Gaussian Process Reinforcement Learning for Fast Opportunistic Spectrum Access
2019 IEEE GLOBAL COMMUNICATIONS CONFERENCE (GLOBECOM)(2020)
摘要
Opportunistic spectrum access (OSA) is envisioned to support the spectrum demand of future-generation wireless networks. In practice, primary channels are usually correlated and network dynamics is unknown alpha-priori. This entails a great challenge on sensing policy design, and conventional model-based methods are generally inapplicable. In this paper, we propose a novel Gaussian process reinforcement learning (GPRL) based model-free solution to enable the fast sensing policy optimization in OSA. In essence, Gaussian process is embedded in RL framework as a Q-function approximator to efficiently utilize the past learning experience. A novel kernel function is first tailor designed to measure spectrum data correlation. Then a covariance-based exploration strategy is developed to strike a better trade-off between the exploration and exploitation in RL. Our simulation results show that the proposed GPRL can obtain a near-optimal policy with significantly reduced learning period compared with deep reinforcement learning.
更多查看译文
关键词
Sensors, Correlation, Kernel, Gaussian processes, Learning (artificial intelligence), Radio frequency, Training, Opportunistic spectrum access, sensing policy, Gaussian process reinforcement learning (GPRL), machine learning
AI 理解论文
溯源树
样例
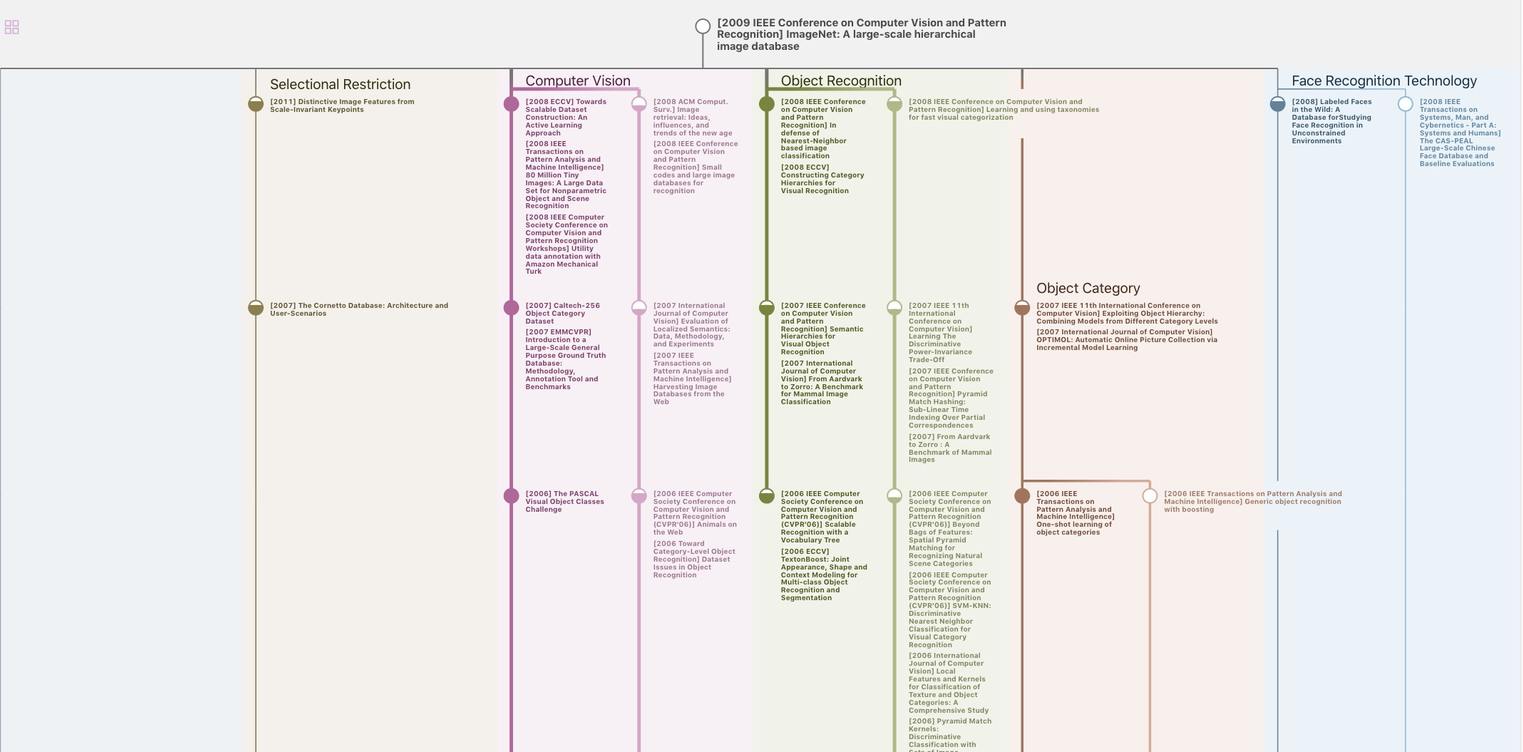
生成溯源树,研究论文发展脉络
Chat Paper
正在生成论文摘要