A Benchmarking Study of Embedding-based Entity Alignment for Knowledge Graphs
PROCEEDINGS OF THE VLDB ENDOWMENT(2020)
Abstract
Entity alignment seeks to find entities in different knowledge graphs (KGs) that refer to the same real-world object. Recent advancement in KG embedding impels the advent of embedding-based entity alignment, which encodes entities in a continuous embedding space and measures entity similarities based on the learned embeddings. In this paper, we conduct a comprehensive experimental study of this emerging field. We survey 23 recent embedding-based entity alignment approaches and categorize them based on their techniques and characteristics. We also propose a new KG sampling algorithm, with which we generate a set of dedicated benchmark datasets with various heterogeneity and distributions for a realistic evaluation. We develop an open-source library including 12 representative embedding-based entity alignment approaches, and extensively evaluate these approaches, to understand their strengths and limitations. Additionally, for several directions that have not been explored in current approaches, we perform exploratory experiments and report our preliminary findings for future studies. The benchmark datasets, open-source library and experimental results are all accessible online and will be duly maintained.
MoreTranslated text
Key words
Knowledge Graph Embedding,Network Embedding,Named Entity Recognition,Signal Processing on Graphs,Data Integration
AI Read Science
Must-Reading Tree
Example
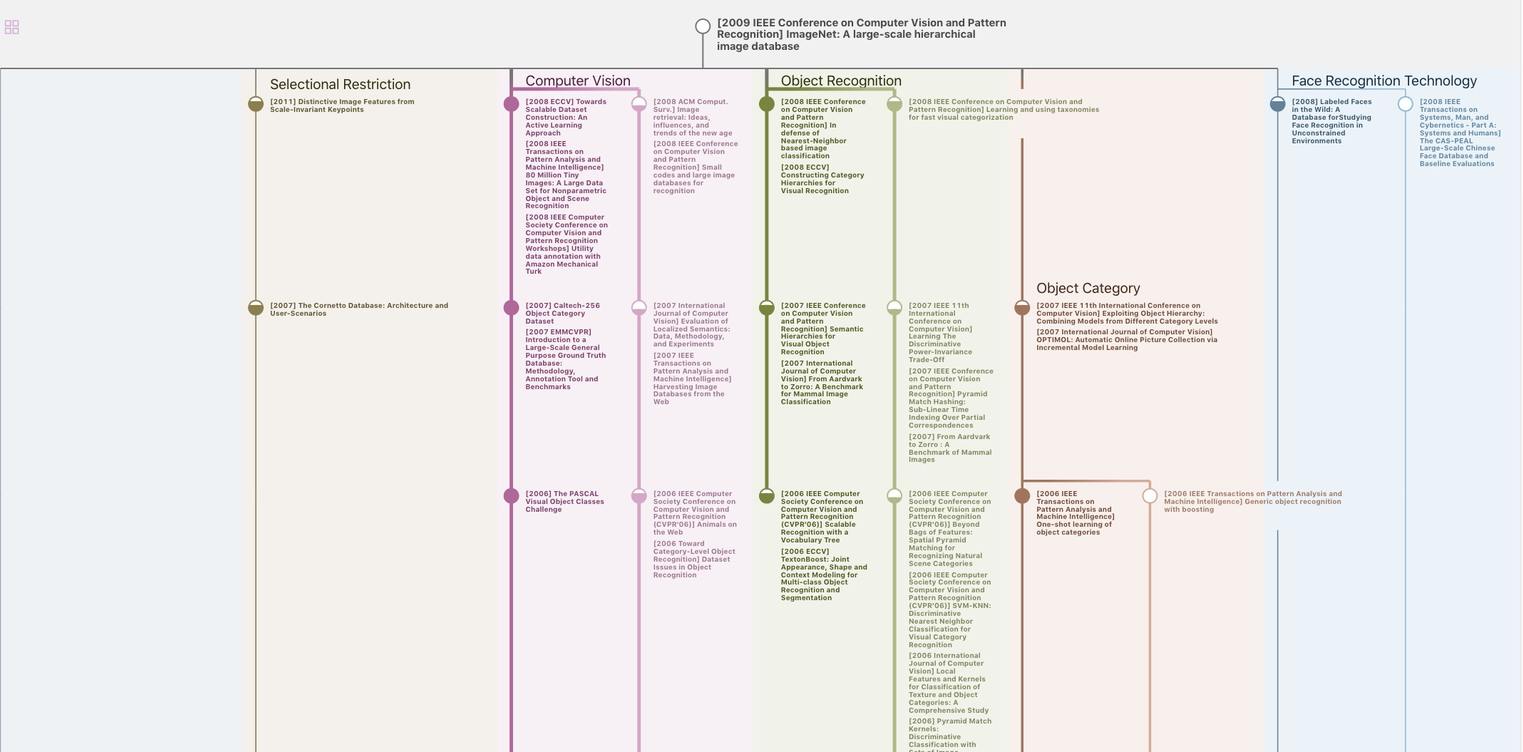
Generate MRT to find the research sequence of this paper
Chat Paper
Summary is being generated by the instructions you defined