Secure Method For De-Identifying And Anonymizing Large Panel Datasets
2019 22ND INTERNATIONAL CONFERENCE ON INFORMATION FUSION (FUSION 2019)(2019)
摘要
Government agencies, as well as private companies, may need to share private information with third party organizations for various reasons. There exist legitimate concerns about disclosing the information of individuals, sensitive details of agencies and organizations, and other private information. Consequently, information shared with external parties may be redacted to hide confidential information about individuals and companies while providing essential data required by third parties in order to perform their duties. This paper presents a method to de-identify and anonymize large-scale panel data from an organization. The method can handle a variety of data types, and it is scalable to datasets of any size. The challenge of de-identification and anonymization a large-scale and diverse dataset is to protect individual identities and retain useful data in the presence of unstructured field data and unpredictable frequency distributions. This is addressed by analyzing the dataset and applying a filtering and aggregation method This is accompanied by a streamlined implementation and post-validation process, which ensures the security of the organization's data, and the computational efficiency of the approach when handling large-scale panel data sets.
更多查看译文
关键词
Data de-identification, Data Anonymization, Large-scale Panel Data, Inference Enterprise
AI 理解论文
溯源树
样例
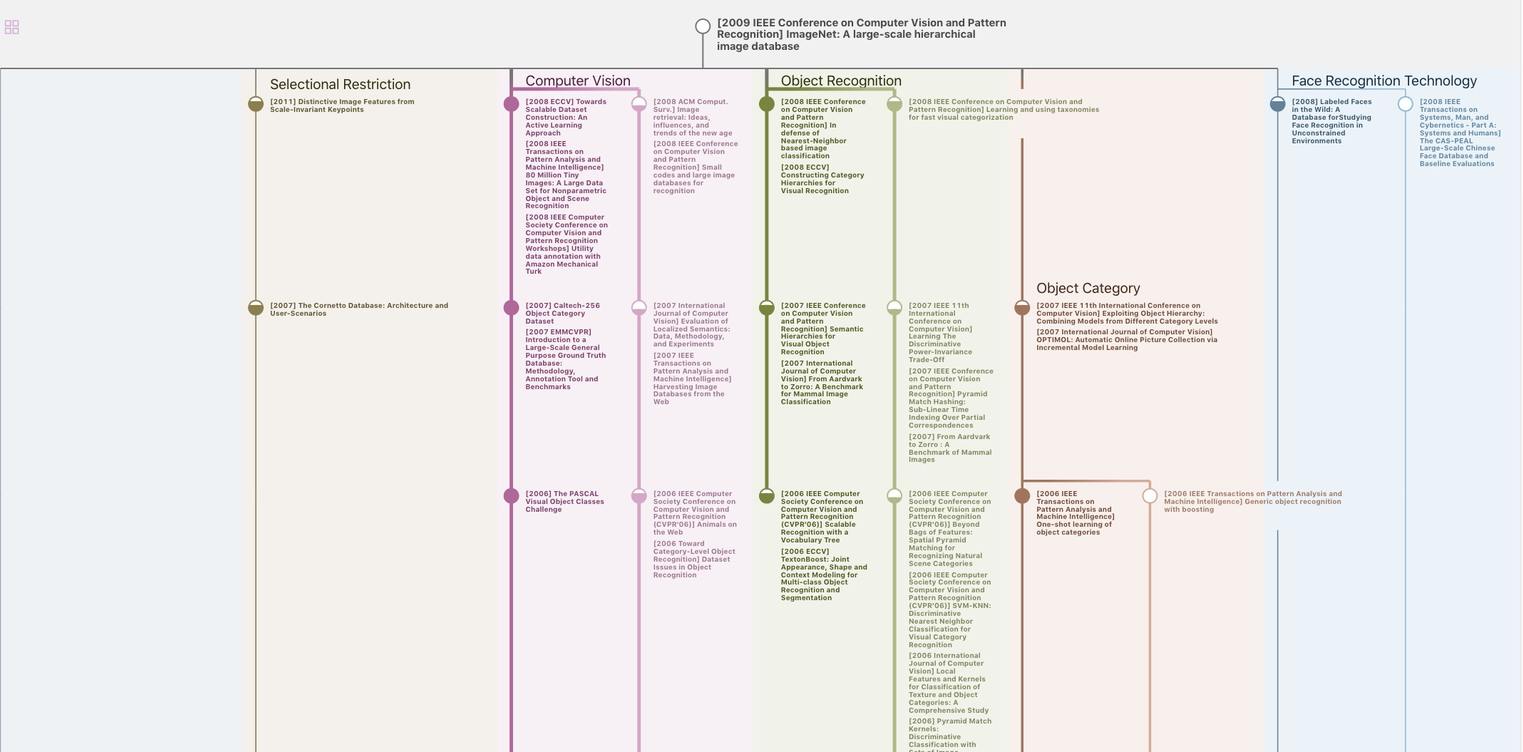
生成溯源树,研究论文发展脉络
Chat Paper
正在生成论文摘要