Touch the Wind: Simultaneous Airflow, Drag and Interaction Sensing on a Multirotor
2020 IEEE/RSJ International Conference on Intelligent Robots and Systems (IROS)(2020)
摘要
Disturbance estimation for Micro Aerial Vehicles (MAVs) is crucial for robustness and safety. In this paper, we use novel, bio-inspired airflow sensors to measure the airflow acting on a MAV, and we fuse this information in an Unscented Kalman filter (UKF) to simultaneously estimate the three-dimensional wind vector, the drag force, and other interaction forces (e.g. due to collisions, interaction with a human) acting on the robot. To this end, we present and compare a fully model-based and a deep learning-based strategy. The model-based approach considers the MAV and airflow sensor dynamics and its interaction with the wind, while the deep learning-based strategy uses a Long Short-Term Memory (LSTM) to obtain an estimate of the relative airflow, which is then fused in the proposed filter. We validate our methods in hardware experiments, showing that we can accurately estimate relative airflow of up to 4 m/s, and we can differentiate drag and interaction force.
更多查看译文
关键词
airflow sensor dynamics,deep learning-based strategy,long short-term memory,interaction force,simultaneous airflow,interaction sensing,multirotor,disturbance estimation,microaerial vehicles,MAV,robustness,bio-inspired airflow sensors,unscented Kalman filter,three-dimensional wind vector,drag force,LSTM
AI 理解论文
溯源树
样例
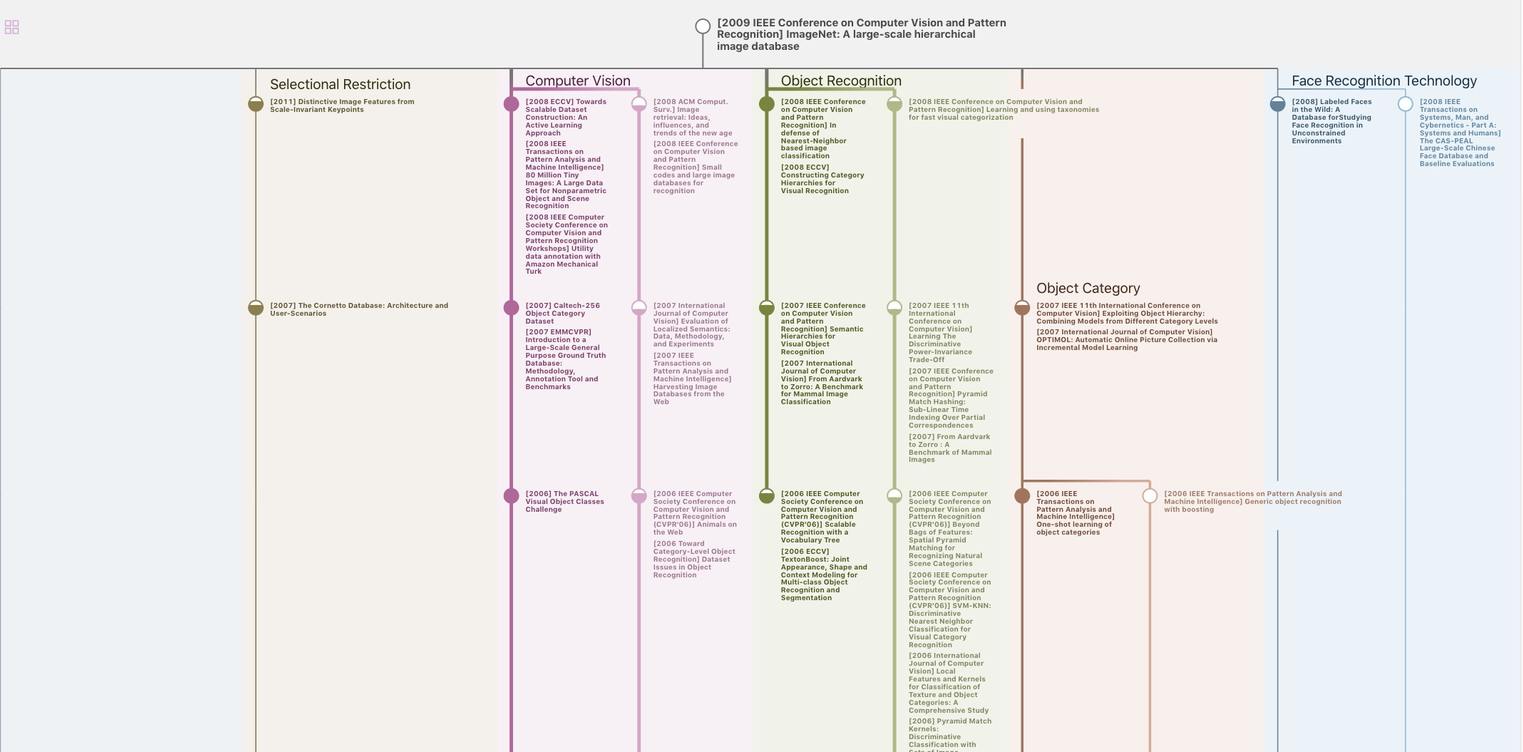
生成溯源树,研究论文发展脉络
Chat Paper
正在生成论文摘要