Interactive Correction of Mislabeled Training Data
2019 IEEE Conference on Visual Analytics Science and Technology (VAST)(2019)
摘要
In this paper, we develop a visual analysis method for interactively improving the quality of labeled data, which is essential to the success of supervised and semi-supervised learning. The quality improvement is achieved through the use of user-selected trusted items. We employ a bi-level optimization model to accurately match the labels of the trusted items and to minimize the training loss. Based on this model, a scalable data correction algorithm is developed to handle tens of thousands of labeled data efficiently. The selection of the trusted items is facilitated by an incremental tSNE with improved computational efficiency and layout stability to ensure a smooth transition between different levels. We evaluated our method on real-world datasets through quantitative evaluation and case studies, and the results were generally favorable.
更多查看译文
关键词
Labeled data debugging,trusted item,tSNE
AI 理解论文
溯源树
样例
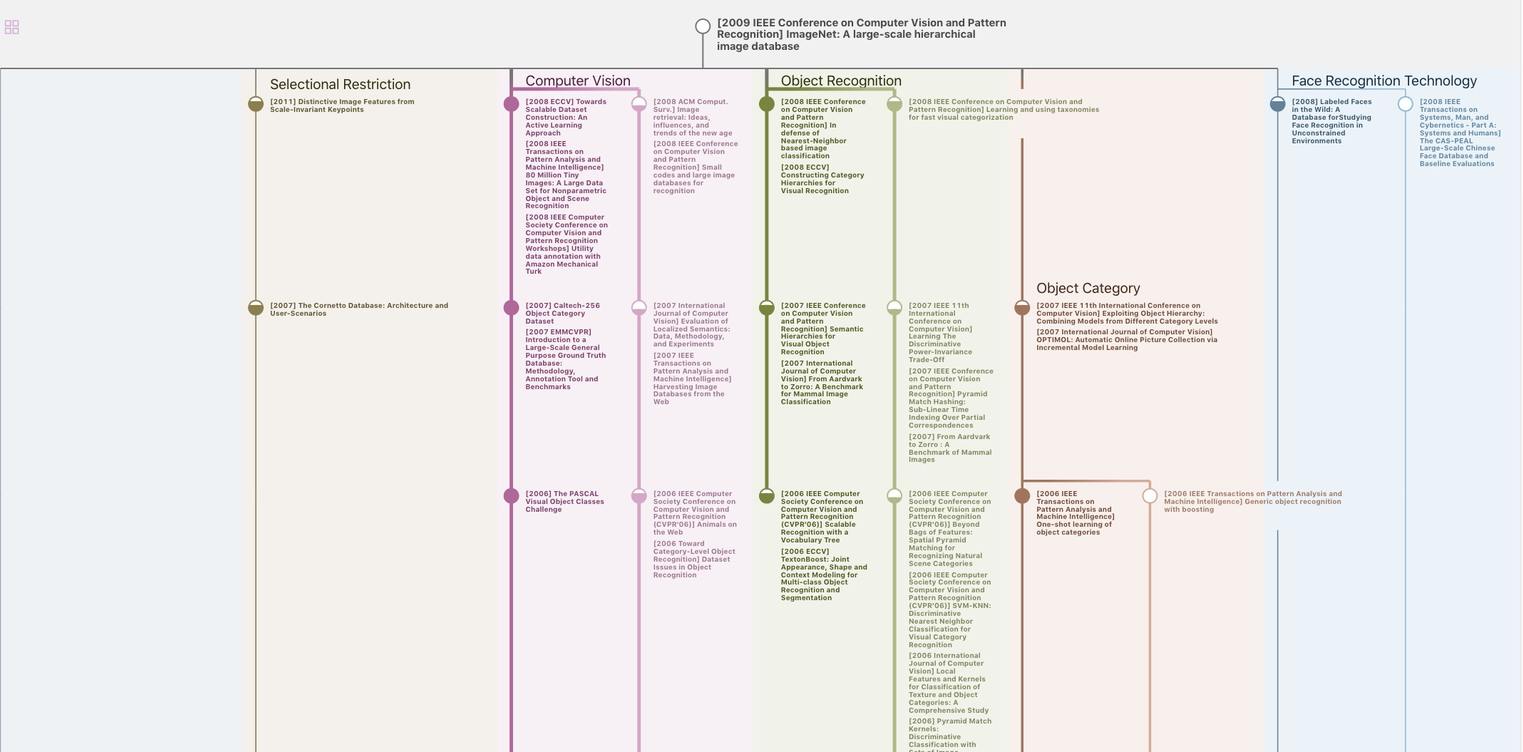
生成溯源树,研究论文发展脉络
Chat Paper
正在生成论文摘要