Multivariate Extremes Over A Random Number Of Observations
SCANDINAVIAN JOURNAL OF STATISTICS(2021)
摘要
The classical multivariate extreme-value theory concerns the modeling of extremes in a multivariate random sample, suggesting the use of max-stable distributions. In this work, the classical theory is extended to the case where aggregated data, such as maxima of a random number of observations, are considered. We derive a limit theorem concerning the attractors for the distributions of the aggregated data, which boil down to a new family of max-stable distributions. We also connect the extremal dependence structure of classical max-stable distributions and that of our new family of max-stable distributions. Using an inversion method, we derive a semiparametric composite-estimator for the extremal dependence of the unobservable data, starting from a preliminary estimator of the extremal dependence of the aggregated data. Furthermore, we develop the large-sample theory of the composite-estimator and illustrate its finite-sample performance via a simulation study.
更多查看译文
关键词
extremal dependence, extreme-value copula, inverse problem, multivariate max-stable distribution, nonparametric estimation, Pickands dependence function
AI 理解论文
溯源树
样例
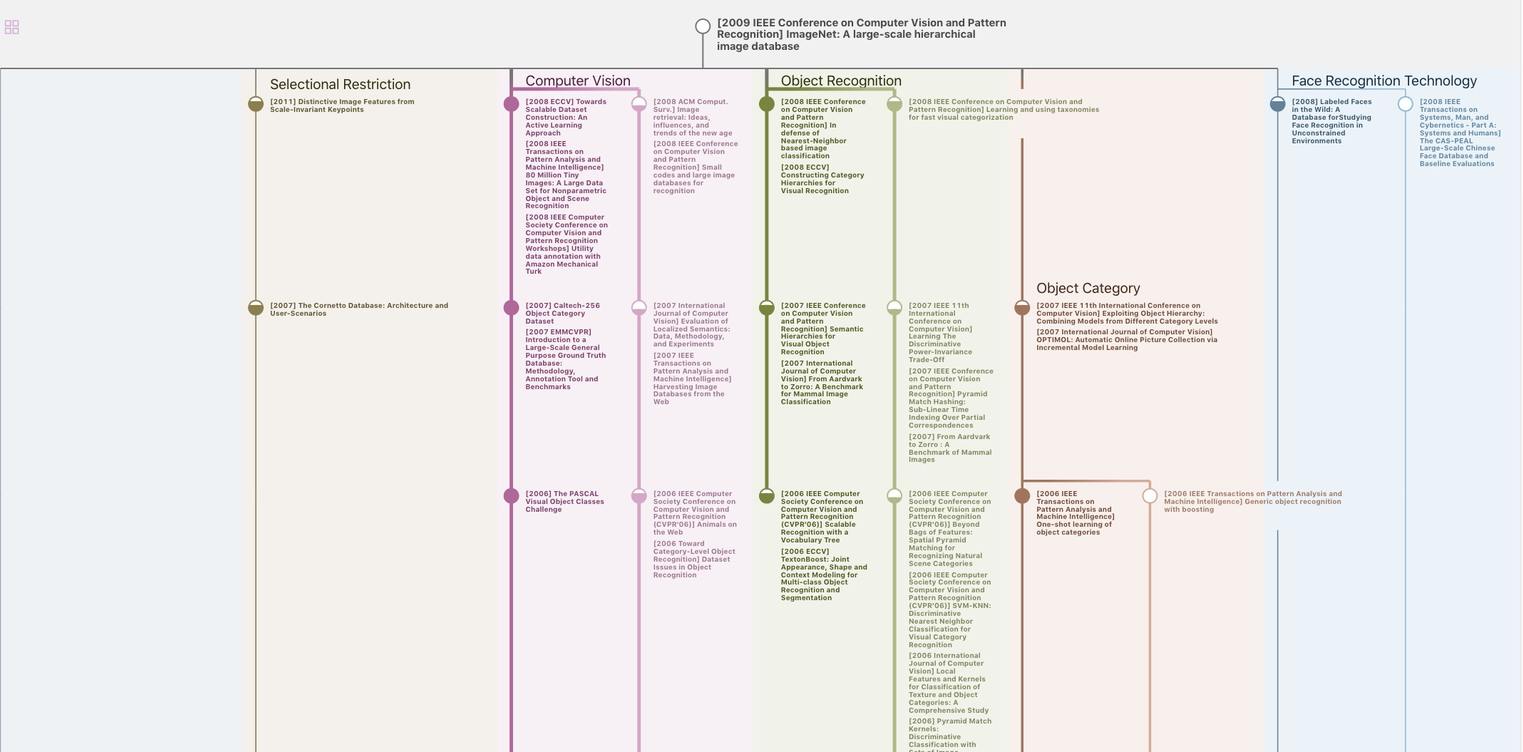
生成溯源树,研究论文发展脉络
Chat Paper
正在生成论文摘要