A comprehensive study on the prediction reliability of graph neural networks for virtual screening
arXiv (Cornell University)(2020)
摘要
Prediction models based on deep neural networks are increasingly gaining attention for fast and accurate virtual screening systems. For decision makings in virtual screening, researchers find it useful to interpret an output of classification system as probability, since such interpretation allows them to filter out more desirable compounds. However, probabilistic interpretation cannot be correct for models that hold over-parameterization problems or inappropriate regularizations, leading to unreliable prediction and decision making. In this regard, we concern the reliability of neural prediction models on molecular properties, especially when models are trained with sparse data points and imbalanced distributions. This work aims to propose guidelines for training reliable models, we thus provide methodological details and ablation studies on the following train principles. We investigate the effects of model architectures, regularization methods, and loss functions on the prediction performance and reliability of classification results. Moreover, we evaluate prediction reliability of models on virtual screening scenario. Our result highlights that correct choice of regularization and inference methods is evidently important to achieve high success rate, especially in data imbalanced situation. All experiments were performed under a single unified model implementation to alleviate external randomness in model training and to enable precise comparison of results.
更多查看译文
关键词
virtual screening,prediction reliability,graph,networks
AI 理解论文
溯源树
样例
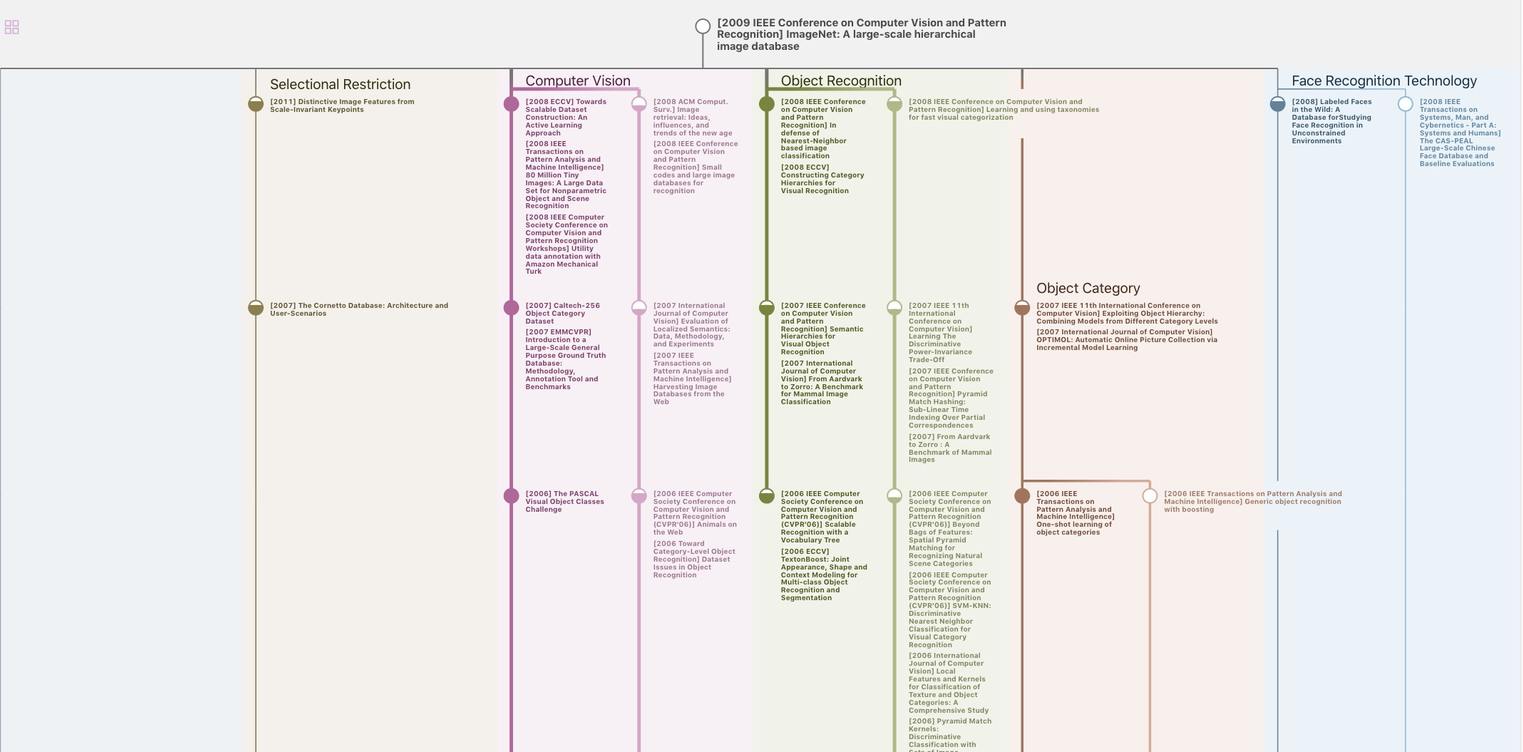
生成溯源树,研究论文发展脉络
Chat Paper
正在生成论文摘要