Fully Convolutional Geometric Features
2019 IEEE/CVF INTERNATIONAL CONFERENCE ON COMPUTER VISION (ICCV 2019)(2019)
摘要
Extracting geometric features from 3D scans or point clouds is the first step in applications such as registration, reconstruction, and tracking. State-of-the-art methods require computing low-level features as input or extracting patch-based features with limited receptive field. In this work, we present fully-convolutional geometric features, computed in a single pass by a 3D fully-convolutional network. We also present new metric learning losses that dramatically improve performance. Fully-convolutional geometric features are compact, capture broad spatial context, and scale to large scenes. We experimentally validate our approach on both indoor and outdoor datasets. Fully-convolutional geometric features achieve state-of-the-art accuracy without requiring prepossessing, are compact (32 dimensions), and are 290 times faster than the most accurate prior method.
更多查看译文
关键词
low-level features,fully-convolutional geometric features,3D fully-convolutional network,patch-based features,point clouds,metric learning losses,spatial context
AI 理解论文
溯源树
样例
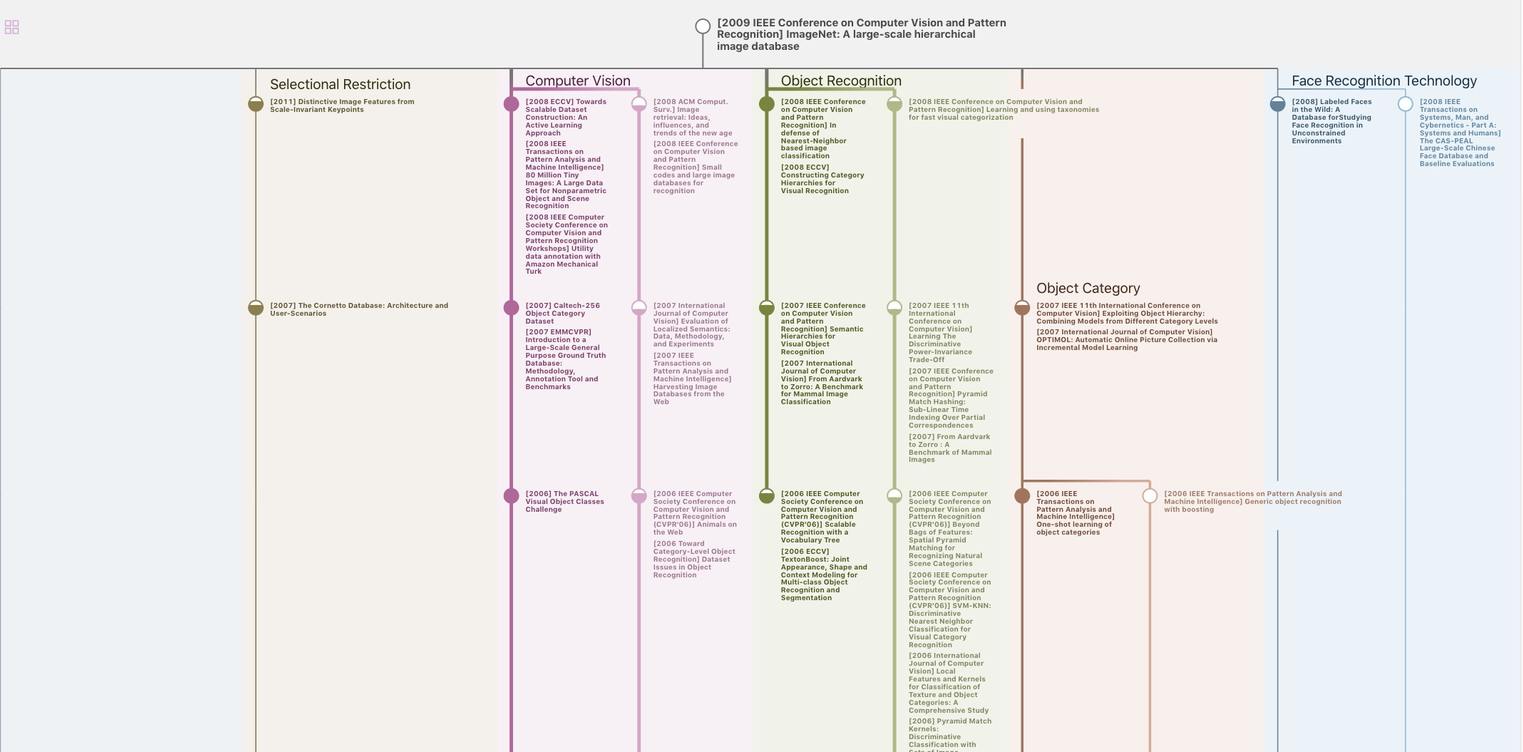
生成溯源树,研究论文发展脉络
Chat Paper
正在生成论文摘要