Efficient Intent Detection with Dual Sentence Encoders
NLP FOR CONVERSATIONAL AI(2020)
摘要
Building conversational systems in new domains and with added functionality requires resource-efficient models that work under low-data regimes (i.e., in few-shot setups). Motivated by these requirements, we introduce intent detection methods backed by pretrained dual sentence encoders such as USE and ConveRT. We demonstrate the usefulness and wide applicability of the proposed intent detectors, showing that: 1) they outperform intent detectors based on fine-tuning the full BERT-Large model or using BERT as a fixed black-box encoder on three diverse intent detection data sets; 2) the gains are especially pronounced in few-shot setups (i.e., with only 10 or 30 annotated examples per intent); 3) our intent detectors can be trained in a matter of minutes on a single CPU; and 4) they are stable across different hyperparameter settings. In hope of facilitating and democratizing research focused on intention detection, we release our code, as well as a new challenging single-domain intent detection dataset comprising 13,083 annotated examples over 77 intents.
更多查看译文
关键词
efficient intent detection,dual
AI 理解论文
溯源树
样例
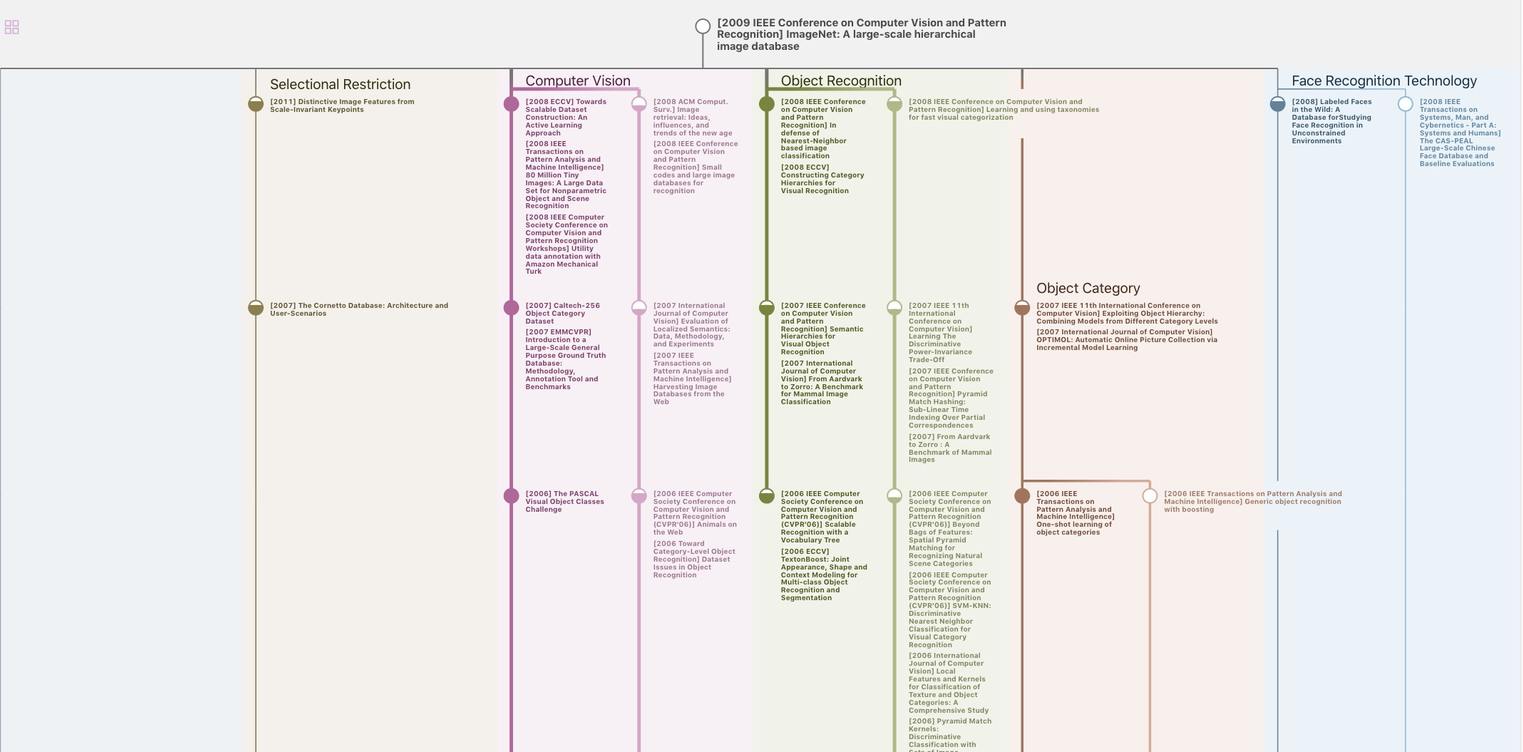
生成溯源树,研究论文发展脉络
Chat Paper
正在生成论文摘要