Bringing in the outliers: A sparse subspace clustering approach to learn a dictionary of mouse ultrasonic vocalizations
2020 IEEE INTERNATIONAL CONFERENCE ON ACOUSTICS, SPEECH, AND SIGNAL PROCESSING(2020)
摘要
Mice vocalize in the ultrasonic range during social interactions. These vocalizations are used in neuroscience and clinical studies to tap into complex behaviors and states. The analysis of these ultrasonic vocalizations (USVs) has been traditionally a manual process, which is prone to errors and human bias, and is not scalable to large scale analysis. We propose a new method to automatically create a dictionary of USVs based on a two-step spectral clustering approach, where we split the set of USVs into inlier and outlier data sets. This approach is motivated by the known degrading performance of sparse subspace clustering with outliers. We apply spectral clustering to the inlier data set and later find the clusters for the outliers. We propose quantitative and qualitative performance measures to evaluate our method in this setting, where there is no ground truth. Our approach outperforms two baselines based on k-means and spectral clustering in all of the proposed performance measures, showing greater distances between clusters and more variability between clusters.
更多查看译文
关键词
sparse subspace clustering, subspace similarity, clustering, mouse ultrasonic vocalizations
AI 理解论文
溯源树
样例
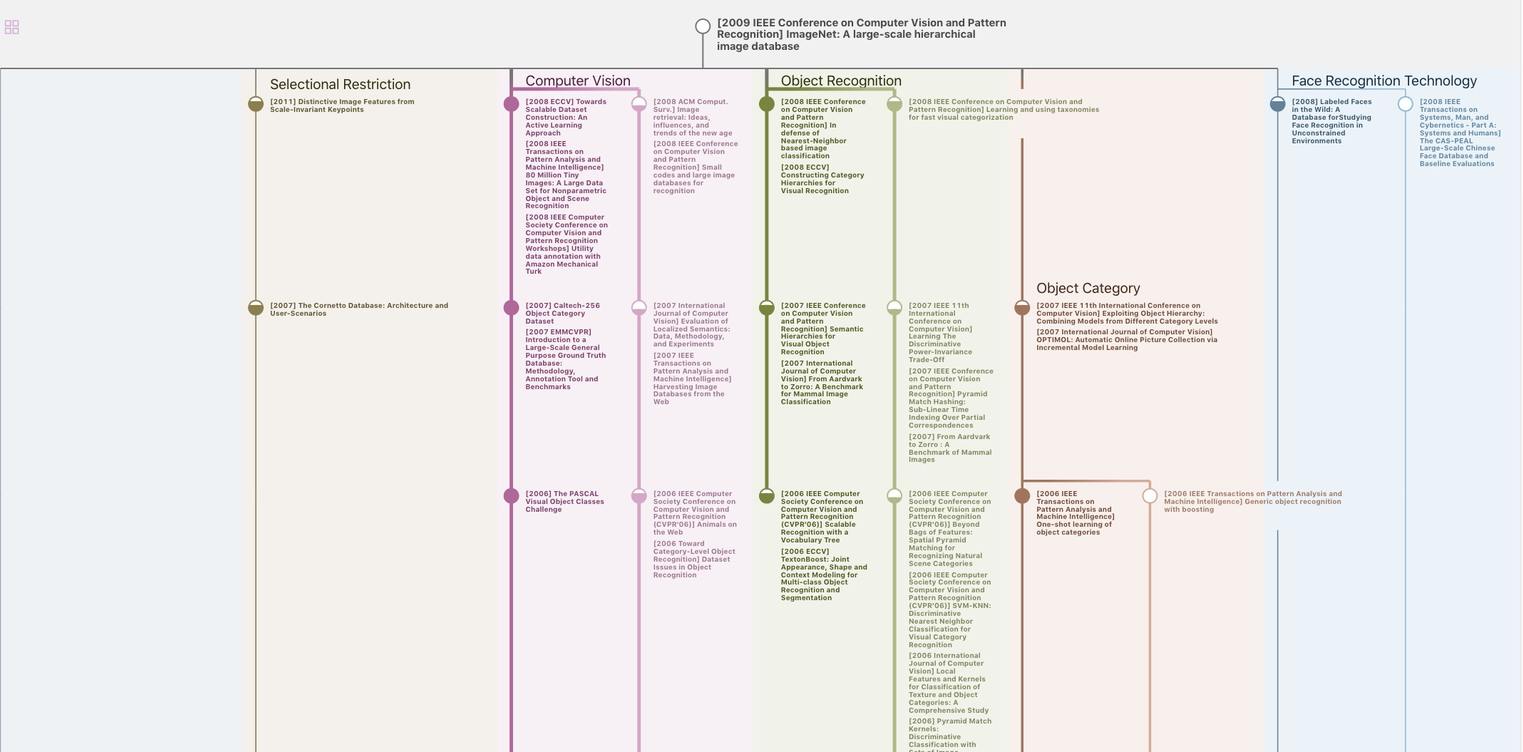
生成溯源树,研究论文发展脉络
Chat Paper
正在生成论文摘要