Joint Power Control and User Association Strategy in Green HetNets Using Deep Q-Network with LSTM
IEEE Global Communications Conference(2019)
Abstract
With the proliferation of users in wireless communication networks and the increase in traffic, heterogeneous networks have emerged. Minimizing the total energy consumption of base stations is an important issue for environmental reasons. Therefore, the concept of a green heterogeneous network was proposed. However, the deployment of a large number of small base stations also poses problems for user management. In order to better improve the decision-making and self-organizing ability of the network, the paper also proposes an user energy consumption prediction model based on Recurrent Neural Network. After that, the article also proposes a power control scheme through a Deep Reinforcement Learning algorithm with Long Short-Term Memory to improve the quality of user service. Finally, a joint optimization scheme of network power control and user management is realized. The experimental results show that the joint optimization effect using this method can converge to better solution and reduce energy consumption compared with traditional deep reinforcement learning.
MoreTranslated text
Key words
network power control,user management,joint optimization effect,traditional deep reinforcement learning,green HetNets,Deep q-network,wireless communication networks,heterogeneous networks,total energy consumption,base stations,environmental reasons,green heterogeneous network,decision-making,self-organizing ability,user energy consumption prediction model,Recurrent Neural Network,power control scheme,Deep Reinforcement Learning algorithm,Long Short-Term Memory,quality of user service,joint optimization scheme
AI Read Science
Must-Reading Tree
Example
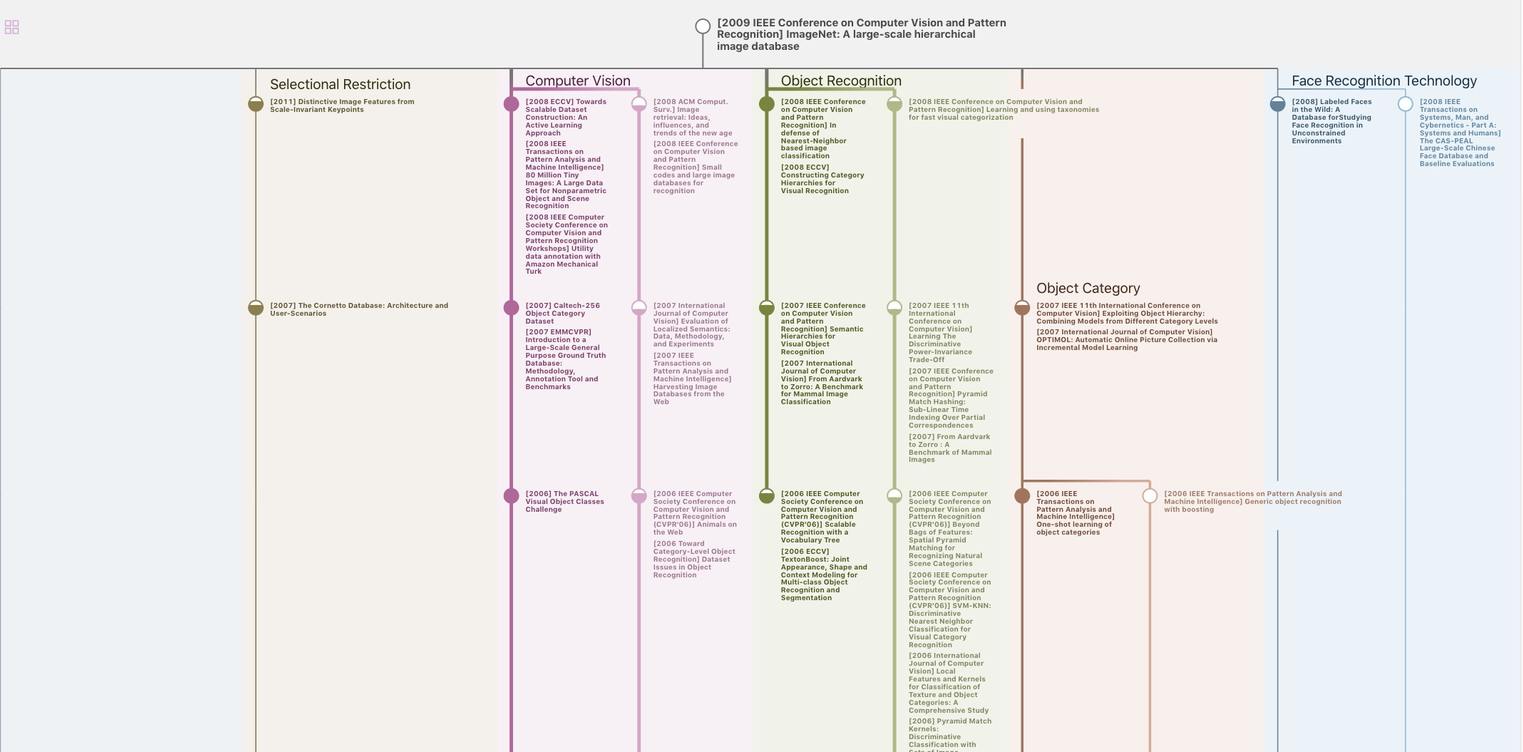
Generate MRT to find the research sequence of this paper
Chat Paper
Summary is being generated by the instructions you defined