Improving Multi-Label Classification for Learning Objects Categorization by Taking into Consideration Usage Information.
JOURNAL OF UNIVERSAL COMPUTER SCIENCE(2019)
摘要
Learning objects are digital resources that can be deployed by means of a web system for supporting teaching. A key advantage is reuse, and this is possible thanks to learning objects repositories that allow learning object search, management and categorization. In this work, we propose a novel approach towards automatically learning object categorization taking into consideration learning object usage information. We use a multi-label learning approach since each learning object might be associated with multiple categories. We have developed a methodology with three main stages allowing us to firstly select the most suitable set of text features from learning objects metadata, secondly selecting how much historical learning object usage information can enhance classification performance, and finally selecting the best multilabel classification algorithms with our data. We have carried out an experimental work using 519 learning objects gathered from the AGORA repository for 8 years. We have compared 13 multi-label classification algorithms over 16 evaluation measures. The results obtained show that usage information about the learning object can improve the classification.
更多查看译文
关键词
multi-label classification,feature selection,usage information,learning object categorization
AI 理解论文
溯源树
样例
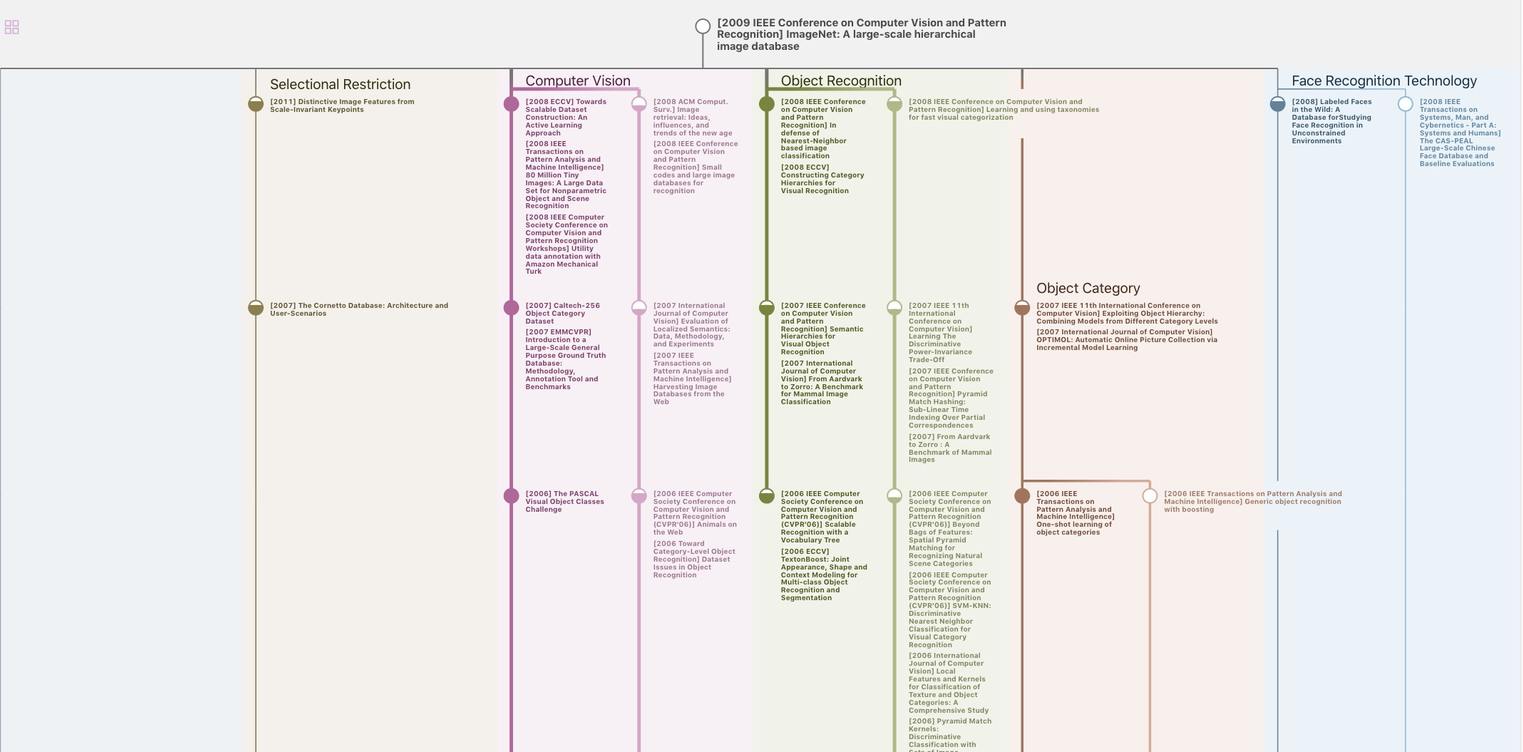
生成溯源树,研究论文发展脉络
Chat Paper
正在生成论文摘要