Fast High-Order Sparse Subspace Clustering With Cumulative MRF for Hyperspectral Images
IEEE Geoscience and Remote Sensing Letters(2021)
摘要
Sparse subspace clustering (SSC), as a powerful tool in hyperspectral image (HSI) segmentation, has caused widespread attention recently. However, the existing methods are generally affected by the limitations of region consistency and time complexity. To address these issues, we propose a fast high-order SSC (FHoSSC) with the cumulative Markov random field (MRF) algorithm in this letter. By capturing high-order information, we explore the pixel-level contextual restraints within the same high-order data to preserve consistency. Also, a new regularization term is introduced by the spatial constraints among adjacent high-order data for improving segmentation accuracy. Finally, cumulative MRF, as a variant of MRF, is used to further refine the segmentation result through combining original HSI information. Experiments on real data sets demonstrate that the proposed method not only outperforms the state-of-the-art methods in segmentation accuracy but also reduces the time complexity significantly.
更多查看译文
关键词
Cumulative Markov random field (MRF),high-order information,hyperspectral image (HSI) segmentation,sparse subspace clustering (SSC)
AI 理解论文
溯源树
样例
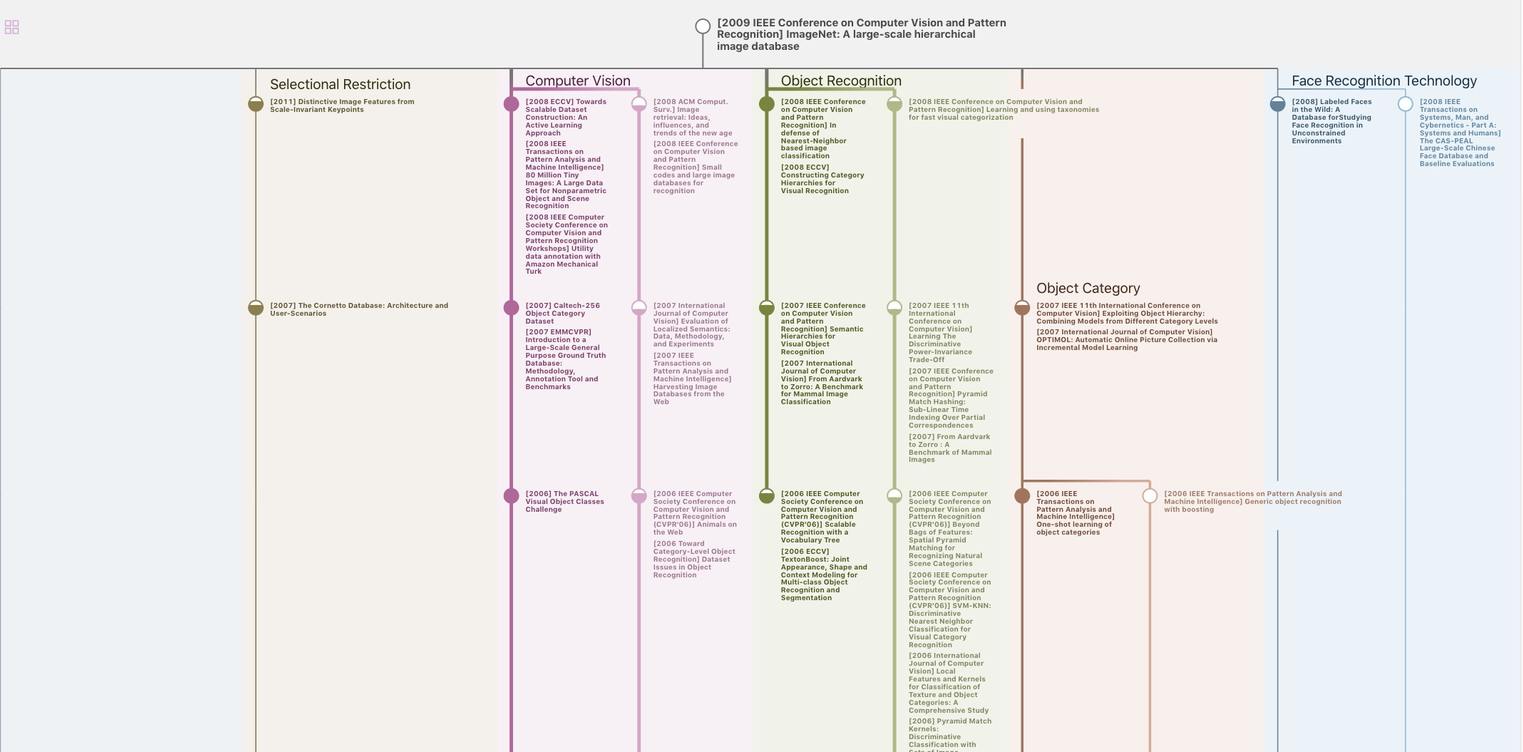
生成溯源树,研究论文发展脉络
Chat Paper
正在生成论文摘要