"An Image is Worth a Thousand Features": Scalable Product Representations for In-Session Type-Ahead Personalization
WWW '20: The Web Conference 2020 Taipei Taiwan April, 2020(2020)
摘要
We address the problem of personalizing query completion in a digital commerce setting, in which the bounce rate is typically high and recurring users are rare. We focus on in-session personalization and improve a standard noisy channel model by injecting dense vectors computed from product images at query time. We argue that image-based personalization displays several advantages over alternative proposals (from data availability to business scalability), and provide quantitative evidence and qualitative support on the effectiveness of the proposed methods. Finally, we show how a shared vector space between similar shops can be used to improve the experience of users browsing across sites, opening up the possibility of applying zero-shot unsupervised personalization to increase conversions. This will prove to be particularly relevant to retail groups that manage multiple brands and/or websites and to multi-tenant SaaS providers that serve multiple clients in the same space.
更多查看译文
关键词
e-commerce query auto-completion, conditional language model, neural networks, zero-shot learning, transfer learning
AI 理解论文
溯源树
样例
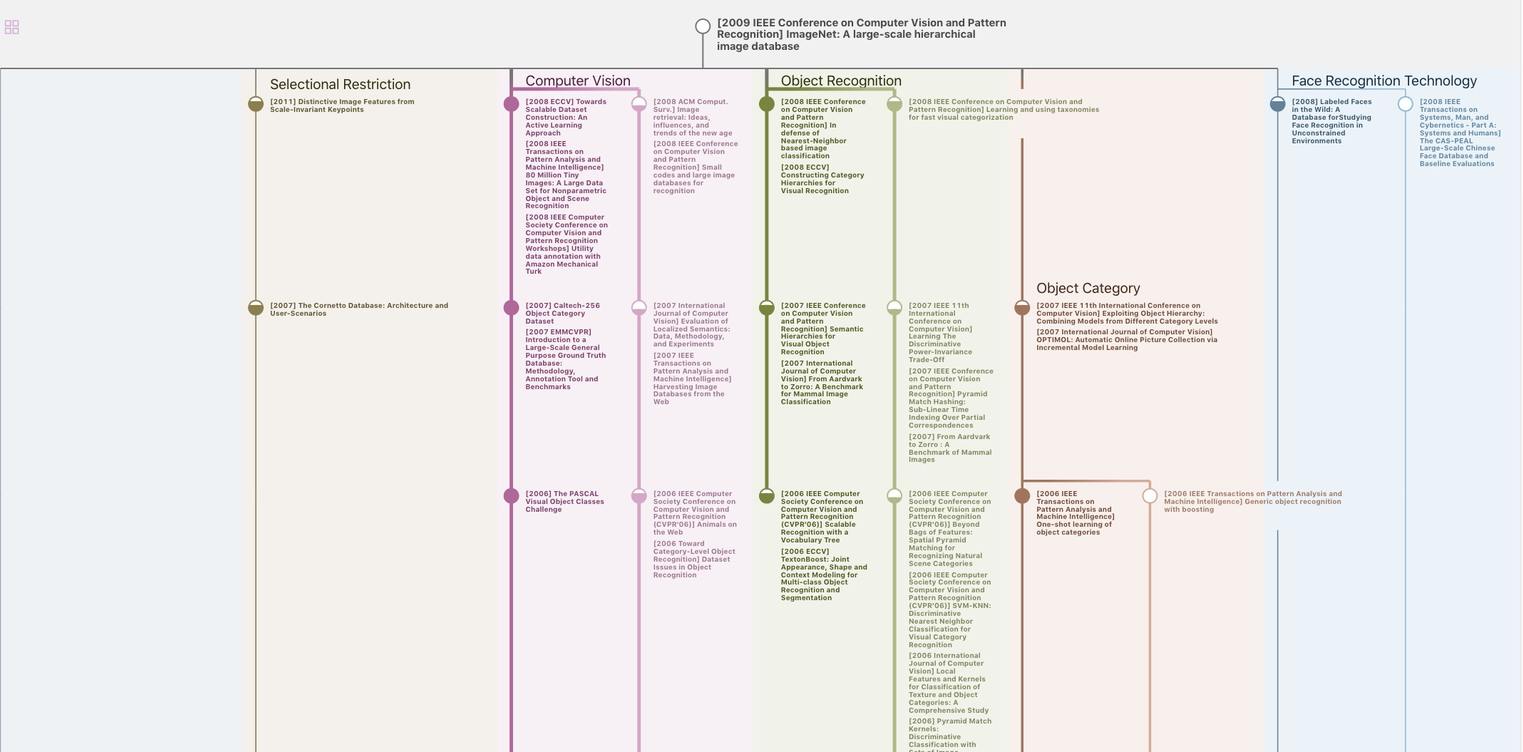
生成溯源树,研究论文发展脉络
Chat Paper
正在生成论文摘要