Classification of different vehicles in traffic using RGB and Depth images: A Fast RCNN Approach
2019 IEEE International Conference on Imaging Systems and Techniques (IST)(2019)
摘要
The Fast RCNN framework utilizes the region proposals generated from the RGB images in general for object classification and detection. This paper describes about the vehicle classification employing the Fast RCNN framework and utilizing the information provided from the combination of depth images and RGB images in the form of region proposals for object detection and classification. We use this underlying system architecture to perform evaluation on the Indian and Thailand vehicle traffic datasets. Overall, we achieve a mAP of 72.91% using RGB region proposals, and mAP of 73.77% using RGB combined with depth proposals, for the Indian dataset; and mAP of 80.61% on RGB region proposals, and mAP of 81.25% on RGB combined with depth region proposals, for the Thailand dataset. Our results show that RGB combined with depth region proposals mAP performance is slightly better than the region proposals generated using RGB images only. Furthermore, we provide insights on the performance of AP(Average Precision) for each vehicle on Thailand dataset and how effective region proposals generation is crucial for object detection using the FastRCNN framework.
更多查看译文
关键词
FastRCNN,RGB region proposals,depth region proposals,vehicle classification,mAP(Mean Average Precision)
AI 理解论文
溯源树
样例
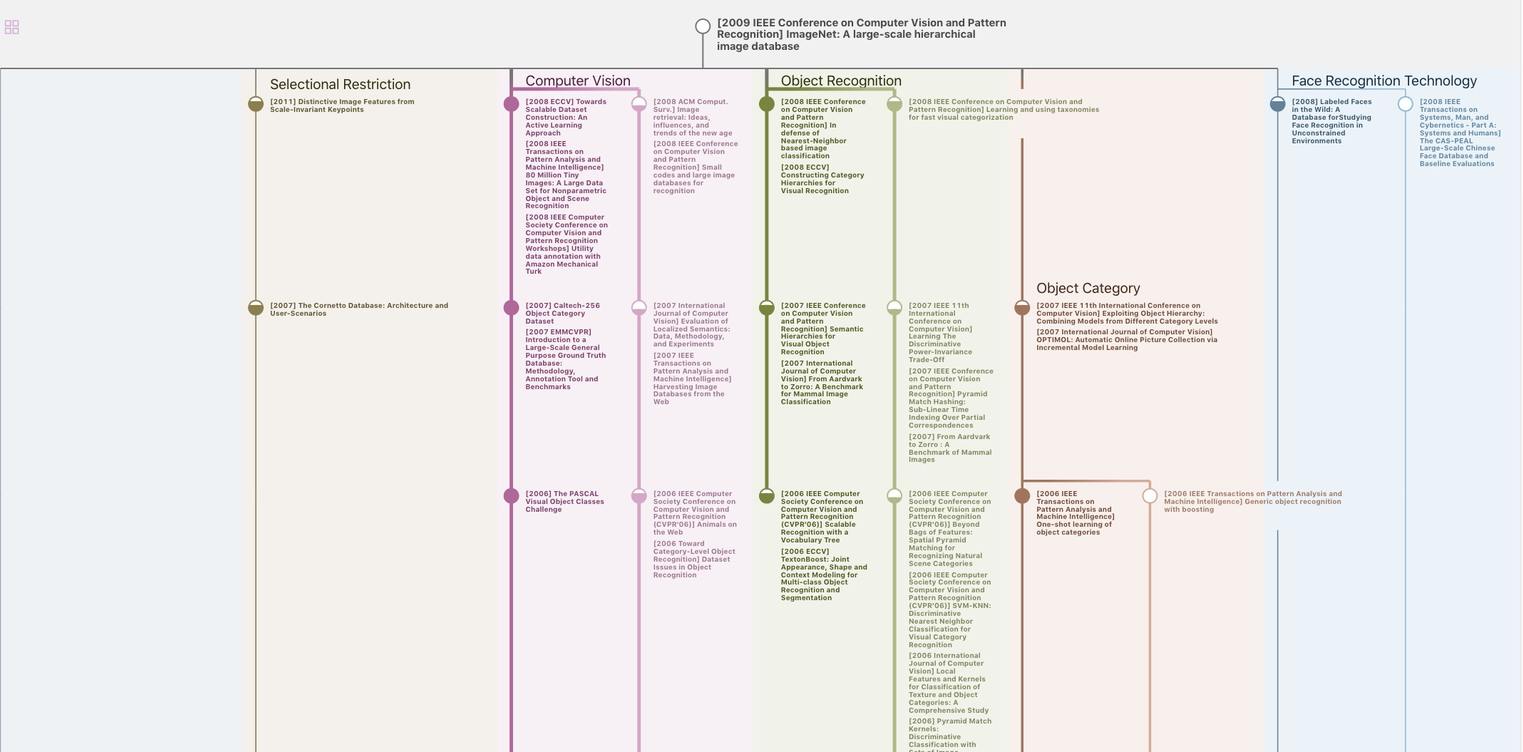
生成溯源树,研究论文发展脉络
Chat Paper
正在生成论文摘要