Data-driven surrogate modelling and benchmarking for process equipment
Data-Centric Engineering(2020)
摘要
A suite of computational fluid dynamics (CFD) simulations geared towards chemical process equipment modelling has been developed and validated with experimental results from the literature. Various regression based active learning strategies are explored with these CFD simulators in-the-loop under the constraints of a limited function evaluation budget. Specifically, five different sampling strategies and five regression techniques are compared, considering a set of three test cases of industrial significance and varying complexity. Gaussian process regression was observed to have a consistently good performance for these applications. The present quantitative study outlines the pros and cons of the different available techniques and highlights the best practices for their adoption. The test cases and tools are available with an open-source license, to ensure reproducibility and engage the wider research community in contributing to both the CFD models and developing and benchmarking new improved algorithms tailored to this field.
更多查看译文
关键词
Computational fluid dynamics, data-driven, machine learning, surrogate models
AI 理解论文
溯源树
样例
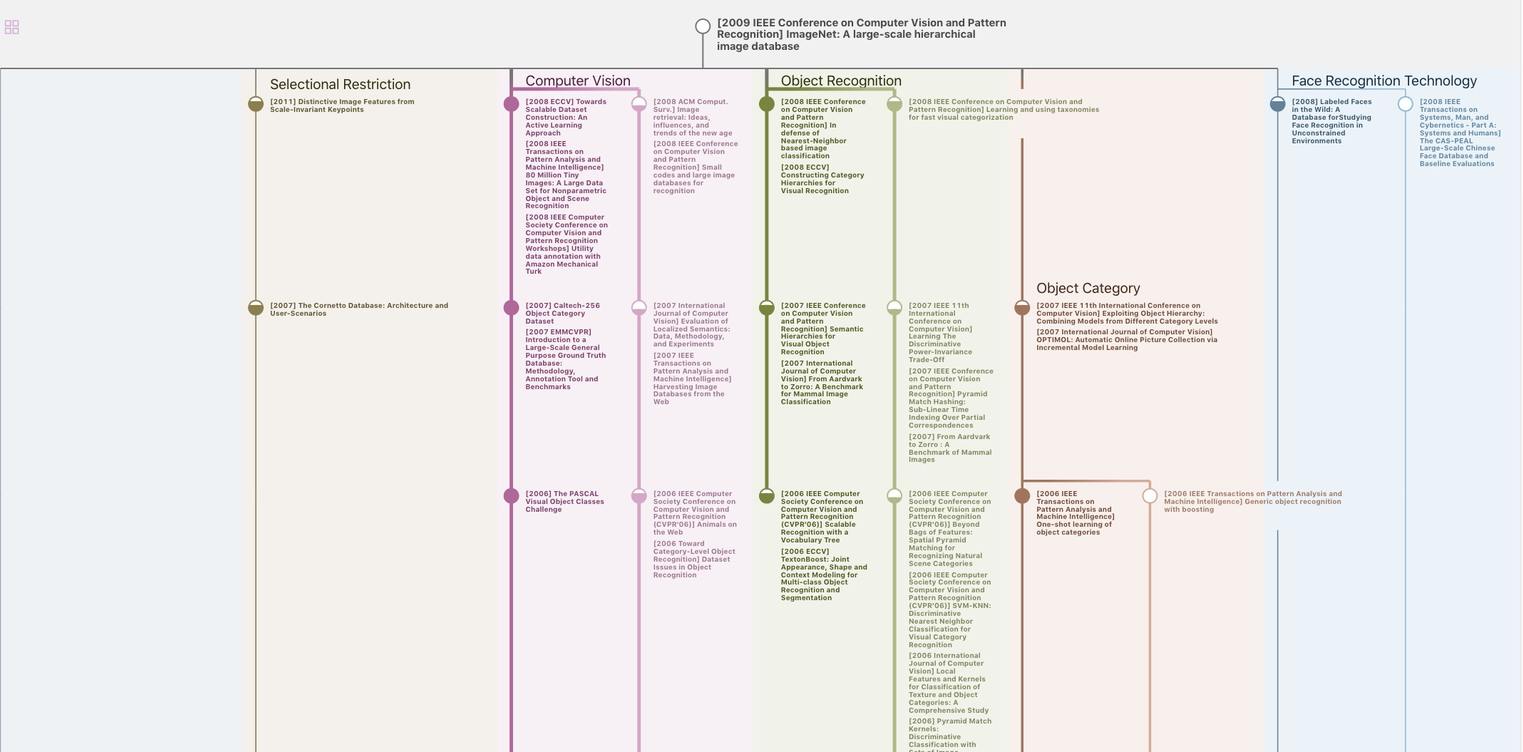
生成溯源树,研究论文发展脉络
Chat Paper
正在生成论文摘要