Anomalous Instance Detection in Deep Learning: A Survey
arXiv (Cornell University)(2020)
Abstract
Deep Learning (DL) is vulnerable to out-of-distribution and adversarial examples resulting in incorrect outputs. To make DL more robust, several posthoc anomaly detection techniques to detect (and discard) these anomalous samples have been proposed in the recent past. This survey tries to provide a structured and comprehensive overview of the research on anomaly detection for DL based applications. We provide a taxonomy for existing techniques based on their underlying assumptions and adopted approaches. We discuss various techniques in each of the categories and provide the relative strengths and weaknesses of the approaches. Our goal in this survey is to provide an easier yet better understanding of the techniques belonging to different categories in which research has been done on this topic. Finally, we highlight the unsolved research challenges while applying anomaly detection techniques in DL systems and present some high-impact future research directions.
MoreTranslated text
Key words
instance,detection,deep learning
AI Read Science
Must-Reading Tree
Example
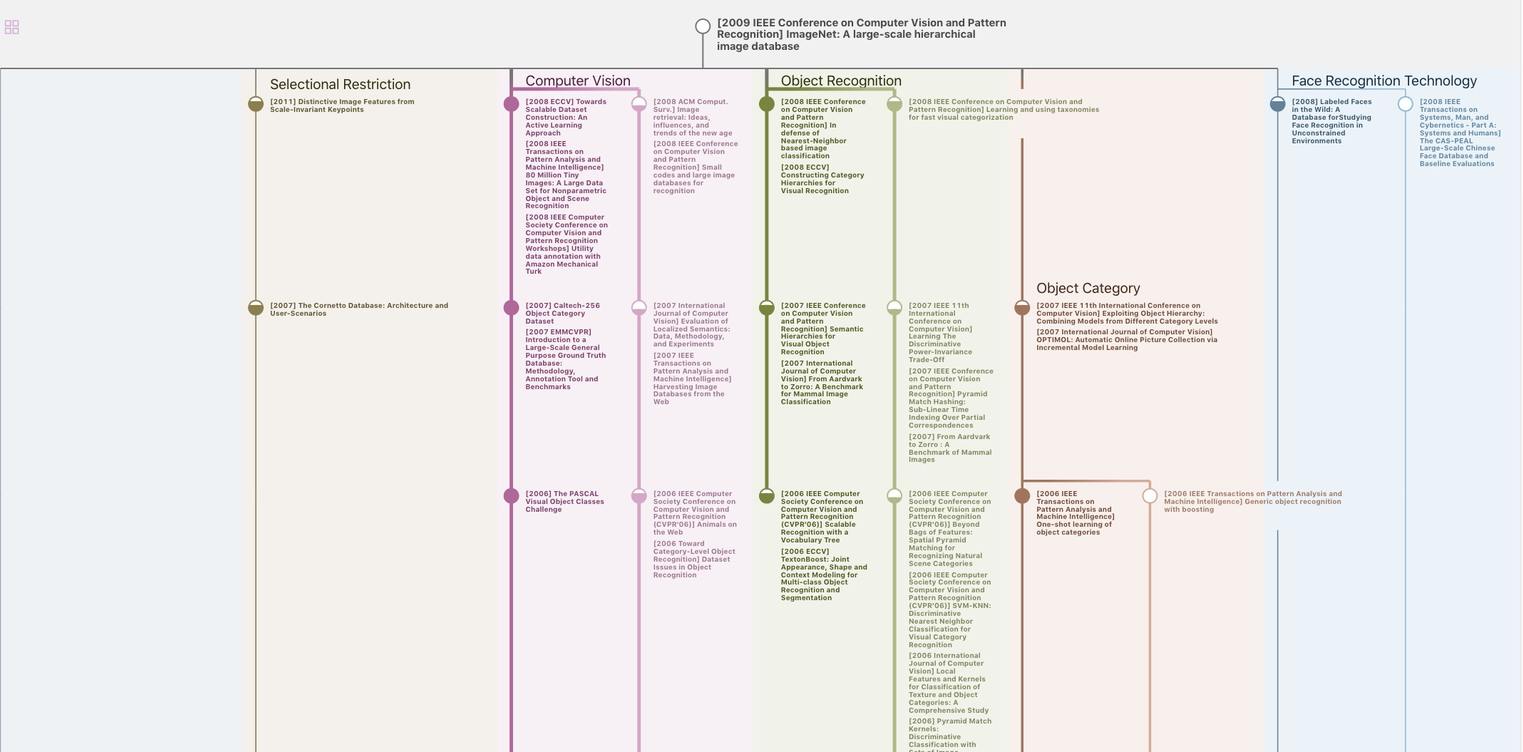
Generate MRT to find the research sequence of this paper
Chat Paper
Summary is being generated by the instructions you defined