has been variously interpreted as either a "blessing" or a "curse", causing uncomfortable inconsistencies in the literature. We propose that these patterns arise from an intrinsically hierarchical generative process. Modeling the process creates a web of constraints that reconcile many different theories and results. The model also implies high dimensional data posses an innate separability that can be exploited for machine learning. We demonstrate how this permits the open-set learning problem to be defined mathematically, leading to qualitative and quantitative improvements in performance.
更多查看译文
关键词
intrinsic separability,hierarchical models,high dimensions
AI 理解论文
溯源树
样例
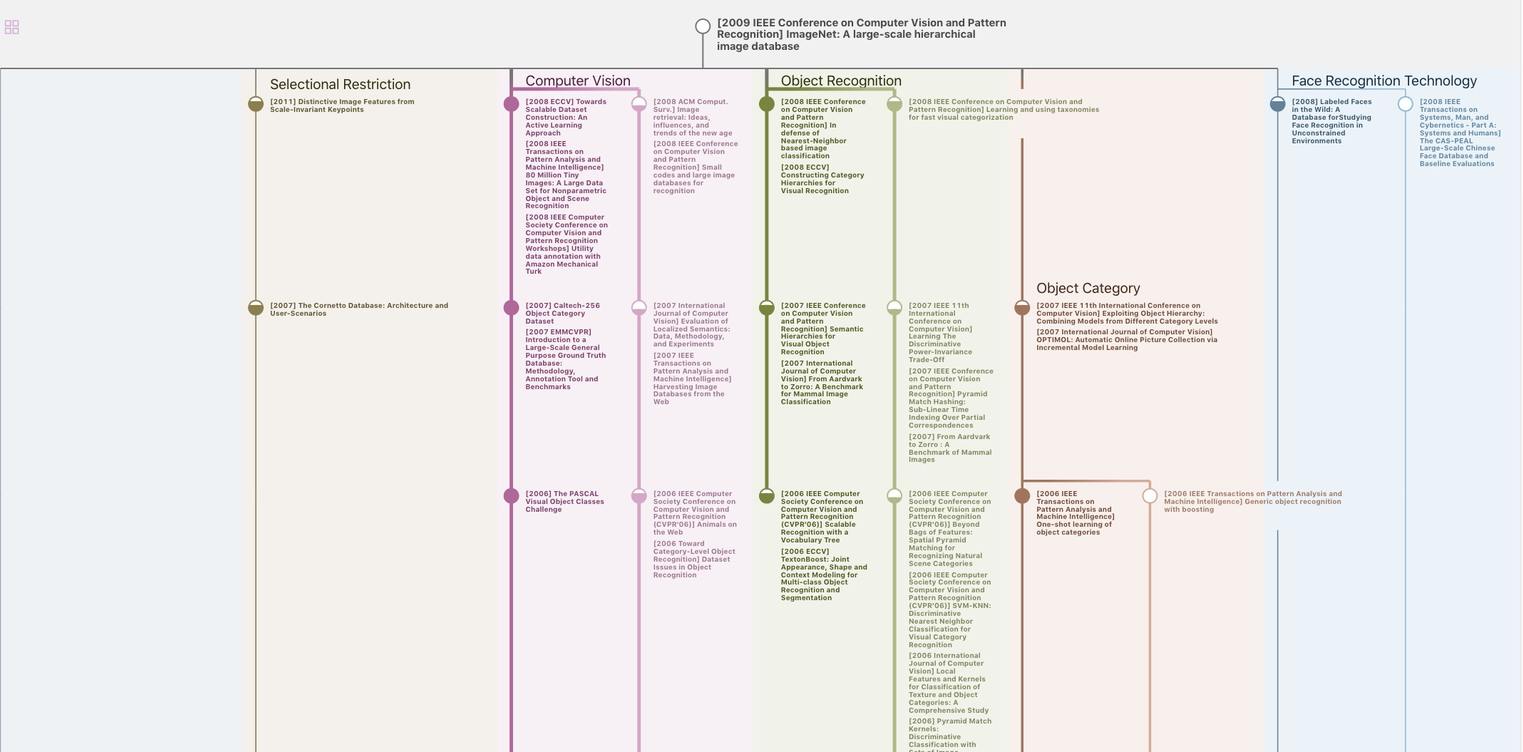
生成溯源树,研究论文发展脉络
Chat Paper
正在生成论文摘要